python实现k-means算法全部过程
时间: 2023-01-30 21:49:53 浏览: 61
k-means 是一种聚类算法,用于将数据分成 k 个不同的簇。
假设你有一个数据集,包含了 n 个数据点,每个数据点有 m 个特征。首先,你需要随机初始化 k 个质心,每个质心表示一个簇。然后,你需要执行以下步骤,直到质心不再改变或者达到最大迭代次数:
1. 计算每个数据点到每个质心的欧几里得距离,并将每个数据点分配到距离它最近的质心所在的簇中。
2. 重新计算每个簇的质心。
下面是 k-means 的 python 代码实现:
```python
from typing import List, Tuple
import numpy as np
def kmeans(X: List[List[float]], k: int, max_iter: int) -> Tuple[List[int], List[List[float]]]:
"""
Perform k-means clustering on the data.
Parameters
----------
X : list of list of float
The data to cluster, where each element is a data point with m features.
k : int
The number of clusters.
max_iter : int
The maximum number of iterations.
Returns
-------
labels : list of int
The cluster labels for each data point.
centroids : list of list of float
The k centroids.
"""
# Initialize the centroids randomly
n, m = len(X), len(X[0])
centroids = [[np.random.random() for _ in range(m)] for _ in range(k)]
labels = [0 for _ in range(n)]
for _ in range(max_iter):
# Assign each data point to the closest centroid
for i, x in enumerate(X):
distances = [euclidean_distance(x, c) for c in centroids]
labels[i] = distances.index(min(distances))
# Recompute the centroids
centroids = [np.zeros(m
阅读全文
相关推荐
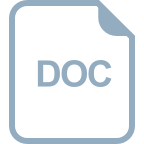
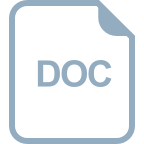
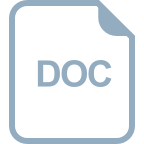
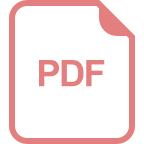
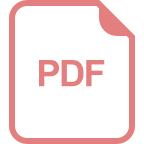
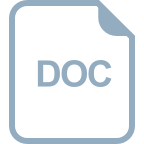
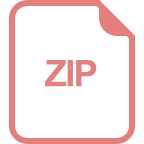
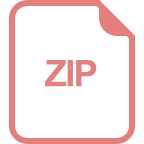
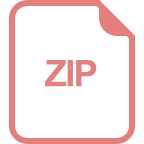
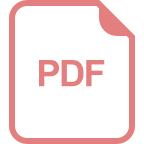
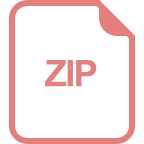
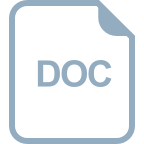
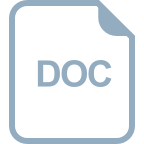



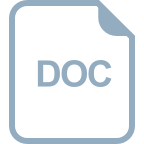
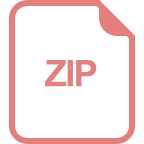