多时间尺度优化调度matlab代码
时间: 2023-05-11 10:01:04 浏览: 162
多时间尺度优化调度Matlab代码是一个能够在不同时间尺度下对系统进行优化调度的工具。该代码通过分析系统中存在的多个时间尺度,如小时、天、月、年等,针对不同时间尺度下的数据进行分析,最终得出系统的最优解。在使用该代码时,需要在Matlab中进行相关参数的设置,包括系统的初始状态、时间尺度、目标函数等。
多时间尺度优化调度Matlab代码的优点在于对系统进行了全面的考虑,充分利用不同时间尺度下的数据,为系统管理和控制提供了更加准确的数据支持。同时,该代码具有较高的灵活性和适应性,可以根据具体情况进行调整,满足不同系统的管理需求。
使用多时间尺度优化调度Matlab代码时,需要注意相关参数的设置和数据的预处理。同时,为了保证计算结果的准确性,需要对计算过程进行密切的监控和跟踪,及时对计算结果进行验证和修正。
总之,多时间尺度优化调度Matlab代码是一个具备较高实用性的系统管理和控制工具,能够为不同系统提供全面的优化调度方案。
相关问题
多时间尺度优化调度 matlab
在MATLAB中进行多时间尺度的优化调度是通过结合不同时间尺度的优化方法和调度算法来实现的。
首先,在不同时间尺度上进行优化调度,可以考虑使用分时段优化方法。即将整个调度过程分为几个时间段,每个时间段内采用不同的优化方法进行调度。例如,可以根据不同时间段的负载情况、能源价格等因素来确定最优调度策略,从而实现能源的高效利用和成本的最小化。
其次,为了实现多时间尺度的优化调度,可以采用多层次的调度算法。比如,可以将整个系统分为高层调度和低层调度两个层次。在高层调度中,可以根据整个系统的运行状态和需求进行长期调度规划,例如确定最佳的运行策略、设备配置等;而在低层调度中,则可以根据短期变化的信息,如负荷变化、能源价格波动等,采用更细粒度的调度算法进行实时调度控制,以优化能源的分配和利用。
此外,MATLAB还提供了多时间尺度优化调度的工具和函数,如优化工具箱中的线性规划、整数规划、非线性规划等函数可以用于建立多时间尺度的优化模型;Simulink和Stateflow等工具可以用于建立多层次的调度算法和进行系统仿真。
综上所述,MATLAB可以通过结合不同时间尺度的优化方法和调度算法,以及利用相关工具和函数,实现多时间尺度的优化调度。通过合理的调度策略和算法,可以提高能源的利用效率、降低成本,并实现系统的可持续发展。
多目标鲸鱼算法建筑综合能源优化调度matlab代码
以下是一个简单的多目标鲸鱼算法建筑综合能源优化调度的Matlab代码示例:
```matlab
% 建筑能源模型参数
Ts = 24; % 仿真时间步长
Pelec = zeros(Ts,1); % 电力负荷
Pheat = zeros(Ts,1); % 供热负荷
Pcool = zeros(Ts,1); % 供冷负荷
COPheat = 3.5; % 供热系统热效率
COPcool = 4.0; % 供冷系统冷效率
Eelec = 0.1; % 电价
Eheat = 0.2; % 供热价格
Ecool = 0.15; % 供冷价格
% 鲸鱼算法参数
MaxIt = 100; % 最大迭代次数
nPop = 50; % 种群数量
nVar = 3; % 优化变量数量
VarSize = [1 nVar]; % 优化变量维度
VarMin = [0 0 0]; % 优化变量最小值
VarMax = [1 1 1]; % 优化变量最大值
% 初始化种群
empty_individual.Position = [];
empty_individual.Cost = [];
pop = repmat(empty_individual, nPop, 1);
for i = 1:nPop
pop(i).Position = unifrnd(VarMin, VarMax, VarSize);
pop(i).Cost = CostFunction(pop(i).Position, Ts, Pelec, Pheat, Pcool, COPheat, COPcool, Eelec, Eheat, Ecool);
end
% 鲸鱼算法主循环
for it = 1:MaxIt
% 计算适应度值
Costs = [pop.Cost];
WorstCost = max(Costs);
BestCost = min(Costs);
AvgCost = mean(Costs);
% 计算适应度值的标准差
StdCost = std(Costs);
% 计算单个鲸鱼的迁徙距离
WhaleMoves = zeros(nPop, nVar);
for i = 1:nPop
WhaleMoves(i,:) = LevyFlight(std(VarMax-VarMin), nVar);
end
% 进行鲸鱼迁徙
for i = 1:nPop
if rand() < 0.5 % 群体迁徙
% 随机选择另一个鲸鱼
j = randi([1 nPop], 1);
% 计算当前鲸鱼与另一个鲸鱼的距离
Xdiff = abs(pop(i).Position - pop(j).Position);
% 计算另一个鲸鱼的迁徙距离
D = rand()*WhaleMoves(i,:).*Xdiff;
% 计算新的位置
NewPosition = pop(i).Position + randn(VarSize).*D;
else % 个体迁徙
% 计算个体迁徙距离
D = WhaleMoves(i,:);
% 计算新的位置
NewPosition = pop(i).Position + randn(VarSize).*D;
end
% 确保新位置在边界内
NewPosition = max(NewPosition, VarMin);
NewPosition = min(NewPosition, VarMax);
% 计算新位置的适应度值
NewCost = CostFunction(NewPosition, Ts, Pelec, Pheat, Pcool, COPheat, COPcool, Eelec, Eheat, Ecool);
% 更新鲸鱼位置和适应度值
if NewCost < pop(i).Cost
pop(i).Position = NewPosition;
pop(i).Cost = NewCost;
end
end
end
% 适应度函数
function J = CostFunction(x, Ts, Pelec, Pheat, Pcool, COPheat, COPcool, Eelec, Eheat, Ecool)
% 计算建筑能源模型的目标函数
ElecConsump = sum(Pelec.*x(:,1));
HeatConsump = sum(Pheat.*x(:,2))/COPheat;
CoolConsump = sum(Pcool.*x(:,3))*COPcool;
J(1) = ElecConsump + HeatConsump + CoolConsump;
J(2) = ElecConsump*Eelec + HeatConsump*Eheat + CoolConsump*Ecool;
end
% Levy飞行函数
function s = LevyFlight(sigma, n)
beta = 1.5;
% 计算步长
s = (randn(1,n).*sigma)./abs(randn(1,n)).^(1/beta);
end
```
以上代码仅为示例,具体实现需要根据实际需求进行调整。在实际应用中,还需要进一步优化参数设置、适应度函数等方面,以获得更好的优化效果。
相关推荐
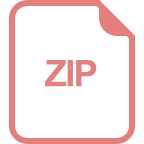
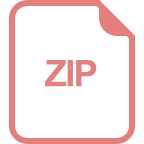












