deeplearning radiomics
时间: 2023-09-04 10:14:37 浏览: 53
深度学习在放射学中的应用广泛,其中之一就是放射学影像特征学(Radiomics)。Radiomics 是一种从医学影像中提取大量定量特征的方法,通过这些特征可以揭示影像中的潜在信息,以辅助诊断、预后评估和治疗决策等。深度学习在Radiomics中发挥了重要作用,它可以自动学习和提取影像特征,为医生提供更准确、快速的分析结果。
深度学习方法在Radiomics中的应用包括图像分割、特征提取和预测模型的构建等。例如,使用深度学习进行肿瘤分割可以帮助医生更精确地判断肿瘤的位置和大小;使用深度学习提取影像特征可以辅助医生进行疾病诊断和分型;使用深度学习构建预测模型可以根据影像特征预测患者的生存期或治疗效果等。
需要注意的是,深度学习在Radiomics中的应用还处于不断发展阶段,尚需进一步研究和验证其可行性和有效性。同时,由于医学影像数据的特殊性,深度学习在Radiomics中的应用也面临一些挑战,例如数据标注、模型解释性和数据隐私等问题。因此,综合考虑实际需求和限制条件,选择合适的方法和策略是非常重要的。
相关问题
radiomics score
radiomics score(放射医学分数)是一种计算用于评估医学影像的指标,通过分析图像中的数字特征来预测疾病的发生、发展和治疗效果等信息。放射医学分数主要应用于医学影像学中,如CT扫描和MRI等。
放射医学分数的计算过程包括四个主要步骤。首先,医学影像获取后,通过图像分割提取感兴趣区域(ROI)。然后,从ROI中提取一系列定量特征(radiomics features),如形状、纹理和密度等。接下来,对这些特征进行特征选择和降维处理,以减少冗余和噪声。最后,使用机器学习算法或其他数学模型对所选特征进行分析并生成放射医学分数。
radiomics score的应用非常广泛。首先,它可以用来帮助医生进行疾病的诊断和分类。例如,在肿瘤学中,radiomics score可以用于辅助肿瘤的分类和分级。其次,radiomics score可以用于预测疾病的进展和治疗效果。例如,在癌症治疗中,通过分析影像的radiomics score可以预测患者的预后和治疗反应。此外,radiomics score还可以用于辅助临床试验的设计和结果评估。
总而言之,radiomics score是一种通过计算医学影像中的数字特征来评估疾病的指标。它可以为医生提供有关疾病诊断、预后和治疗效果等方面的信息,对于改善临床决策和个体化治疗具有重要意义。
安装radiomics
安装radiomics需要先安装Python和pip,在命令行中输入以下命令即可安装:
```
pip install radiomics
```
如果想要安装特定版本的radiomics,可以使用以下命令:
```
pip install radiomics==version_number
```
其中,version_number为具体的版本号。
相关推荐
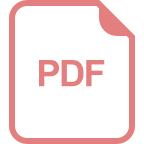













