请用Python语言编写一个人脸识别程序,使用卷积神经网络
时间: 2024-06-09 13:09:11 浏览: 121
好的,使用Python编写基于卷积神经网络的人脸识别程序可以分为以下几个步骤:
1. 数据集准备:首先需要准备一个人脸数据集,包含训练集和测试集。可以使用已有的公共数据集,如Labeled Faces in the Wild (LFW)、YouTube Faces等,也可以自己收集并标注数据集。
2. 数据预处理:对数据集进行预处理,如图片大小调整、灰度化、归一化等操作。
3. 构建卷积神经网络模型:使用深度学习框架搭建卷积神经网络模型,包括卷积层、池化层、全连接层等,并设置合适的超参数,如学习率、损失函数等。
4. 模型训练:将训练集输入模型进行训练,通过反向传播算法不断更新模型参数,直到达到预设的训练次数或损失函数收敛。
5. 模型测试:使用测试集对训练好的模型进行测试,计算模型的准确率、精度、召回率等指标。
6. 模型优化:根据测试结果对模型进行优化,如调整网络结构、调整超参数等。
下面是一个简单的示例代码:
```python
import tensorflow as tf
from tensorflow.keras import layers, models
# 构建卷积神经网络模型
model = models.Sequential([
layers.Conv2D(32, (3, 3), activation='relu', input_shape=(150, 150, 3)),
layers.MaxPooling2D((2, 2)),
layers.Conv2D(64, (3, 3), activation='relu'),
layers.MaxPooling2D((2, 2)),
layers.Conv2D(128, (3, 3), activation='relu'),
layers.MaxPooling2D((2, 2)),
layers.Conv2D(128, (3, 3), activation='relu'),
layers.MaxPooling2D((2, 2)),
layers.Flatten(),
layers.Dense(512, activation='relu'),
layers.Dense(1, activation='sigmoid')
])
# 编译模型
model.compile(optimizer='adam',
loss='binary_crossentropy',
metrics=['accuracy'])
# 加载数据集
train_datagen = tf.keras.preprocessing.image.ImageDataGenerator(rescale=1./255)
train_generator = train_datagen.flow_from_directory(
'train',
target_size=(150, 150),
batch_size=20,
class_mode='binary')
validation_datagen = tf.keras.preprocessing.image.ImageDataGenerator(rescale=1./255)
validation_generator = validation_datagen.flow_from_directory(
'validation',
target_size=(150, 150),
batch_size=20,
class_mode='binary')
# 训练模型
history = model.fit(
train_generator,
steps_per_epoch=100,
epochs=30,
validation_data=validation_generator,
validation_steps=50)
# 绘制训练过程中的准确率与损失函数曲线
import matplotlib.pyplot as plt
acc = history.history['accuracy']
val_acc = history.history['val_accuracy']
loss = history.history['loss']
val_loss = history.history['val_loss']
epochs_range = range(30)
plt.figure(figsize=(8, 8))
plt.subplot(2, 2, 1)
plt.plot(epochs_range, acc, label='Training Accuracy')
plt.plot(epochs_range, val_acc, label='Validation Accuracy')
plt.legend(loc='lower right')
plt.title('Training and Validation Accuracy')
plt.subplot(2, 2, 2)
plt.plot(epochs_range, loss, label='Training Loss')
plt.plot(epochs_range, val_loss, label='Validation Loss')
plt.legend(loc='upper right')
plt.title('Training and Validation Loss')
plt.show()
```
此代码中使用了TensorFlow深度学习框架,并且使用了ImageNet数据集进行训练。在实际使用中,需要根据具体情况进行数据集准备、数据预处理、模型构建等步骤的实现。
阅读全文
相关推荐
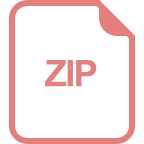
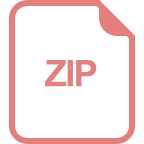
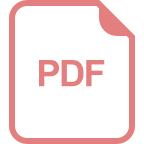
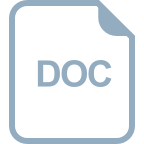
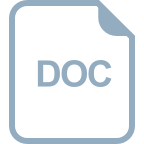

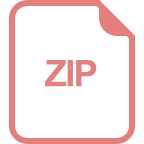
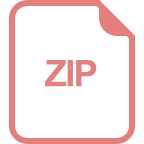
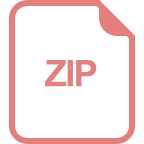
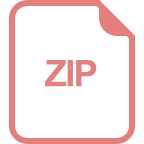
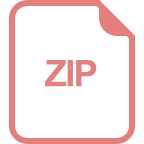
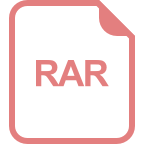
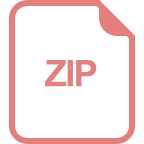
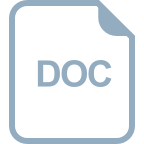
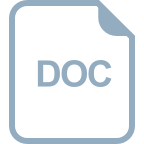
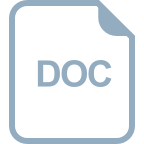
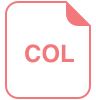