train, val, test, s = (data.get(x) for x in ('train', 'val', 'test', 'download')) if val: val = [Path(x).resolve() for x in (val if isinstance(val, list) else [val])] # val path if not all(x.exists() for x in val): LOGGER.info('\nDataset not found ⚠️, missing paths %s' % [str(x) for x in val if not x.exists()])
时间: 2023-06-27 07:03:06 浏览: 91
这段代码是一个数据集加载的代码。首先,它从`data`字典中获取`train`、`val`、`test`和`download`四个键对应的值。如果`val`不为`None`,则将其转化成路径对象,并检查路径是否存在。如果有任何一个路径不存在,则会在日志中输出警告信息。这段代码的目的是确保数据集的文件路径都是存在的,以便后续能够正确地读取数据。
相关问题
def get_CIFAR10_data(num_training=500, num_validation=50, num_test=50): """ Load the CIFAR-10 dataset from disk and perform preprocessing to prepare it for classifiers. These are the same steps as we used for the SVM, but condensed to a single function. """ # Load the raw CIFAR-10 data cifar10_dir = 'C:/download/cifar-10-python/cifar-10-batches-py/data_batch_1' X_train, y_train, X_test, y_test = load_CIFAR10(cifar10_dir) print (X_train.shape) # Subsample the data mask = range(num_training, num_training + num_validation) X_val = X_train[mask] y_val = y_train[mask] mask = range(num_training) X_train = X_train[mask] y_train = y_train[mask] mask = range(num_test) X_test = X_test[mask] y_test = y_test[mask] # Normalize the data: subtract the mean image mean_image = np.mean(X_train, axis=0) X_train -= mean_image X_val -= mean_image X_test -= mean_image # Transpose so that channels come first X_train = X_train.transpose(0, 3, 1, 2).copy() X_val = X_val.transpose(0, 3, 1, 2).copy() X_test = X_test.transpose(0, 3, 1, 2).copy() # Package data into a dictionary return { 'X_train': X_train, 'y_train': y_train, 'X_val': X_val, 'y_val': y_val, 'X_test': X_test, 'y_test': y_test, }
这段代码定义了一个函数get_CIFAR10_data,用于加载和预处理CIFAR-10数据集,并返回一个包含训练集、验证集和测试集的字典。
具体来说,这个函数完成了以下几个步骤:
1. 调用load_CIFAR10函数加载CIFAR-10数据集,得到原始的训练集和测试集数据(X_train, y_train, X_test, y_test)。
2. 对原始数据进行子采样,得到num_training个样本作为训练集,num_validation个样本作为验证集,num_test个样本作为测试集。
3. 对训练集、验证集和测试集进行均值归一化,即将每个像素减去训练集的均值像素值。这个操作可以提高模型的训练效果和泛化能力。
4. 对训练集、验证集和测试集进行通道转置,即将原来的(样本数, 高, 宽, 通道数)的四维数组转换为(样本数, 通道数, 高, 宽)的四维数组。这个操作是因为在深度学习中,通常把通道数放在第二个维度上,方便卷积计算。
5. 将处理后的训练集、验证集和测试集打包成一个字典,返回给调用者。
需要注意的是,这个函数中使用了load_CIFAR10函数,也就是另一个函数,用于从文件中加载CIFAR-10数据集。另外,这里的cifar10_dir变量指定了CIFAR-10数据集的路径,需要根据实际情况进行修改。
Task: - Download the UCM dataset from the official source or any reputable repository. - Preprocess the dataset by splitting it into training, validation, and test sets. Please use an 80-10-10 split, respectively. - Choose a suitable deep-learning algorithm for scene classification. You can use popular classifiers such as Convolutional Neural Networks (CNNs). - Implement the chosen algorithm in Python, using appropriate libraries. - Train the classifier using the training set and tune the hyperparameters using the validation set. - Evaluate the trained classifier on the test set using the following metrics: Accuracy: The overall classification accuracy. Precision: The ability to correctly classify positive samples. Recall: The ability to correctly identify positive samples. F1-score: The harmonic mean of precision and recall. Confusion Matrix: A table showing the number of correct and incorrect predictions for each class. Report: Prepare a one-page report summarizing the results of your scene classification experiment and the architecture of your network. Submit your Python code along with the one-page report summarizing the results. Make sure to organize your code and report in a clear and readable manner.
Sure, here's an outline of the steps you'll need to take to complete this task:
1. Download the UCM dataset from the official source or any reputable repository.
2. Preprocess the dataset by splitting it into training, validation, and test sets. Use an 80-10-10 split, respectively. You can use a library like scikit-learn to do this.
3. Choose a suitable deep-learning algorithm for scene classification. Convolutional Neural Networks (CNNs) are a popular choice for image classification tasks like this.
4. Implement the chosen algorithm in Python, using appropriate libraries such as TensorFlow or PyTorch.
5. Train the classifier using the training set and tune the hyperparameters using the validation set.
6. Evaluate the trained classifier on the test set using the following metrics: accuracy, precision, recall, F1-score, and confusion matrix. You can use libraries like scikit-learn to compute these metrics.
7. Prepare a one-page report summarizing the results of your scene classification experiment and the architecture of your network. Include any relevant information such as which hyperparameters you tuned and which ones you used for the final model.
Here's some sample code to get you started:
```
# Step 1: Download UCM dataset
# TODO: Download dataset and extract files
# Step 2: Preprocess dataset
from sklearn.model_selection import train_test_split
# TODO: Load dataset into memory
X_train_val, X_test, y_train_val, y_test = train_test_split(X, y, test_size=0.1, random_state=42)
X_train, X_val, y_train, y_val = train_test_split(X_train_val, y_train_val, test_size=0.1, random_state=42)
# Step 3: Choose deep-learning algorithm
import tensorflow as tf
from tensorflow.keras.layers import Conv2D, MaxPooling2D, Flatten, Dense
model = tf.keras.Sequential([
Conv2D(32, (3, 3), activation='relu', input_shape=(256, 256, 3)),
MaxPooling2D((2, 2)),
Conv2D(64, (3, 3), activation='relu'),
MaxPooling2D((2, 2)),
Conv2D(128, (3, 3), activation='relu'),
MaxPooling2D((2, 2)),
Conv2D(256, (3, 3), activation='relu'),
MaxPooling2D((2, 2)),
Flatten(),
Dense(256, activation='relu'),
Dense(21, activation='softmax')
])
# Step 4: Implement algorithm in Python
model.compile(optimizer='adam',
loss='categorical_crossentropy',
metrics=['accuracy'])
# Step 5: Train classifier
history = model.fit(X_train, y_train, epochs=10, validation_data=(X_val, y_val))
# Step 6: Evaluate trained classifier
from sklearn.metrics import accuracy_score, precision_score, recall_score, f1_score, confusion_matrix
y_pred = model.predict(X_test)
y_pred_classes = np.argmax(y_pred, axis=1)
y_test_classes = np.argmax(y_test, axis=1)
accuracy = accuracy_score(y_test_classes, y_pred_classes)
precision = precision_score(y_test_classes, y_pred_classes, average='macro')
recall = recall_score(y_test_classes, y_pred_classes, average='macro')
f1 = f1_score(y_test_classes, y_pred_classes, average='macro')
confusion_mat = confusion_matrix(y_test_classes, y_pred_classes)
print("Accuracy:", accuracy)
print("Precision:", precision)
print("Recall:", recall)
print("F1-score:", f1)
print("Confusion matrix:\n", confusion_mat)
# Step 7: Prepare report
# TODO: Write report summarizing results and network architecture
```
阅读全文
相关推荐
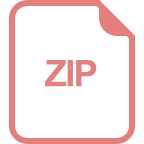
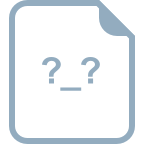
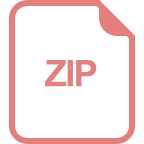
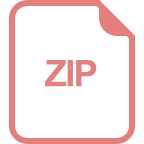
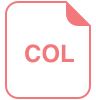
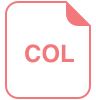
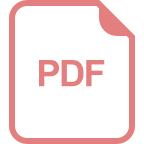
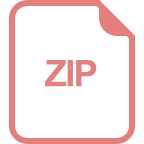
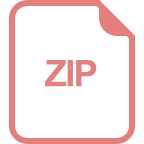
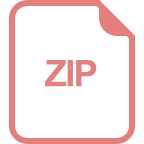
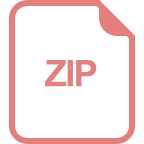
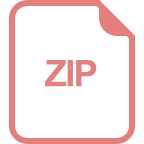
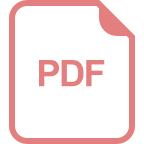
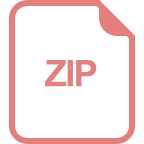
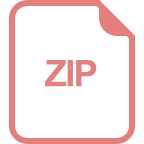
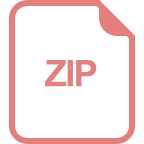