pca主成分分析几篇文献
时间: 2024-11-02 13:08:35 浏览: 21
PCA(Principal Component Analysis,主成分分析)是一种常用的数据降维技术,在统计学和机器学习领域广泛应用。它通过线性变换将原始数据转换到新的坐标系,新坐标系下的方向(主成分)最大化了数据方差。以下是几篇经典的关于PCA的文献推荐:
1. **Jolliffe, I.T. (2002)**,《Principal Component Analysis**》** - 这是一本经典教材,详细介绍了PCA的基本理论和应用方法。
2. **Wold, S., Esbensen, K., & Geladi, P. (1987)**,《Soft Computing in Chemometrics**》** - 第二章专门讨论了PCA,对于化学计量学的应用特别有价值。
3. **Tipping, M.E., & Bishop, C.M. (1999)**,《Probabilistic Principal Component Analysis**》** - 提出了一种概率模型解释PCA,并将其扩展到了高维数据。
4. **Hotelling, H. (1933)**,“Analysis of a complex of statistical variables into principal components” - PCA最初由Hotelling提出,他的工作奠定了基础。
5. **Pearson, K. (1901)**,“LIII. On Lines and Planes of Closest Fit to Systems of Points in Space” - 尽管不是完全关于PCA,但Pearson的工作对PCA的发展有影响。
如果你想深入研究PCA的最新进展和技术应用,可以查阅最近几年的数据挖掘、机器学习会议如NIPS、ICML或IJCAI的论文,以及顶级期刊如Journal of Machine Learning Research和Pattern Recognition Letters。
阅读全文
相关推荐
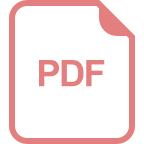
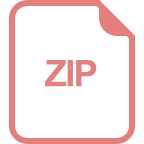
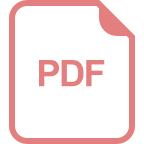
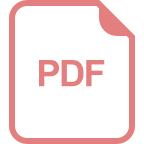
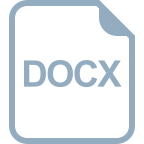
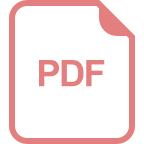
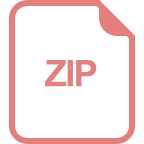
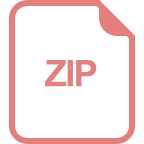
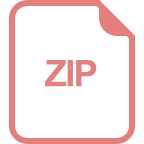
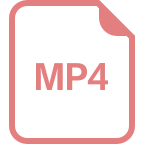
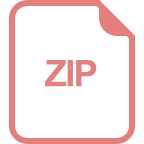
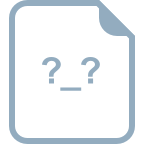
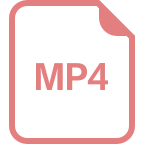
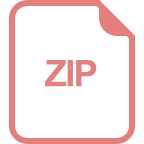
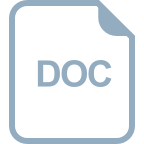
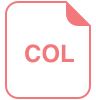
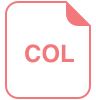
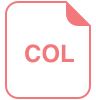
