注意力机制的图卷积网络与时序卷积网络如何进行组合?请给出相应代码并注释
时间: 2024-05-01 07:21:50 浏览: 10
注意力机制的图卷积网络与时序卷积网络可以通过将它们的输出连接起来来进行组合。具体来说,我们可以将图卷积网络和时序卷积网络的输出在最后一个维度上进行拼接,然后再通过一些全连接层进行融合和预测。
以下是一个可能的代码实现及注释:
```python
import torch.nn as nn
import torch.nn.functional as F
class GraphConvNet(nn.Module):
def __init__(self, in_dim, hidden_dim, out_dim):
super(GraphConvNet, self).__init__()
self.conv1 = nn.Conv2d(in_dim, hidden_dim, kernel_size=3, padding=1)
self.conv2 = nn.Conv2d(hidden_dim, out_dim, kernel_size=3, padding=1)
def forward(self, x, adj):
# x: input feature matrix, shape=(batch_size, in_dim, num_nodes, num_timesteps)
# adj: adjacency matrix, shape=(batch_size, num_nodes, num_nodes)
x = F.relu(self.conv1(x))
x = F.relu(self.conv2(x))
# calculate attention weights using adjacency matrix
attn = F.softmax(adj, dim=-1)
# apply attention weights to the output of the graph convolutional layers
x = torch.matmul(attn, x)
return x
class TimeConvNet(nn.Module):
def __init__(self, in_dim, hidden_dim, out_dim):
super(TimeConvNet, self).__init__()
self.conv1 = nn.Conv2d(in_dim, hidden_dim, kernel_size=(3, 1), padding=(1, 0))
self.conv2 = nn.Conv2d(hidden_dim, out_dim, kernel_size=(3, 1), padding=(1, 0))
def forward(self, x):
# x: input feature matrix, shape=(batch_size, in_dim, num_nodes, num_timesteps)
x = F.relu(self.conv1(x))
x = F.relu(self.conv2(x))
# apply max-pooling operation along the temporal dimension
x = F.max_pool2d(x, kernel_size=(1, x.size(-1)))
return x
class GraphTimeNet(nn.Module):
def __init__(self, graph_in_dim, graph_hidden_dim, graph_out_dim,
time_in_dim, time_hidden_dim, time_out_dim, fc_dim, num_classes):
super(GraphTimeNet, self).__init__()
self.graph_conv = GraphConvNet(graph_in_dim, graph_hidden_dim, graph_out_dim)
self.time_conv = TimeConvNet(time_in_dim, time_hidden_dim, time_out_dim)
self.fc1 = nn.Linear(graph_out_dim + time_out_dim, fc_dim)
self.fc2 = nn.Linear(fc_dim, num_classes)
def forward(self, x, adj):
# x: input feature matrix, shape=(batch_size, in_dim, num_nodes, num_timesteps)
# adj: adjacency matrix, shape=(batch_size, num_nodes, num_nodes)
graph_x = self.graph_conv(x, adj)
time_x = self.time_conv(x)
# concatenate the output of the graph and time convolutions
x = torch.cat([graph_x, time_x], dim=1)
x = F.relu(self.fc1(x))
x = self.fc2(x)
return x
```
在这个例子中,我们首先定义了一个图卷积网络和一个时序卷积网络作为子模块。然后,我们定义了一个包含这两个子模块的主模块。主模块的 `forward` 方法首先对输入数据分别调用子模块的 `forward` 方法,然后将两个输出在最后一个维度上进行拼接。最后,我们通过一些全连接层对拼接后的输出进行融合和预测。注意,在这个例子中,我们使用了一个简单的全连接层来完成融合和预测,实际上可以根据具体任务需要使用不同的结构。
相关推荐
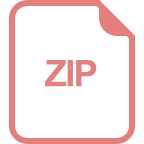
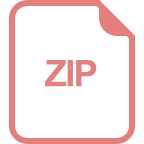














