function [weight,bias] = SGD(hidenActiFcn,outputActiFcn,weight,bias,nabla_weight,nabla_bias,nlayer,mini_batch_size,eta,a,z,y,lambda,n) %SGD stochastic gradient descent delta = (a{nlayer}-y).*outputActiFcn(z{nlayer}); nabla_bias{end} = mean(delta,2); nabla_weight{end} = (delta*a{end-1}')/mini_batch_size; for in = nlayer-1:-1:2 delta = weight{in+1}'*delta.*hidenActiFcn(z{in}); nabla_bias{in} = mean(delta,2); nabla_weight{in} = (delta*a{in-1}')/mini_batch_size; end for in = 2:nlayer weight{in} = (1-eta*lambda/n)*weight{in} - eta*nabla_weight{in}; bias{in} = bias{in} - eta*nabla_bias{in}; end end
时间: 2024-02-18 12:06:16 浏览: 21
这段代码是实现了一个基于随机梯度下降(Stochastic Gradient Descent, SGD)算法的神经网络训练函数。该函数接收的输入参数包括:
- `hidenActiFcn`,隐藏层的激活函数类型;
- `outputActiFcn`,输出层的激活函数类型;
- `weight`,网络的权重参数;
- `bias`,网络的偏置参数;
- `nabla_weight`,当前mini-batch的权重梯度;
- `nabla_bias`,当前mini-batch的偏置梯度;
- `nlayer`,神经网络的层数;
- `mini_batch_size`,mini-batch的大小;
- `eta`,学习率;
- `a`,每层的激活值;
- `z`,每层的加权输入值;
- `y`,期望输出值;
- `lambda`,L2正则化系数;
- `n`,训练集大小。
具体来说,该函数实现了以下步骤:
1. 计算输出层的偏导数 `delta = (a{nlayer}-y).*outputActiFcn(z{nlayer})`,并计算输出层的权重和偏置的梯度 `nabla_bias{end} = mean(delta,2); nabla_weight{end} = (delta*a{end-1}')/mini_batch_size;`。
2. 从倒数第二层开始,计算每一层的偏导数和梯度,并将其存储在 `delta`、`nabla_bias` 和 `nabla_weight` 中。
3. 根据梯度下降算法更新每层的权重和偏置参数。
4. 返回更新后的权重和偏置参数。
其中,步骤3中的权重和偏置参数的更新公式为:
```matlab
weight{in} = (1-eta*lambda/n)*weight{in} - eta*nabla_weight{in};
bias{in} = bias{in} - eta*nabla_bias{in};
```
其中,`in` 表示当前层的下标。这里使用了L2正则化来防止过拟合。
相关推荐
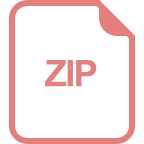















