ls = s.split()
时间: 2024-02-12 08:06:51 浏览: 157
这段代码将字符串`s`按照空格分割成一个列表`ls`,其中每个元素都是以空格为分隔符的子字符串。具体来说,`s.split()`方法会将字符串`s`按照空格分割成多个子字符串,并将这些子字符串保存到一个列表中。如果`s`中存在连续的空格,`split()`方法会忽略这些空格,并将它们看作一个空字符串。
例如,如果`s`为`"hello world"`,那么`ls`将会是`['hello', 'world']`;如果`s`为`" hello world "`,那么`ls`也是`['hello', 'world']`。需要注意的是,`split()`方法默认使用空格作为分隔符,但也可以指定其他分隔符,例如:
```
s = "hello|world|python"
ls = s.split('|') # 使用竖线作为分隔符
print(ls) # 输出['hello', 'world', 'python']
```
需要注意的是,如果`s`为空字符串或者只包含空格,那么`split()`方法将返回一个空列表`[]`。
相关问题
s = "I love Python"ls = s.split()ls.reverse()print(ls)
该程序的输出结果是 `['Python', 'love', 'I']`。
程序首先将字符串 `s` 以空格为分隔符进行切割,得到一个包含三个元素的列表 `ls`,内容为 `['I', 'love', 'Python']`。然后使用 `reverse()` 方法将列表反转,得到的新列表为 `['Python', 'love', 'I']`。最后将该新列表输出,即为程序的结果。
Python中ls=s.split()啥意思
这行代码的意思是将一个字符串s按照空格分割成多个字符串,并将这些字符串存储在列表ls中。例如,如果s为"hello world",那么执行这行代码后,ls就会变成['hello', 'world']。可以通过以下代码来验证:
```
s = "hello world"
ls = s.split()
print(ls)
```
输出结果为:['hello', 'world']
阅读全文
相关推荐
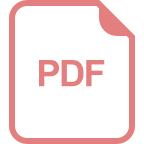
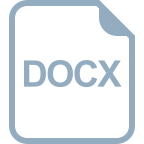
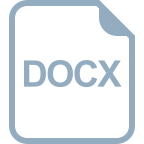














