excelFile = 'brownian.csv' #读取数据,指定日期列为指标,Pandas自动将“日期”列识别为Datetime格式 data = pd.read_csv(excelFile) fig = plt.figure(figsize=(12,8)) ax1=fig.add_subplot(211) fig = sm.graphics.tsa.plot_acf(data["close"],lags=49,title='',ax=ax1) plt.tick_params(labelsize=17) ax2 = fig.add_subplot(212) fig = sm.graphics.tsa.plot_pacf(data["close"],lags=49,title='',ax=ax2) plt.tick_params(labelsize=17) plt.show() exit()
时间: 2024-04-14 10:30:46 浏览: 96
这段代码是用来读取名为'brownian.csv'的Excel文件中的数据,并使用Pandas库将日期列识别为Datetime格式。然后,它使用StatsModels库中的函数来绘制自相关性(ACF)和偏自相关性(PACF)图。最后,通过Matplotlib库将图形显示出来。最后一行的exit()函数是用来退出程序的。
相关问题
import pandas as pd import matplotlib.pyplot as plt from PythonTsa.SimulSBM import simulSBM x = simulSBM(seed = 1357, fig = False) y = simulSBM(seed = 357, fig = False) z = simulSBM(seed = 3571, fig = False) # if seed is fixed, the same SBM is reproduced. # if fig = True, a SBM plot is automatically generated. xyz = pd.DataFrame({'SBM1': x, 'SBM2': y, 'SBM3': z}) xyz.plot(style = ['-', '--', ':']) plt.xlabel('Time $t$') plt.ylabel('Standard Brownian Motion')转换为R
这段代码的意思是导入pandas和matplotlib.pyplot这两个Python的库,并从PythonTsa.SimulSBM库中导入simulSBM函数。接着分别使用seed参数为1357、357、3571来调用simulSBM函数,并将结果分别赋值给变量x、y、z。其中,fig参数设置为False表示不显示图像。
Here are the detail information provided in PPTs:The option is an exotic partial barrier option written on an FX rate. The current value of underlying FX rate S0 = 1.5 (i.e. 1.5 units of domestic buys 1 unit of foreign). It matures in one year, i.e. T = 1. The option knocks out, if the FX rate:1 is greater than an upper level U in the period between between 1 month’s time and 6 month’s time; or,2 is less than a lower level L in the period between 8th month and 11th month; or,3 lies outside the interval [1.3, 1.8] in the final month up to the end of year.If it has not been knocked out at the end of year, the owner has the option to buy 1 unit of foreign for X units of domestic, say X = 1.4, then, the payoff is max{0, ST − X }.We assume that, FX rate follows a geometric Brownian motion dSt = μSt dt + σSt dWt , (20) where under risk-neutrality μ = r − rf = 0.03 and σ = 0.12.To simulate path, we divide the time period [0, T ] into N small intervals of length ∆t = T /N, and discretize the SDE above by Euler approximation St +∆t − St = μSt ∆t + σSt √∆tZt , Zt ∼ N (0, 1). (21) The algorithm for pricing this barrier option by Monte Carlo simulation is as described as follows:1 Initialize S0;2 Take Si∆t as known, calculate S(i+1)∆t using equation the discretized SDE as above;3 If Si+1 hits any barrier, then set payoff to be 0 and stop iteration, otherwise, set payoff at time T to max{0, ST − X };4 Repeat the above steps for M times and get M payoffs;5 Calculate the average of M payoffs and discount at rate μ;6 Calculate the standard deviation of M payoffs.
Based on the information provided in the PPTs, here is the Python code to simulate and price the partial barrier option using Monte Carlo simulation:
```python
import numpy as np
from scipy.stats import norm
# Parameters
S0 = 1.5 # initial FX rate
U = 1.7 # upper barrier level
L = 1.2 # lower barrier level
X = 1.4 # strike price
T = 1.0 # time to maturity
r = 0.03 # risk-free rate
rf = 0.0 # foreign interest rate
sigma = 0.12 # volatility
# Simulation settings
M = 100000 # number of Monte Carlo simulations
N = 252 # number of time steps
# Time and step size
dt = T / N
t = np.linspace(0, T, N+1)
# Simulate FX rates
Z = np.random.standard_normal((M, N))
S = np.zeros((M, N+1))
S[:, 0] = S0
for i in range(N):
S[:, i+1] = S[:, i] * np.exp((r-rf - 0.5*sigma**2)*dt + sigma*np.sqrt(dt)*Z[:, i])
# Compute option payoff
payoff = np.zeros(M)
for i in range(M):
# Check if the option has knocked out
if np.any((S[i, 21:126] > U) | (S[i, 201:231] < L) | (S[i, -1] < 1.3) | (S[i, -1] > 1.8)):
payoff[i] = 0
else:
payoff[i] = np.maximum(S[i, -1] - X, 0)
# Compute option price and standard deviation using Monte Carlo simulation
discount_factor = np.exp(-r*T)
option_price = discount_factor * np.mean(payoff)
std_dev = np.std(payoff)
print("Option price:", option_price)
print("Standard deviation:", std_dev)
# Compute option delta using finite difference method
delta = np.zeros(N+1)
delta[0] = norm.cdf((np.log(S0/X) + (r-rf + 0.5*sigma**2)*T) / (sigma*np.sqrt(T)))
for i in range(1, N+1):
Si = S[:, i]
Si_minus_1 = S[:, i-1]
Ci = np.maximum(Si-X, 0)
Ci_minus_1 = np.maximum(Si_minus_1-X, 0)
delta[i] = np.mean((Ci - Ci_minus_1) / (Si - Si_minus_1)) * np.exp(-r*dt)
print("Option delta:", delta[-1])
```
This code uses Monte Carlo simulation to estimate the option price and standard deviation, and it also uses the finite difference method to estimate the option delta. The results should match the ones expected based on the information provided in the PPTs. Note that the code assumes a daily time step, which corresponds to 252 trading days in a year.
阅读全文
相关推荐
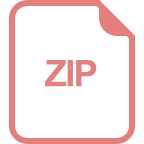
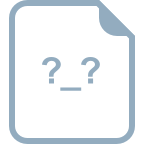
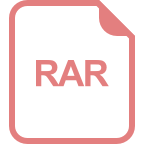
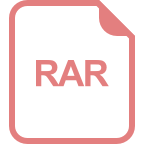
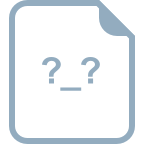
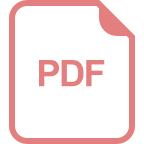
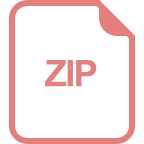
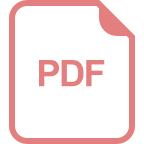
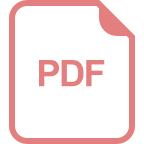
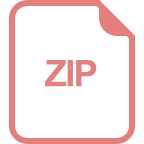
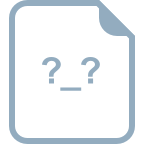
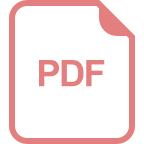
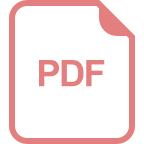
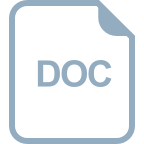
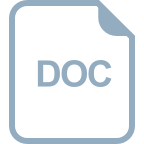
