unet模型特征提取模块
时间: 2024-11-29 08:15:33 浏览: 16
Unet是一种深度学习模型,特别适用于图像分割任务,其名称来源于它的网络结构,类似于字母U形。在Unet中,特征提取模块是非常关键的部分,它通常包括几个主要步骤:
1. **输入层**:接受原始的图像数据作为输入。
2. **卷积层**:使用一组卷积核对输入进行多次卷积操作,这一步用于从原始像素中提取低级特征,如边缘、纹理等。
3. **下采样(编码路径)**:通过池化层(如最大池化)逐渐减小空间分辨率并增加特征的抽象程度,同时捕获更大的上下文信息。
4. **瓶颈层**:在下采样的过程中,有时候会包含一个全连接层,这是为了保留更多的细节特征。
5. **上采样(解码路径)**:使用反卷积层(也称作转置卷积或上采样卷积),逐步恢复原始尺寸,并结合之前编码阶段捕获的信息,以提高精度。
6. **融合**:在解码路径的每一层,都会与对应编码层位置的特征图进行空间上或通道上的合并,这有助于结合不同尺度的特征。
7. **输出层**:最后一般是一个1x1的卷积层,输出与输入图像同样大小的预测结果,每个像素代表类别概率。
Unet的一个显著特点是它能够在同一张图片的粗细粒度之间自由穿梭,既能捕捉到局部的细节,又能保持全局的视野。
相关问题
Unet模型pytorch
可以使用PyTorch实现Unet模型。Unet是一种常用的图像分割模型,它具有编码器-解码器结构和跳跃连接,可以有效地提取图像特征并进行像素级别的分割。在PyTorch中,可以使用nn.Module类来定义Unet模型,同时使用nn.Conv2d、nn.MaxPool2d、nn.ConvTranspose2d等模块来构建编码器和解码器。
UNet经典模型实战
UNet是一种经典的语义分割模型,其结构简单而有效。以下是一个使用PyTorch实现UNet模型的示例代码:
```python
import torch
import torch.nn as nn
class DoubleConv(nn.Module):
def __init__(self, in_channels, out_channels):
super().__init__()
self.conv = nn.Sequential(
nn.Conv2d(in_channels, out_channels, kernel_size=3, padding=1),
nn.BatchNorm2d(out_channels),
nn.ReLU(inplace=True),
nn.Conv2d(out_channels, out_channels, kernel_size=3, padding=1),
nn.BatchNorm2d(out_channels),
nn.ReLU(inplace=True)
)
def forward(self, x):
return self.conv(x)
class UNet(nn.Module):
def __init__(self, in_channels=3, out_channels=1, features=[64, 128, 256, 512]):
super().__init__()
self.ups = nn.ModuleList()
self.downs = nn.ModuleList()
self.pool = nn.MaxPool2d(kernel_size=2, stride=2)
# Down part of UNet
for feature in features:
self.downs.append(DoubleConv(in_channels, feature))
in_channels = feature
# Up part of UNet
for feature in reversed(features):
self.ups.append(nn.ConvTranspose2d(feature*2, feature, kernel_size=2, stride=2))
self.ups.append(DoubleConv(feature*2, feature))
self.bottleneck = DoubleConv(features[-1], features[-1]*2)
self.final_conv = nn.Conv2d(features[0], out_channels, kernel_size=1)
def forward(self, x):
skip_connections = []
# Down part of UNet
for down in self.downs:
x = down(x)
skip_connections.append(x)
x = self.pool(x)
x = self.bottleneck(x)
skip_connections = skip_connections[::-1]
# Up part of UNet
for idx in range(0, len(self.ups), 2):
x = self.ups[idx](x)
skip_connection = skip_connections[idx//2]
if x.shape != skip_connection.shape:
x = nn.functional.interpolate(x, size=skip_connection.shape[2:], mode='bilinear', align_corners=True)
concat_skip = torch.cat((skip_connection, x), dim=1)
x = self.ups[idx+1](concat_skip)
return self.final_conv(x)
```
上述代码中,DoubleConv是UNet中使用的基本模块,包含两个3x3卷积层和一个ReLU激活函数。UNet类中,首先定义了一个Down part,其中包含多个DoubleConv模块和一个MaxPool2d层,用于从输入图像中提取特征。然后定义了一个Bottleneck模块,用于从提取的特征中进一步提取信息。最后定义了一个Up part,其中包含多个ConvTranspose2d层和多个DoubleConv模块,用于将特征图上采样并与Down part中的对应特征图拼接,最终输出分割结果。
使用该模型进行训练时,可以使用像Dice Loss或Cross-Entropy Loss等常见的分割任务损失函数,同时可以使用像Adam或SGD等常见的优化器进行模型优化。
阅读全文
相关推荐
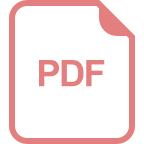
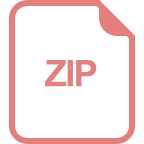
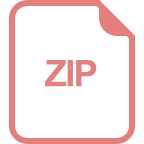
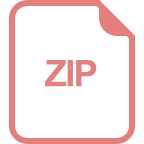
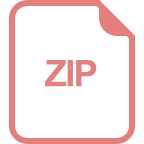
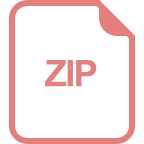
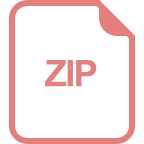
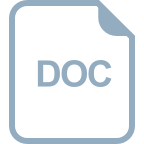
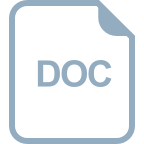
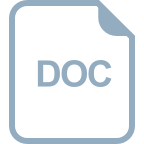
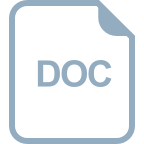
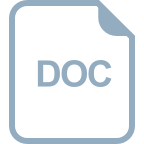
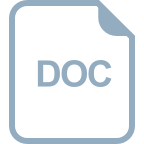
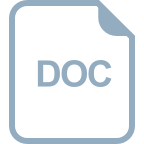
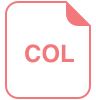

