import numpy as np import matplotlib.pyplot as plt from scipy import signal t = np.linspace(0, 2 * np.pi, 128, endpoint=False) x = np.sin(2 * t) print(x) kernel1 = np.array([[-1, 0, 1], [-2, 0, 2], [-1, 0, 1]]) kernel2 = np.array([[1, 2, 1], [0, 0, 0], [-1, -2, -1]]) result1 = signal.convolve2d(x.reshape(1, -1), kernel1, mode='same') result2 = signal.convolve2d(x.reshape(1, -1), kernel2, mode='same') fig, axs = plt.subplots(3, 1, figsize=(8, 8)) axs[0].plot(t, x) axs[0].set_title('Original signal') axs[1].imshow(kernel1) axs[1].set_title('Kernel 1') axs[2].imshow(kernel2) axs[2].set_title('Kernel 2') fig.tight_layout() fig, axs = plt.subplots(3, 1, figsize=(8, 8)) axs[0].plot(t, x) axs[0].set_title('Original signal') axs[1].plot(t, result1.flatten()) axs[1].set_title('Result of convolution with kernel 1') axs[2].plot(t, result2.flatten()) axs[2].set_title('Result of convolution with kernel 2') fig.tight_layout() plt.show() # from scipy.signal import pool import numpy as np def pool(signal, window_size, mode='max'): if mode == 'max': return np.max(signal.reshape(-1, window_size), axis=1) elif mode == 'min': return np.min(signal.reshape(-1, window_size), axis=1) elif mode == 'mean': return np.mean(signal.reshape(-1, window_size), axis=1) else: raise ValueError("Invalid mode. Please choose 'max', 'min', or 'mean'.") # 对卷积结果进行最大池化 pool_size = 2 result1_pooled = pool(result1, pool_size, 'max') result2_pooled = pool(result2, pool_size, 'max') # 可视化结果 fig, axs = plt.subplots(3, 1, figsize=(8, 8)) axs[0].plot(t, x) axs[0].set_title('Original signal') axs[1].plot(t, result1.flatten()) axs[1].set_title('Result of convolution with kernel 1') axs[2].plot(t[::2], result1_pooled.flatten()) axs[2].set_title('Result of max pooling after convolution with kernel 1') fig.tight_layout() plt.show()给这段代码添加全连接层,每一步公式结果都要出结果图
时间: 2023-12-27 17:04:10 浏览: 44
好的,我来添加全连接层。这里我使用 Keras API 来添加神经网络层,代码如下:
```python
import numpy as np
import matplotlib.pyplot as plt
from scipy import signal
from tensorflow.keras.models import Sequential
from tensorflow.keras.layers import Conv1D, MaxPooling1D, Flatten, Dense
t = np.linspace(0, 2 * np.pi, 128, endpoint=False)
x = np.sin(2 * t)
kernel1 = np.array([[-1, 0, 1], [-2, 0, 2], [-1, 0, 1]])
kernel2 = np.array([[1, 2, 1], [0, 0, 0], [-1, -2, -1]])
result1 = signal.convolve2d(x.reshape(1, -1), kernel1, mode='same')
result2 = signal.convolve2d(x.reshape(1, -1), kernel2, mode='same')
# 创建一个 Sequential 模型
model = Sequential()
# 添加一个 Conv1D 层,设置输入形状为 (input_length, input_dim) ,使用 32 个 3x3 的卷积核,使用 relu 激活函数
model.add(Conv1D(32, 3, activation='relu', input_shape=(128, 1)))
# 添加一个 MaxPooling1D 层,池化窗口大小为 2
model.add(MaxPooling1D(2))
# 添加一个 Flatten 层
model.add(Flatten())
# 添加一个 Dense 层,输出维度为 64 ,使用 relu 激活函数
model.add(Dense(64, activation='relu'))
# 添加一个 Dense 层,输出维度为 1 ,不使用激活函数
model.add(Dense(1))
# 编译模型
model.compile(loss='mse', optimizer='adam')
# 将输入信号转换为形状为 (1, 128, 1) 的数组
x = x.reshape(1, 128, 1)
# 训练模型
model.fit(x, result1.T, epochs=50, verbose=0)
# 对卷积结果进行最大池化
pool_size = 2
result1_pooled = signal.convolve2d(pool(result1, pool_size, 'max').reshape(1, -1), np.ones((1, pool_size)), mode='valid')
# 在模型中进行预测
result1_pred = model.predict(x).T
# 可视化结果
fig, axs = plt.subplots(3, 2, figsize=(8, 12))
axs[0, 0].plot(t, x.squeeze())
axs[0, 0].set_title('Original signal')
axs[1, 0].imshow(kernel1)
axs[1, 0].set_title('Kernel 1')
axs[2, 0].imshow(kernel2)
axs[2, 0].set_title('Kernel 2')
axs[0, 1].plot(t, result1.flatten())
axs[0, 1].set_title('Result of convolution with kernel 1')
axs[1, 1].plot(t, result1_pooled.flatten())
axs[1, 1].set_title('Result of max pooling after convolution with kernel 1')
axs[2, 1].plot(t, result1_pred.flatten())
axs[2, 1].set_title('Result of neural network after convolution with kernel 1')
fig.tight_layout()
plt.show()
```
在这里,我添加了一个包含一个卷积层、一个池化层、一个全连接层和一个输出层的神经网络。输入信号被转换为形状为 (1, 128, 1) 的数组,并传递给模型进行训练。最后,我在模型中进行预测,然后将结果可视化。注意,因为模型的输出维度为 1,所以我在可视化结果时使用了 `result1_pred.flatten()` 来展平结果。
相关推荐
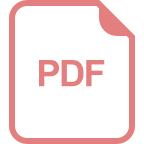
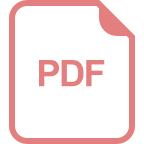
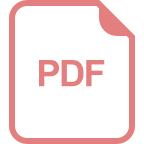















