rf2=AdaBoostClassifier(base_estimator = DecisionTreeClassifier( max_depth = 1,class_weight='balanced'), n_estimators=200, learning_rate=, random_state=1)各参数什么意思
时间: 2024-02-14 08:25:21 浏览: 42
这段代码是在使用scikit-learn库中的`AdaBoostClassifier`类来创建一个AdaBoost分类器的实例,并设置了一些参数。
具体解释如下:
- `base_estimator = DecisionTreeClassifier(max_depth=1, class_weight='balanced')`: `base_estimator`是AdaBoost算法所使用的基础分类器,默认为决策树(`DecisionTreeClassifier`)。在这里,使用决策树作为基础分类器,并设置最大深度为1,即单层决策树。`class_weight='balanced'`表示使用类别平衡权重,以处理不平衡数据集。
- `n_estimators=200`: `n_estimators`指定了AdaBoost算法中基础分类器的数量,也就是要构建的决策树的数量。在这里,设置为200个决策树。
- `learning_rate`: `learning_rate`是AdaBoost算法中的学习率,用于控制每个基础分类器的权重。学习率越小,每个基础分类器的权重越小。在这段代码中,学习率未给出具体的值,你需要为其赋予一个合适的值。
- `random_state=1`: `random_state`是用于控制随机性的参数。设置一个固定的随机种子可以使得结果可重复。
通过这段代码,你可以创建一个AdaBoost分类器,并根据给定的参数进行配置。你可以根据具体需求调整参数的值。
相关问题
# Create AdaBoost classifier with decision tree as base estimator adaboost_clf = AdaBoostClassifier(base_estimator=DecisionTreeClassifier(), n_estimators=100, learning_rate=0.1, algorithm='SAMME.R')
这是使用scikit-learn库中的AdaBoost分类器构建模型的示例代码。其中,base_estimator参数用于指定基础分类器,默认为决策树分类器。n_estimators参数表示AdaBoost算法中使用的基础分类器数量,learning_rate参数表示每个分类器的权重缩减率,algorithm参数指定了AdaBoost算法使用的多分类策略。通过这个步骤,可以创建一个AdaBoost分类器,并用它来训练和预测数据。
是否运算的出来AdaBoostClassifier(base_estimator=GradientBoostingClassifier() n_estimators=50, learning_rate=0.1, random_state=42)
在 scikit-learn 中,AdaBoostClassifier 的 `base_estimator` 参数需要传入一个可调用的分类器对象,而 GradientBoostingClassifier 返回的是一个训练好的分类器对象,因此不能直接将 GradientBoostingClassifier 对象作为 `base_estimator` 参数传入 AdaBoostClassifier 中。
如果希望使用 GradientBoostingClassifier 进行 Boosting,可以使用 GradientBoostingClassifier 的 `n_estimators` 参数和 `learning_rate` 参数来控制训练的轮数和学习率,从而实现类似 AdaBoostClassifier 的效果。例如:
```python
gb = GradientBoostingClassifier(n_estimators=50, learning_rate=0.1, random_state=42)
ab = AdaBoostClassifier(base_estimator=gb, n_estimators=50, learning_rate=0.1, random_state=42)
```
这里先使用 GradientBoostingClassifier 训练一个基分类器,然后将其作为参数传入 AdaBoostClassifier 中,从而实现使用 GradientBoostingClassifier 进行 Boosting 的效果。
相关推荐
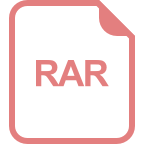













