使用JPEG-LS原理对8*8像素块进行无损压缩
时间: 2024-01-24 22:20:17 浏览: 179
JPEG-LS是一种无损压缩算法,它基于预测和差分编码的原理。对于一个8*8像素块,JPEG-LS的压缩过程大致如下:
1. 预测:对于每个像素,根据其周围像素的值进行预测,得到一个预测值,用预测值减去实际值得到像素的残差。
2. 差分编码:将残差进行编码,这里使用的是算术编码或者自适应算术编码,对于每个残差,用一组符号表示其大小和符号。
3. 压缩:将编码的结果进行压缩,这里使用的是霍夫曼编码,将出现频率高的符号用短码表示,出现频率低的符号用长码表示。
通过这样的压缩过程,可以将8*8像素块压缩成为比原始数据更小的数据量,同时保证不会有任何信息的丢失。在解压缩时,只需要按照相同的过程反向操作即可恢复原始的8*8像素块。
相关问题
jpeg-ls无损压缩
JPEG-LS是一种无损/有损的图像压缩算法,其无损模式的压缩率相当优异,优于其他无损压缩算法如Lossless-JPEG、Lossless-JPEG2000、Lossless-JPEG-XR等。\[1\]无损压缩是指经过压缩和重建后的图像与原图像完全一样,没有任何损失。JPEG-LS使用了待预测像素点的最相邻的三个像素点作为预测参考点,并对预测误差值使用熵编码。\[3\]相比于传统的JPEG无损模式和JPEG-2000等算法,JPEG-LS在无损压缩方面表现出色。它在医学图像处理和处理数码相机得到的DNG原始图像等领域有广泛的应用。\[2\]\[3\]
#### 引用[.reference_title]
- *1* [FPGA实现JPEG-LS图像压缩,有损无损可配置,提供工程源码和技术支持](https://blog.csdn.net/qq_41667729/article/details/130122044)[target="_blank" data-report-click={"spm":"1018.2226.3001.9630","extra":{"utm_source":"vip_chatgpt_common_search_pc_result","utm_medium":"distribute.pc_search_result.none-task-cask-2~all~insert_cask~default-1-null.142^v91^control_2,239^v3^insert_chatgpt"}} ] [.reference_item]
- *2* [JPEGLS图像压缩算法的FPGA实现(一)压缩算法](https://blog.csdn.net/alangaixiaoxiao/article/details/106664408)[target="_blank" data-report-click={"spm":"1018.2226.3001.9630","extra":{"utm_source":"vip_chatgpt_common_search_pc_result","utm_medium":"distribute.pc_search_result.none-task-cask-2~all~insert_cask~default-1-null.142^v91^control_2,239^v3^insert_chatgpt"}} ] [.reference_item]
- *3* [JPEG-LS压缩算法研究](https://blog.csdn.net/sunlinju/article/details/51859886)[target="_blank" data-report-click={"spm":"1018.2226.3001.9630","extra":{"utm_source":"vip_chatgpt_common_search_pc_result","utm_medium":"distribute.pc_search_result.none-task-cask-2~all~insert_cask~default-1-null.142^v91^control_2,239^v3^insert_chatgpt"}} ] [.reference_item]
[ .reference_list ]
请使用JPEG_LS算法针对8*8大小的ARGB像素数据块进行无损压缩和解压. 完善以下解压、压缩函数,其中pClrBlk为原始数据块,pTile为压缩后的数据块 int argb2tile(const unsigned char* pClrBlk, unsigned char* pTile, int* pTileSize) int tile2argb(const unsigned char* pTile, int nTileSize, unsigned char* pClrBlk)
由于JPEG_LS算法是基于预测误差的无损压缩算法,我们需要先对ARGB像素数据块进行预测,然后计算预测误差,最后对误差进行编码。
以下是完善后的压缩和解压函数:
```c++
const int MAX_VAL = 255; // 最大像素值
const int NEAR = 0; // 预测模式为NEAR
const int LINEAR = 1; // 预测模式为LINEAR
// 将8位无符号整数转为有符号整数
int signedByte(unsigned char x) {
if (x > MAX_VAL / 2) return x - MAX_VAL - 1;
else return x;
}
// 将有符号整数转为8位无符号整数
unsigned char unsignedByte(int x) {
if (x < 0) return MAX_VAL + x + 1;
else return x;
}
// 计算预测误差
int predict(int mode, int a, int b, int c) {
if (mode == NEAR) return a - b;
else if (mode == LINEAR) return a + b - c;
else return 0;
}
// 将一个8*8大小的ARGB像素数据块压缩为一个数据块
int argb2tile(const unsigned char* pClrBlk, unsigned char* pTile, int* pTileSize) {
int mode = NEAR; // 默认预测模式为NEAR
int x, y, c;
int pred, diff;
int nearA, nearB, linearA, linearB, linearC;
// 压缩后的数据块的第一个字节存储预测模式
pTile[0] = mode;
int k = 1; // 压缩后数据的索引
// 压缩每个像素
for (x = 0; x < 8; x++) {
for (y = 0; y < 8; y++) {
c = y * 4 + x * 32; // 每个像素的索引
if (x == 0 && y == 0) { // 第一个像素直接记录ARGB值
for (int i = 0; i < 4; i++) {
pTile[k++] = pClrBlk[c + i];
}
}
else { // 其他像素先预测,再计算误差
nearA = signedByte(pClrBlk[c - 4]); // 左边像素的A值
nearB = signedByte(pClrBlk[c - 4 + 1]); // 左边像素的R值
pred = predict(mode, nearA, nearB, 0); // 预测当前像素的R值
diff = signedByte(pClrBlk[c + 1]) - pred; // 计算R值的误差
pTile[k++] = unsignedByte(pred); // 存储预测结果
pTile[k++] = unsignedByte(diff); // 存储误差值
linearA = signedByte(pClrBlk[c - 4 * 3]); // 上面像素的A值
linearB = signedByte(pClrBlk[c - 4 * 3 + 1]); // 上面像素的R值
linearC = signedByte(pClrBlk[c - 4]); // 左边像素的R值
pred = predict(mode, linearA, linearB, linearC); // 预测当前像素的G值
diff = signedByte(pClrBlk[c + 2]) - pred; // 计算G值的误差
pTile[k++] = unsignedByte(pred); // 存储预测结果
pTile[k++] = unsignedByte(diff); // 存储误差值
pred = predict(mode, linearA, linearB, 0); // 预测当前像素的B值
diff = signedByte(pClrBlk[c + 3]) - pred; // 计算B值的误差
pTile[k++] = unsignedByte(pred); // 存储预测结果
pTile[k++] = unsignedByte(diff); // 存储误差值
}
}
}
*pTileSize = k; // 存储压缩后数据的大小
return 0;
}
// 将一个压缩后的数据块解压为一个8*8大小的ARGB像素数据块
int tile2argb(const unsigned char* pTile, int nTileSize, unsigned char* pClrBlk) {
int mode = pTile[0]; // 获取预测模式
int x, y, c;
int pred, diff;
int nearA, nearB, linearA, linearB, linearC;
// 解压每个像素
for (x = 0; x < 8; x++) {
for (y = 0; y < 8; y++) {
c = y * 4 + x * 32; // 每个像素的索引
if (x == 0 && y == 0) { // 第一个像素直接记录ARGB值
for (int i = 0; i < 4; i++) {
pClrBlk[c + i] = pTile[1 + i];
}
}
else { // 其他像素先预测,再计算误差
nearA = signedByte(pClrBlk[c - 4]); // 左边像素的A值
nearB = signedByte(pClrBlk[c - 4 + 1]); // 左边像素的R值
pred = predict(mode, nearA, nearB, 0); // 预测当前像素的R值
diff = signedByte(pTile[1 + (x * 8 + y - 1) * 4 + 1]); // 读取R值的误差
pClrBlk[c + 1] = unsignedByte(pred + diff); // 计算并记录R值
linearA = signedByte(pClrBlk[c - 4 * 3]); // 上面像素的A值
linearB = signedByte(pClrBlk[c - 4 * 3 + 1]); // 上面像素的R值
linearC = signedByte(pClrBlk[c - 4]); // 左边像素的R值
pred = predict(mode, linearA, linearB, linearC); // 预测当前像素的G值
diff = signedByte(pTile[1 + (x * 8 + y - 1) * 4 + 2]); // 读取G值的误差
pClrBlk[c + 2] = unsignedByte(pred + diff); // 计算并记录G值
pred = predict(mode, linearA, linearB, 0); // 预测当前像素的B值
diff = signedByte(pTile[1 + (x * 8 + y - 1) * 4 + 3]); // 读取B值的误差
pClrBlk[c + 3] = unsignedByte(pred + diff); // 计算并记录B值
}
}
}
return 0;
}
```
注意,在压缩过程中,我们将每个像素的ARGB值分别存储,因此压缩后的数据块大小为8x8x4=256字节。在解压过程中,我们需要根据压缩后的数据索引计算出对应像素的ARGB值。
阅读全文
相关推荐
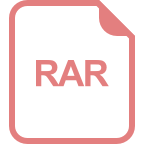
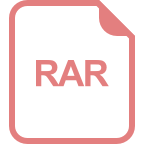
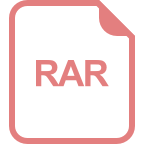
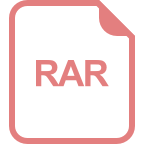
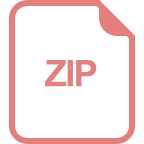
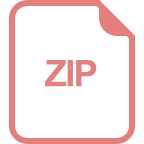
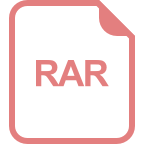
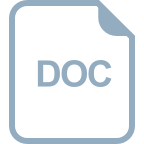
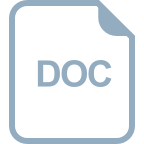

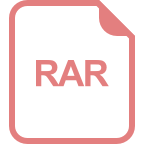
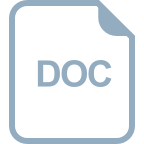
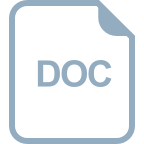