编写卷积神经网络ResNet34的模型代码
时间: 2023-10-23 19:10:59 浏览: 286
好的,以下是基于 PyTorch 的 ResNet34 的模型代码:
```python
import torch.nn as nn
import torch.nn.functional as F
class ResidualBlock(nn.Module):
def __init__(self, in_channels, out_channels, stride=1, downsample=None):
super(ResidualBlock, self).__init__()
self.conv1 = nn.Conv2d(in_channels, out_channels, kernel_size=3, stride=stride, padding=1, bias=False)
self.bn1 = nn.BatchNorm2d(out_channels)
self.conv2 = nn.Conv2d(out_channels, out_channels, kernel_size=3, stride=1, padding=1, bias=False)
self.bn2 = nn.BatchNorm2d(out_channels)
self.downsample = downsample
def forward(self, x):
identity = x
out = self.conv1(x)
out = self.bn1(out)
out = F.relu(out)
out = self.conv2(out)
out = self.bn2(out)
if self.downsample is not None:
identity = self.downsample(x)
out += identity
out = F.relu(out)
return out
class ResNet(nn.Module):
def __init__(self, block, layers, num_classes=1000):
super(ResNet, self).__init__()
self.in_channels = 64
self.conv1 = nn.Conv2d(3, 64, kernel_size=7, stride=2, padding=3, bias=False)
self.bn1 = nn.BatchNorm2d(64)
self.maxpool = nn.MaxPool2d(kernel_size=3, stride=2, padding=1)
self.layer1 = self.make_layer(block, 64, layers[0])
self.layer2 = self.make_layer(block, 128, layers[1], stride=2)
self.layer3 = self.make_layer(block, 256, layers[2], stride=2)
self.layer4 = self.make_layer(block, 512, layers[3], stride=2)
self.avgpool = nn.AdaptiveAvgPool2d((1, 1))
self.fc = nn.Linear(512 * block.expansion, num_classes)
def make_layer(self, block, out_channels, blocks, stride=1):
downsample = None
if stride != 1 or self.in_channels != out_channels * block.expansion:
downsample = nn.Sequential(
nn.Conv2d(self.in_channels, out_channels * block.expansion, kernel_size=1, stride=stride, bias=False),
nn.BatchNorm2d(out_channels * block.expansion)
)
layers = []
layers.append(block(self.in_channels, out_channels, stride, downsample))
self.in_channels = out_channels * block.expansion
for i in range(1, blocks):
layers.append(block(self.in_channels, out_channels))
return nn.Sequential(*layers)
def forward(self, x):
x = self.conv1(x)
x = self.bn1(x)
x = F.relu(x)
x = self.maxpool(x)
x = self.layer1(x)
x = self.layer2(x)
x = self.layer3(x)
x = self.layer4(x)
x = self.avgpool(x)
x = x.view(x.size(0), -1)
x = self.fc(x)
return x
def ResNet34():
return ResNet(ResidualBlock, [3, 4, 6, 3])
```
ResNet34 是由4个 ResNet 模块组成,每个模块由多个残差块(Residual Block)组成。其中第一个模块使用1个3x3的卷积层,第2到第4个模块使用2个3x3的卷积层,卷积层后面跟着一个 Batch Normalization 层和 ReLU 激活函数。每个残差块包括两个3x3的卷积层,后面也跟着一个 Batch Normalization 层和 ReLU 激活函数。ResNet34 模型最后使用一个全局平均池化层,然后连接一个全连接层输出预测结果。
阅读全文
相关推荐
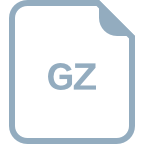
















