三.蝠鲼觅食优化算法MRFO
时间: 2023-09-28 20:10:27 浏览: 55
蝠鲼觅食优化算法(Marine Ray Fish Swarm Optimization,简称MRFO)是一种基于自然界中蝠鲼觅食行为的优化算法。它模拟了蝠鲼在海洋中觅食的过程,通过不断调整搜索策略,使得搜索过程更加高效。MRFO算法的主要思想是利用蝠鲼在觅食过程中的多种行为策略,包括探测、追踪、逃避等,来优化目标函数。相比于其他优化算法,MRFO算法具有较强的全局搜索能力和收敛速度。
相关问题
三.蝠鲼觅食优化算法MRFO伪代码
以下是蝠鲼觅食优化算法MRFO的伪代码:
```
Initialize the population of bats
While stopping criterion is not met:
For each bat i in the population:
Generate a new solution x_i by updating the bat's position:
x_i = x_i + A*sin(2*pi*f)*|r_i,j - x_j|
where A is the loudness of the bat, f is the frequency, r_i,j is the position of a randomly selected bat, and x_j is the position of the best bat in the population
If the new solution x_i is better than the current solution x_i, replace x_i with the new solution
With probability r_i, generate a new solution x_i by random walk:
x_i = x_i + epsilon_i
where epsilon_i is a vector of random values drawn from a normal distribution
Update the loudness A and frequency f of the bat:
A = alpha*A
f = f_min + (f_max - f_min)*rand()
If the loudness A is less than a threshold value A_min, reset the bat's position to a new random solution
If the new solution x_i is better than the best solution in the population, update the best solution
End for
End while
```
其中,蝠鲼觅食优化算法通过模拟蝙蝠觅食的行为来进行优化。每个蝙蝠代表一个潜在解,其位置表示解的参数值。蝙蝠根据当前最优解和其他蝙蝠的位置信息来更新自己的位置。此外,每个蝙蝠还具有一个“响度”和“频率”的属性,可以调整其搜索范围和速度。在搜索过程中,每个蝙蝠还有一定概率进行随机游走以增加搜索多样性。最终,算法的输出结果是最优解。
蝠鲼觅食优化算法MRFO原理
蝠鲼觅食优化算法(MRFO)的原理是模拟蝠鲼在觅食过程中的搜索行为,通过自适应调整蝠鲼的搜索策略,来优化解的搜索过程。具体来说,MRFO算法包括以下步骤:
1. 初始化种群:随机生成一定数量的蝠鲼,并随机分配初始位置和速度。
2. 蝠鲼搜索行为:在每一次搜索迭代中,每一只蝠鲼都会根据自身的位置和速度,计算出新的位置和速度,并根据一定的策略来调整搜索行为。
3. 更新种群:根据每一只蝠鲼的适应度值,更新全局最优解和个体最优解。
4. 改进搜索策略:根据全局最优解和个体最优解,自适应地调整蝠鲼的搜索策略,以提高搜索效率。
5. 判断终止条件:在达到一定的搜索次数或者达到一定的解精度时,结束搜索过程并输出最优解。
总的来说,MRFO算法利用了蝠鲼在觅食过程中搜索范围和搜索速度的自适应调整机制,能够有效地避免陷入局部最优解,提高全局搜索能力和搜索效率,因此在解决多种优化问题上具有一定的优势。
相关推荐
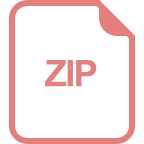
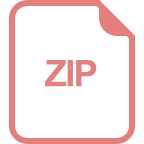





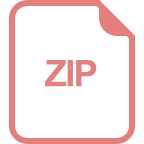
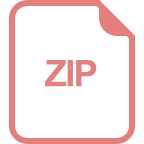
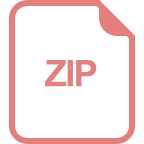
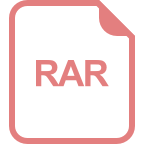
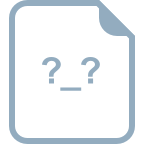
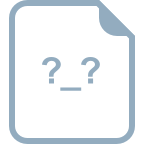
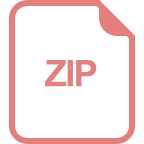