用pytorch实现车牌识别
时间: 2023-12-19 10:29:57 浏览: 54
基于引用[1]和引用,使用pytorch实现车牌识别的步骤如下:
1.使用YOLOv4或YOLOv5网络进行车辆检测,得到车辆的位置信息。
2.使用LPRNet网络进行车牌检测,得到车牌的位置信息。
3.对车牌进行图像处理,例如旋转、缩放等操作,使车牌图像更加清晰。
4.使用LPRNet网络进行车牌识别,得到车牌号码。
下面是一个使用pytorch实现车牌识别的代码示例:
```python
# 导入相关库
import cv2
import torch
import numpy as np
from models import *
from utils.datasets import *
from utils.utils import *
# 设置相关参数
device = torch.device('cuda' if torch.cuda.is_available() else 'cpu')
img_size = 416
conf_thres = 0.5
nms_thres = 0.4
# 加载YOLOv5模型
model = attempt_load('yolov5s.pt', map_location=device)
model.to(device).eval()
# 加载LPRNet模型
lprnet = LPRNet(class_num=len(alphabet) + 1, dropout_rate=0)
lprnet.load_state_dict(torch.load('LPRNet.pth', map_location=device))
lprnet.to(device).eval()
# 加载车牌字符集
alphabet = '0123456789abcdefghijklmnopqrstuvwxyz'
# 加载测试图片
img = cv2.imread('test.jpg')
img0 = img.copy()
# 图片预处理
img = letterbox(img, new_shape=img_size)[0]
img = img[:, :, ::-1].transpose(2, 0, 1)
img = np.ascontiguousarray(img)
# 将图片转换为Tensor
img = torch.from_numpy(img).to(device).float()
img /= 255.0
# 对图片进行目标检测
pred = model(img.unsqueeze(0))[0]
pred = non_max_suppression(pred, conf_thres, nms_thres)[0]
# 遍历所有检测到的车辆
for det in pred:
if det is not None:
# 获取车辆的位置信息
det[:, :4] = scale_coords(img.shape[2:], det[:, :4], img0.shape).round()
# 获取车辆图像
crop_img = img0[int(det[1]):int(det[3]), int(det[0]):int(det[2])]
# 对车牌进行检测
plate, _, _, _ = lprnet.inference(crop_img, 0.5)
# 对车牌进行识别
plate = ''.join([alphabet[int(x)] for x in plate])
print('车牌号码:', plate)
# 显示结果
cv2.imshow('result', img0)
cv2.waitKey(0)
cv2.destroyAllWindows()
```
相关推荐
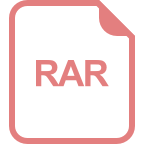
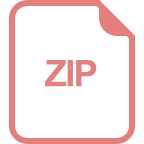
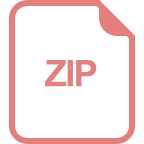














