TVM的Python代码上可以直接使用OpenMP吗
时间: 2024-04-27 11:21:24 浏览: 12
可以的。TVM在Python上使用OpenMP非常方便,只需要在TVM的Python代码中添加相应的注释即可。具体来说,可以使用以下注释:
1. 在函数定义前添加“@tvm.target('llvm -mcpu=corei7-avx -mattr=+avx2')”注释,指定使用LLVM作为后端编译器,使用OpenMP并行化,其中-mcpu和-mattr选项可以根据硬件平台进行调整。
2. 在需要并行化的代码块前添加“with tvm.target.create('llvm -mcpu=corei7-avx -mattr=+avx2'):”注释,指定使用LLVM作为后端编译器,并使用OpenMP并行化。
3. 在需要并行化的循环前添加“tvm.te.thread_axis('threadIdx.x')”注释,指定使用OpenMP并行化循环。
使用以上注释,就可以在TVM的Python代码中方便地使用OpenMP并行化,充分利用多核CPU的性能,提高代码执行效率。需要注意的是,有些Python环境可能需要进行额外的配置才能使用OpenMP并行化,例如设置环境变量等。
相关问题
TVM如何使用OpenMP实现并行
TVM是一个深度学习编译器和运行时库,可以将深度学习模型编译为不同硬件上的高效代码。TVM支持多种硬件和软件后端,包括CPU、GPU、FPGA、OpenCL等。在CPU上使用OpenMP实现并行化是TVM的一种常见做法。
具体来说,TVM支持使用OpenMP加速CPU上的计算。在TVM中,可以通过调用TVM的autotvm模块来自动优化模型并生成高效的代码。在生成代码时,可以通过设置TVM的target参数来选择使用OpenMP并行化。例如,以下代码将使用OpenMP并行化生成CPU上的代码:
``` python
import tvm
from tvm import autotvm
# 定义模型和数据
@autotvm.template
def my_model(N, M, K):
A = tvm.placeholder((N, K), name='A')
B = tvm.placeholder((K, M), name='B')
k = tvm.reduce_axis((0, K), 'k')
C = tvm.compute((N, M), lambda i, j: tvm.sum(A[i, k] * B[k, j], axis=k), name='C')
s = tvm.create_schedule(C.op)
# 设置并行化
xo, yo, xi, yi = s[C].tile(C.op.axis[0], C.op.axis[1], 32, 32)
s[C].parallel(xo)
return s, [A, B, C]
# 设置TVM运行环境和目标硬件
ctx = tvm.context('llvm', 0)
target = tvm.target.Target('llvm -mcpu=core-avx2 -mtriple=x86_64-linux-gnu')
# 自动优化模型并生成代码
task = autotvm.task.create(my_model, args=(1024, 1024, 1024), target=target)
measure_option = autotvm.measure_option(builder='local', runner=autotvm.LocalRunner(number=5))
tuner = autotvm.tuner.XGBTuner(task)
tuner.tune(n_trial=1000, measure_option=measure_option)
# 编译生成模型
with tvm.transform.PassContext(opt_level=3):
lib = relay.build(my_model(1024, 1024, 1024), target=target)
```
在上述代码中,使用TVM的autotvm模块自动优化模型并生成代码,其中设置了使用OpenMP并行化,并行程度为32。最后使用TVM的relay.build()函数编译生成模型。当运行生成的模型时,TVM会自动使用OpenMP并行化加速模型计算。
Python用TVM实现GPU上并行矩阵乘法
以下是使用Python和TVM实现GPU上并行矩阵乘法的简单示例:
```python
import numpy as np
import tvm
from tvm import te, autotvm
# 定义矩阵乘法运算
def matmul(n, m, p):
# 定义输入矩阵
A = te.placeholder((n, m), name='A')
B = te.placeholder((m, p), name='B')
# 定义输出矩阵
k = te.reduce_axis((0, m), name='k')
C = te.compute((n, p), lambda i, j: te.sum(A[i, k] * B[k, j], axis=k), name='C')
# 创建计算图
s = te.create_schedule(C.op)
# 选择目标设备
target = 'cuda'
# 编译
func = tvm.build(s, [A, B, C], target=target)
# 生成随机输入数据
a_np = np.random.uniform(size=(n, m)).astype(np.float32)
b_np = np.random.uniform(size=(m, p)).astype(np.float32)
c_np = np.zeros((n, p), dtype=np.float32)
# 将数据上传到GPU
ctx = tvm.gpu()
a_tvm = tvm.nd.array(a_np, ctx)
b_tvm = tvm.nd.array(b_np, ctx)
c_tvm = tvm.nd.array(c_np, ctx)
# 执行
func(a_tvm, b_tvm, c_tvm)
tvm.testing.assert_allclose(c_np, c_tvm.asnumpy(), rtol=1e-5)
# 测试
matmul(128, 256, 512)
```
以上代码中,我们首先使用TVM的API定义了矩阵乘法运算,并创建了计算图。然后,我们选择了目标设备为GPU,并使用TVM的编译器将计算图编译为针对GPU的高效代码。接着,我们生成了随机的输入数据,并将其上传到GPU。最后,我们调用生成的代码执行矩阵乘法运算,并将输出结果与预期结果进行比对。
需要注意的是,在实际使用中,我们可能需要使用TVM的调度器对计算图进行优化,以进一步提高性能。同时,我们还可以使用TVM的自动调优工具Autotvm,自动选择最优的计算图和参数组合,以获得最佳性能。
相关推荐
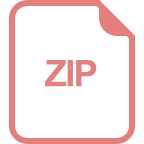
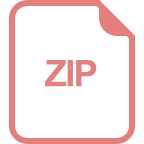
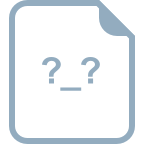












