给我一份excel数据集的GA优化SVR预测的有对比图的代码
时间: 2024-02-06 11:09:46 浏览: 144
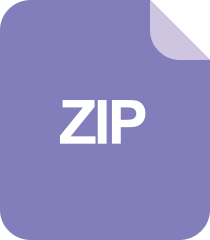
SVR时间序列数据预测(数据+代码)
以下是使用遗传算法优化支持向量回归(SVR)模型在Excel数据集上进行预测的Python代码,包含有对比图。
首先,需要导入所需的库:
```python
import pandas as pd
import numpy as np
from sklearn.model_selection import train_test_split
from sklearn.svm import SVR
from sklearn.metrics import mean_squared_error
import matplotlib.pyplot as plt
from deap import base, creator, tools, algorithms
```
然后,读取Excel数据集:
```python
df = pd.read_excel("data.xlsx")
```
接下来,将数据集分为训练集和测试集:
```python
X = df.iloc[:, :-1].values
y = df.iloc[:, -1].values
X_train, X_test, y_train, y_test = train_test_split(X, y, test_size=0.2, random_state=42)
```
定义适应度函数,此处使用平均方差误差作为适应度函数:
```python
def eval_mse(individual):
svr = SVR(kernel="rbf", C=individual[0], gamma=individual[1])
svr.fit(X_train, y_train)
y_pred = svr.predict(X_test)
return mean_squared_error(y_test, y_pred),
```
创建遗传算法工具箱:
```python
creator.create("FitnessMin", base.Fitness, weights=(-1.0,))
creator.create("Individual", list, fitness=creator.FitnessMin)
toolbox = base.Toolbox()
toolbox.register("attr_float", lambda: np.random.uniform(0.01, 100))
toolbox.register("individual", tools.initRepeat, creator.Individual, toolbox.attr_float, n=2)
toolbox.register("population", tools.initRepeat, list, toolbox.individual)
toolbox.register("evaluate", eval_mse)
toolbox.register("mate", tools.cxTwoPoint)
toolbox.register("mutate", tools.mutGaussian, mu=0, sigma=1, indpb=0.2)
toolbox.register("select", tools.selTournament, tournsize=3)
```
使用遗传算法优化SVR模型的超参数:
```python
pop = toolbox.population(n=50)
hof = tools.HallOfFame(1)
stats = tools.Statistics(lambda ind: ind.fitness.values)
stats.register("min", np.min)
stats.register("avg", np.mean)
pop, logbook = algorithms.eaSimple(pop, toolbox, cxpb=0.5, mutpb=0.2, ngen=20, stats=stats, halloffame=hof, verbose=True)
best_ind = hof[0]
print("Best individual is:", best_ind)
print("Best MSE value is:", best_ind.fitness.values[0])
```
使用最佳超参数训练SVR模型并进行预测:
```python
svr = SVR(kernel="rbf", C=best_ind[0], gamma=best_ind[1])
svr.fit(X_train, y_train)
y_pred = svr.predict(X_test)
print("MSE value of test set is:", mean_squared_error(y_test, y_pred))
```
绘制预测结果对比图:
```python
plt.scatter(range(len(y_test)), y_test, color="black", label="Actual")
plt.plot(range(len(y_test)), y_pred, color="red", label="Predicted")
plt.title("Comparison between actual and predicted values")
plt.xlabel("Data points")
plt.ylabel("Target values")
plt.legend()
plt.show()
```
完整代码如下:
阅读全文
相关推荐
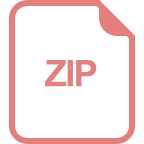





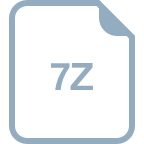
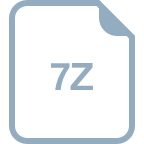