y_pred = model.predict(x_test) # y_pred = np.squeeze(y_pred) plt.imshow(y_pred, cmap='gray') # cmap='gray' 表示以灰度图显示 plt.axis('off') # 禁用轴坐标 plt.tight_layout() save_path = forecast_path + '/' + images[0].split('/')[-1].split('.')[0] + '_forecast.png' plt.savefig(save_path) 以上代码保存的图片仍然带有白边
时间: 2024-04-21 07:22:27 浏览: 14
如果调用`plt.tight_layout()`后仍然存在白边,你可以尝试使用`bbox_inches='tight'`参数来保存图片。将以下代码替换你的保存图片的代码部分:
```python
plt.savefig(save_path, bbox_inches='tight')
```
这将使用更紧凑的边界框来保存图片,从而消除白边。重新运行代码,应该能够得到没有白边的图片。
相关问题
import numpy as np import matplotlib.pyplot as plt from keras.models import Sequential from keras.layers import Dense, Flatten, Conv1D, MaxPooling1D from keras import backend as K # 生成正弦函数数据 x = np.linspace(0, 100, 1000) y = np.sin(2*x) # 将数据转换为卷积神经网络需要的格式 X = np.zeros((len(x), 10)) for i in range(len(x)): for j in range(10): X[i][j] = y[(i+j)%len(x)] X = np.reshape(X, (X.shape[0], X.shape[1], 1)) # 构建卷积神经网络模型 model = Sequential() model.add(Conv1D(filters=32, kernel_size=3, activation='relu', input_shape=(10,1))) model.add(MaxPooling1D(pool_size=2)) model.add(Flatten()) model.add(Dense(100, activation='relu')) model.add(Dense(1, activation='linear')) # 打印模型结构 model.summary() # 编译模型 model.compile(loss='mse', optimizer='adam') # 训练模型并可视化损失函数 history = model.fit(X, y, epochs=100, batch_size=32, validation_split=0.2) loss = history.history['loss'] val_loss = history.history['val_loss'] epochs = range(1, len(loss)+1) plt.plot(epochs, loss, 'bo', label='Training loss') plt.plot(epochs, val_loss, 'b', label='Validation loss') plt.title('Training and validation loss') plt.xlabel('Epochs') plt.ylabel('Loss') plt.legend() plt.show() # 预测并可视化结果 y_pred = model.predict(X) plt.plot(x, y, label='true') plt.plot(x, y_pred, label='predict') plt.legend() plt.show() # 定义一个函数,用于获取卷积层的输出 get_conv_output = K.function([model.layers[0].input], [model.layers[0].output]) # 获取卷积层的输出 conv_output = get_conv_output([X])[0] # 将输出可视化 plt.figure(figsize=(10, 10)) for i in range(32): plt.subplot(4, 8, i+1) plt.imshow(np.squeeze(conv_output[:, :, i]), cmap='gray') plt.show()分析下代码
这段代码使用 Keras 构建了一个卷积神经网络模型,用于对正弦函数数据进行回归预测,并可视化训练过程和预测结果。具体分析如下:
1. 导入所需的库:
```python
import numpy as np
import matplotlib.pyplot as plt
from keras.models import Sequential
from keras.layers import Dense, Flatten, Conv1D, MaxPooling1D
from keras import backend as K
```
2. 生成正弦函数数据:
```python
x = np.linspace(0, 100, 1000)
y = np.sin(2*x)
```
3. 将数据转换为卷积神经网络需要的格式:
```python
X = np.zeros((len(x), 10))
for i in range(len(x)):
for j in range(10):
X[i][j] = y[(i+j)%len(x)]
X = np.reshape(X, (X.shape[0], X.shape[1], 1))
```
4. 构建卷积神经网络模型:
```python
model = Sequential()
model.add(Conv1D(filters=32, kernel_size=3, activation='relu', input_shape=(10,1)))
model.add(MaxPooling1D(pool_size=2))
model.add(Flatten())
model.add(Dense(100, activation='relu'))
model.add(Dense(1, activation='linear'))
```
该模型包含一个卷积层、一个池化层、一个展开层、两个全连接层。输入数据的形状为 (10, 1),其中 10 表示每次输入 10 个时间步的数据,1 表示每个时间步只有一个特征。输出数据的形状为 (1, ),表示模型输出一个标量。
5. 打印模型结构:
```python
model.summary()
```
6. 编译模型:
```python
model.compile(loss='mse', optimizer='adam')
```
7. 训练模型并可视化损失函数:
```python
history = model.fit(X, y, epochs=100, batch_size=32, validation_split=0.2)
loss = history.history['loss']
val_loss = history.history['val_loss']
epochs = range(1, len(loss)+1)
plt.plot(epochs, loss, 'bo', label='Training loss')
plt.plot(epochs, val_loss, 'b', label='Validation loss')
plt.title('Training and validation loss')
plt.xlabel('Epochs')
plt.ylabel('Loss')
plt.legend()
plt.show()
```
8. 预测并可视化结果:
```python
y_pred = model.predict(X)
plt.plot(x, y, label='true')
plt.plot(x, y_pred, label='predict')
plt.legend()
plt.show()
```
9. 获取卷积层的输出并可视化:
```python
get_conv_output = K.function([model.layers[0].input], [model.layers[0].output])
conv_output = get_conv_output([X])[0]
plt.figure(figsize=(10, 10))
for i in range(32):
plt.subplot(4, 8, i+1)
plt.imshow(np.squeeze(conv_output[:, :, i]), cmap='gray')
plt.show()
```
该部分代码定义了一个函数 get_conv_output,用于获取卷积层的输出。然后通过该函数获取卷积层的输出,并将其可视化。具体来说,首先通过 get_conv_output 函数获取卷积层的输出,然后将其可视化为 32 幅灰度图像,其中每幅图像对应一个卷积核的输出。
这是对单个文件进行预测“import os import json import torch from PIL import Image from torchvision import transforms import matplotlib.pyplot as plt from model import convnext_tiny as create_model def main(): device = torch.device("cuda:0" if torch.cuda.is_available() else "cpu") print(f"using {device} device.") num_classes = 5 img_size = 224 data_transform = transforms.Compose( [transforms.Resize(int(img_size * 1.14)), transforms.CenterCrop(img_size), transforms.ToTensor(), transforms.Normalize([0.485, 0.456, 0.406], [0.229, 0.224, 0.225])]) # load image img_path = "../tulip.jpg" assert os.path.exists(img_path), "file: '{}' dose not exist.".format(img_path) img = Image.open(img_path) plt.imshow(img) # [N, C, H, W] img = data_transform(img) # expand batch dimension img = torch.unsqueeze(img, dim=0) # read class_indict json_path = './class_indices.json' assert os.path.exists(json_path), "file: '{}' dose not exist.".format(json_path) with open(json_path, "r") as f: class_indict = json.load(f) # create model model = create_model(num_classes=num_classes).to(device) # load model weights model_weight_path = "./weights/best_model.pth" model.load_state_dict(torch.load(model_weight_path, map_location=device)) model.eval() with torch.no_grad(): # predict class output = torch.squeeze(model(img.to(device))).cpu() predict = torch.softmax(output, dim=0) predict_cla = torch.argmax(predict).numpy() print_res = "class: {} prob: {:.3}".format(class_indict[str(predict_cla)], predict[predict_cla].numpy()) plt.title(print_res) for i in range(len(predict)): print("class: {:10} prob: {:.3}".format(class_indict[str(i)], predict[i].numpy())) plt.show() if __name__ == '__main__': main()”,改为对指定文件夹下的左右文件进行预测,并绘制混淆矩阵
好的,你的问题是如何将上述代码修改为对指定文件夹下的所有文件进行预测,并绘制混淆矩阵。
要实现这个功能,你需要进行以下几个步骤:
1. 导入必要的包:
```python
import os
import json
import torch
from PIL import Image
from torchvision import transforms
from sklearn.metrics import confusion_matrix
import matplotlib.pyplot as plt
import numpy as np
from model import convnext_tiny as create_model
```
2. 定义函数 `predict_folder`,用于对指定文件夹下的所有文件进行预测:
```python
def predict_folder(folder_path, model_weight_path, json_path, img_size, num_classes, device):
data_transform = transforms.Compose([
transforms.Resize(int(img_size * 1.14)),
transforms.CenterCrop(img_size),
transforms.ToTensor(),
transforms.Normalize([0.485, 0.456, 0.406], [0.229, 0.224, 0.225])
])
# read class_indict
with open(json_path, "r") as f:
class_indict = json.load(f)
# create model
model = create_model(num_classes=num_classes).to(device)
# load model weights
model.load_state_dict(torch.load(model_weight_path, map_location=device))
model.eval()
y_true = []
y_pred = []
for root, dirs, files in os.walk(folder_path):
for file in files:
img_path = os.path.join(root, file)
assert os.path.exists(img_path), "file: '{}' dose not exist.".format(img_path)
img = Image.open(img_path)
# [N, C, H, W]
img = data_transform(img)
# expand batch dimension
img = torch.unsqueeze(img, dim=0)
with torch.no_grad():
# predict class
output = torch.squeeze(model(img.to(device))).cpu()
predict = torch.softmax(output, dim=0)
predict_cla = torch.argmax(predict).numpy()
y_true.append(class_indict[os.path.basename(root)])
y_pred.append(predict_cla)
return y_true, y_pred
```
这个函数接受五个参数:
- `folder_path`:要预测的文件夹路径。
- `model_weight_path`:模型权重文件路径。
- `json_path`:类别标签文件路径。
- `img_size`:输入图片的大小。
- `num_classes`:分类器的类别数。
- `device`:设备类型。
函数会返回两个列表 `y_true` 和 `y_pred`,分别代表真实标签和预测标签。
3. 加载类别标签:
```python
json_path = './class_indices.json'
assert os.path.exists(json_path), "file: '{}' dose not exist.".format(json_path)
with open(json_path, "r") as f:
class_indict = json.load(f)
```
4. 调用 `predict_folder` 函数进行预测:
```python
folder_path = './test'
assert os.path.exists(folder_path), "folder: '{}' dose not exist.".format(folder_path)
y_true, y_pred = predict_folder(folder_path, "./weights/best_model.pth", json_path, 224, 5, device)
```
这里假设要预测的文件夹路径为 `./test`,模型权重文件路径为 `./weights/best_model.pth`,输入图片大小为 224,分类器的类别数为 5。
5. 绘制混淆矩阵:
```python
cm = confusion_matrix(y_true, y_pred)
fig, ax = plt.subplots()
im = ax.imshow(cm, interpolation='nearest', cmap=plt.cm.Blues)
ax.figure.colorbar(im, ax=ax)
ax.set(xticks=np.arange(cm.shape[1]),
yticks=np.arange(cm.shape[0]),
xticklabels=list(class_indict.values()), yticklabels=list(class_indict.values()),
title='Confusion matrix',
ylabel='True label',
xlabel='Predicted label')
plt.setp(ax.get_xticklabels(), rotation=45, ha="right",
rotation_mode="anchor")
fmt = 'd'
thresh = cm.max() / 2.
for i in range(cm.shape[0]):
for j in range(cm.shape[1]):
ax.text(j, i, format(cm[i, j], fmt),
ha="center", va="center",
color="white" if cm[i, j] > thresh else "black")
fig.tight_layout()
plt.show()
```
这里使用了 `sklearn.metrics` 中的 `confusion_matrix` 函数进行混淆矩阵的计算。然后使用 `matplotlib` 绘制混淆矩阵图像。
相关推荐









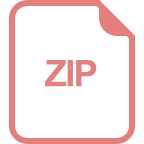