自适应差分进化算法matlab
时间: 2023-06-24 08:02:52 浏览: 49
自适应差分进化算法(Adaptive Differential Evolution, ADE)是一种优化算法,可以用于解决复杂的非线性优化问题。它是差分进化算法(Differential Evolution, DE)的一种改进,通过自适应地调整算法的控制参数来提高算法的性能。
以下是使用MATLAB实现ADE算法的基本步骤:
1. 初始化种群:选择适当的初始种群大小,并使用随机数生成器生成初始种群中每个个体的随机初始解。
2. 确定适应度函数:根据问题的特征选择适当的适应度函数。对于最小化问题,适应度函数越小越好。
3. 设定算法参数:包括交叉率、变异率、种群大小等。
4. 开始迭代:对于每一代,对种群中每个个体进行以下步骤:
a. 选择父代:从种群中随机选择3个个体作为父代。
b. 变异:根据变异率,对父代进行变异生成一组新的解。
c. 交叉:根据交叉率,对新解和原始解进行交叉生成一个后代。
d. 评估适应度:计算后代的适应度值。
e. 更新种群:根据选择策略,选择后代或原始解中适应度值更好的一个作为下一代种群中的个体。
5. 判断终止条件:如果达到了预设的迭代次数或满足预设的停止准则,则终止迭代。
6. 输出结果:输出最优解及其对应的适应度值。
参考代码实现:
```matlab
function [bestsol, bestval, history] = ade(fhd, dim, bounds, maxfunevals, options)
% fhd: function handle to the objective function
% dim: number of decision variables
% bounds: [lower bound; upper bound]
% maxfunevals: maximum number of function evaluations
% options: algorithmic options
% set algorithmic options and parameters
popsize = options.PopulationSize;
F = options.F;
CR = options.CR;
strategy = options.Strategy;
% initialize population and memory
pop = repmat(bounds(1,:), popsize, 1) + repmat((bounds(2,:) - bounds(1,:)), popsize, 1) .* rand(popsize, dim);
memory.pop = pop;
memory.f = feval(fhd, pop');
% set history
history = zeros(maxfunevals, 1);
funevals = popsize;
% main loop
while funevals < maxfunevals
% generate trial vectors
switch strategy
case 1 % DE/rand/1
idx = randperm(popsize, 3);
v = pop(idx(1),:) + F * (pop(idx(2),:) - pop(idx(3),:));
case 2 % DE/current-to-best/1
[~, bestidx] = min(memory.f);
idx = randperm(popsize, 2);
v = pop(bestidx,:) + F * (pop(idx(1),:) - pop(idx(2),:));
otherwise
error('Unknown DE strategy');
end
% clip trial vectors to bounds
v = max(min(v, bounds(2,:)), bounds(1,:));
% crossover
cridx = rand(popsize, dim) < CR;
u = pop;
u(cridx) = v(cridx);
% evaluate new population
f = feval(fhd, u');
funevals = funevals + popsize;
% update population and memory
switch strategy
case 1 % DE/rand/1
betteridx = f < memory.f;
case 2 % DE/current-to-best/1
betteridx = f < memory.f | (f == memory.f & rand(1,popsize) < 0.5);
otherwise
error('Unknown DE strategy');
end
memory.pop(betteridx,:) = u(betteridx,:);
memory.f(betteridx) = f(betteridx);
pop(betteridx,:) = u(betteridx,:);
% update history
history(funevals-popsize+1:funevals) = min(memory.f);
end
% output best solution and its value
[bestval, bestidx] = min(memory.f);
bestsol = memory.pop(bestidx,:);
end
```
这里提供了两种策略:DE/rand/1和DE/current-to-best/1,分别对应了上述步骤4中的 a~e。其中,DE/current-to-best/1 在选择向量时使用了当前种群中最优个体的信息,通常比 DE/rand/1 更有效。在实际应用中,可以根据问题的特点选择适当的策略。
使用该函数时,需要传入目标函数的函数句柄、决策变量个数、决策变量的上下界、最大函数评价次数和算法选项等参数。返回最优解及其对应的函数值,以及算法的收敛曲线。
相关推荐
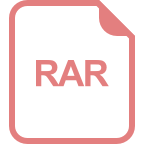
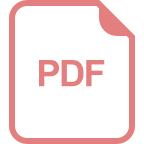
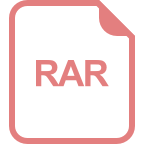








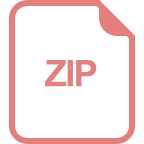
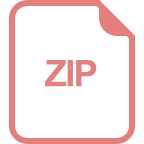
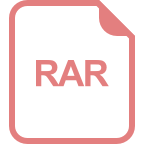