文中代码运行出错,代码有问题跑不了,怎么改
时间: 2024-12-20 13:28:36 浏览: 4
文中代码在调用 `pso` 函数时遇到了错误。具体问题是 `objective_function` 返回了一个列表 `[total_cost, total_carbon]`,而 `pso` 函数期望的是一个标量值。我们需要将目标函数改为返回单个值,同时调整优化目标以平衡成本和碳排放。
以下是修改后的代码:
```python
import numpy as np
import pandas as pd
from pyswarm import pso
from math import radians, sin, cos, sqrt, atan2
# 计算两点之间的距离(Haversine公式)
def haversine(lat1, lon1, lat2, lon2):
R = 6371 # 地球半径,单位为公里
lat1, lon1, lat2, lon2 = map(radians, [lat1, lon1, lat2, lon2])
dlat = lat2 - lon1
a = sin(dlat / 2) ** 2 + cos(lat1) * cos(lat2) * sin(dlon / 2) ** 2
c = 2 * atan2(sqrt(a), sqrt(1 - a))
distance = R * c
return distance
# 读取数据
waste_data = {
"name": ["石桥镇", "百节镇", "万家镇", "南岳镇", "大树镇", "景市镇", "平滩镇", "赵家镇", "双庙镇", "渡市镇", "罐子镇", "管村镇", "大堰镇", "赵固镇", "石梯镇", "桥湾镇", "堡子镇"],
"latitude": [31.298319, 31.023069, 30.956475, 31.215063, 30.903638, 31.012362, 30.933431, 30.962931, 30.984120, 31.059865, 31.135825, 31.182932, 31.236690, 31.240285, 31.228216, 31.261140, 31.344459],
"longitude": [107.118101, 107.454102, 107.623465, 107.502044, 107.633019, 107.552492, 107.512259, 107.416361, 107.367131, 107.277676, 107.301602, 107.311350, 107.332680, 107.280691, 107.160852, 107.202620, 107.303139],
"amount": [2.86, 0.87, 3.71, 1.52, 3.39, 0.97, 1.55, 1.24, 1.61, 1.62, 1.81, 2.35, 0.93, 0.75, 3.64, 3.18, 1.68]
}
recycling_center_data = {
"name": ["分类回收节点 1", "分类回收节点 2", "分类回收节点 3", "分类回收节点 4", "分类回收节点 5", "分类回收节点 6", "分类回收节点 7", "分类回收节点 8", "分类回收节点 9", "分类回收节点 10"],
"latitude": [31.469126, 31.374130, 31.182932, 31.023069, 31.559861, 31.415139, 31.355846, 31.477050, 31.084636, 31.030836],
"longitude": [107.381715, 107.520675, 107.311350, 107.454102, 107.662229, 107.792071, 107.934038, 107.862990, 107.865317, 107.950456],
"fixed_cost": [150000, 160000, 155000, 160000, 156000, 170000, 165000, 175000, 180000, 160000],
"variable_cost": [65, 60, 62, 65, 70, 68, 65, 68, 78, 60],
"carbon_emission": [7.4, 6.8, 6.5, 6.6, 7.1, 6.5, 6.8, 7.2, 7.9, 6.1],
"recovery_rate": [0.87, 0.88, 0.85, 0.84, 0.88, 0.82, 0.83, 0.84, 0.82, 0.86]
}
manufacturing_center_data = {
"name": ["再制造中心 1", "再制造中心 2", "再制造中心 3", "再制造中心 4", "再制造中心 5", "再制造中心 6", "再制造中心 7", "再制造中心 8", "再制造中心 9", "再制造中心 10"],
"latitude": [30.759173, 30.881567, 31.166667, 31.511537, 31.189239, 31.583331, 31.773757, 31.88538, 32.111504, 31.705033],
"longitude": [107.095849, 107.393755, 107.293659, 107.561008, 107.954275, 108.033926, 107.750011, 107.93333, 108.045688, 108.206486],
"fixed_cost": [300000, 305000, 310000, 320000, 315000, 330000, 320000, 310000, 325000, 330000],
"variable_cost": [200, 210, 205, 210, 215, 220, 215, 225, 230, 210],
"carbon_emission": [102, 108, 98, 96, 102, 103, 106, 110, 98, 104],
"recovery_rate": [0.87, 0.86, 0.88, 0.85, 0.89, 0.83, 0.85, 0.84, 0.86, 0.87]
}
landfill_data = {
"name": ["填埋场 1", "填埋场 4", "填埋场 7"],
"latitude": [31.3623822568, 31.583337, 30.741412],
"longitude": [107.063886246, 107.92318, 107.364349],
"variable_cost": [54, 55, 58],
"carbon_emission": [6.23, 6.21, 6.32]
}
# 将数据转换为 DataFrame
df_waste = pd.DataFrame(waste_data)
df_recycling_centers = pd.DataFrame(recycling_center_data)
df_manufacturing_centers = pd.DataFrame(manufacturing_center_data)
df_landfills = pd.DataFrame(landfill_data)
# 计算所有节点之间的距离矩阵
dist_matrix = np.zeros((len(df_waste) + len(df_recycling_centers) + len(df_manufacturing_centers) + len(df_landfills),
len(df_waste) + len(df_recycling_centers) + len(df_manufacturing_centers) + len(df_landfills)))
for i in range(len(df_waste)):
for j in range(i + 1, len(df_waste)):
dist_matrix[i, j] = haversine(df_waste.iloc[i]['latitude'], df_waste.iloc[i]['longitude'],
df_waste.iloc[j]['latitude'], df_waste.iloc[j]['longitude'])
dist_matrix[j, i] = dist_matrix[i, j]
for i in range(len(df_waste)):
for j in range(len(df_waste), len(df_waste) + len(df_recycling_centers)):
dist_matrix[i, j] = haversine(df_waste.iloc[i]['latitude'], df_waste.iloc[i]['longitude'],
df_recycling_centers.iloc[j - len(df_waste)]['latitude'],
df_recycling_centers.iloc[j - len(df_waste)]['longitude'])
for i in range(len(df_waste)):
for j in range(len(df_waste) + len(df_recycling_centers),
len(df_waste) + len(df_recycling_centers) + len(df_manufacturing_centers)):
dist_matrix[i, j] = haversine(df_waste.iloc[i]['latitude'], df_waste.iloc[i]['longitude'],
df_manufacturing_centers.iloc[j - (len(df_waste) + len(df_recycling_centers))][
'latitude'],
df_manufacturing_centers.iloc[j - (len(df_waste) + len(df_recycling_centers))][
'longitude'])
for i in range(len(df_waste)):
for j in range(len(df_waste) + len(df_recycling_centers) + len(df_manufacturing_centers),
len(df_waste) + len(df_recycling_centers) + len(df_manufacturing_centers) + len(df_landfills)):
dist_matrix[i, j] = haversine(df_waste.iloc[i]['latitude'], df_waste.iloc[i]['longitude'],
df_landfills.iloc[j - (len(df_waste) + len(df_recycling_centers) +
len(df_manufacturing_centers))]['latitude'],
df_landfills.iloc[j - (len(df_waste) + len(df_recycling_centers) +
len(df_manufacturing_centers))]['longitude'])
# 定义目标函数
def objective_function(x):
n_waste_nodes = len(df_waste)
n_recycling_nodes = len(df_recycling_centers)
n_manufacturing_nodes = len(df_manufacturing_centers)
n_landfill_nodes = len(df_landfills)
recycling_nodes = x[:n_recycling_nodes].astype(int)
manufacturing_nodes = x[n_recycling_nodes:n_recycling_nodes + n_manufacturing_nodes].astype(int)
total_cost = 0
total_carbon = 0
for i in range(n_waste_nodes):
waste_amount = df_waste.iloc[i]['amount']
if sum(recycling_nodes) > 0:
nearest_recycling_node = np.argmin([dist_matrix[i, j] for j in range(n_waste_nodes,
n_waste_nodes + n_recycling_nodes) if
recycling_nodes[j - n_waste_nodes]])
recycling_node_index = nearest_recycling_node + n_waste_nodes
recycling_fixed_cost = df_recycling_centers.iloc[nearest_recycling_node]['fixed_cost']
recycling_variable_cost = df_recycling_centers.iloc[nearest_recycling_node]['variable_cost']
recycling_carbon_emission = df_recycling_centers.iloc[nearest_recycling_node]['carbon_emission']
transport_distance_to_recycling = dist_matrix[i, recycling_node_index]
transport_cost_to_recycling = transport_distance_to_recycling * waste_amount * 5.5
transport_carbon_to_recycling = transport_distance_to_recycling * waste_amount * 0.35
total_cost += recycling_fixed_cost + recycling_variable_cost * waste_amount + transport_cost_to_recycling
total_carbon += recycling_carbon_emission * waste_amount + transport_carbon_to_recycling
if sum(manufacturing_nodes) > 0:
nearest_manufacturing_node = np.argmin(
[dist_matrix[recycling_node_index, j] for j in range(n_waste_nodes + n_recycling_nodes,
n_waste_nodes + n_recycling_nodes +
n_manufacturing_nodes) if
manufacturing_nodes[j - (n_waste_nodes + n_recycling_nodes)]])
manufacturing_node_index = nearest_manufacturing_node + n_waste_nodes + n_recycling_nodes
manufacturing_fixed_cost = df_manufacturing_centers.iloc[nearest_manufacturing_node]['fixed_cost']
manufacturing_variable_cost = df_manufacturing_centers.iloc[nearest_manufacturing_node]['variable_cost']
manufacturing_carbon_emission = df_manufacturing_centers.iloc[nearest_manufacturing_node]['carbon_emission']
transport_distance_to_manufacturing = dist_matrix[recycling_node_index, manufacturing_node_index]
transport_cost_to_manufacturing = transport_distance_to_manufacturing * waste_amount * 5.5
transport_carbon_to_manufacturing = transport_distance_to_manufacturing * waste_amount * 0.35
total_cost += manufacturing_fixed_cost + manufacturing_variable_cost * waste_amount + transport_cost_to_manufacturing
total_carbon += manufacturing_carbon_emission * waste_amount + transport_carbon_to_manufacturing
else:
nearest_landfill_node = np.argmin(
[dist_matrix[recycling_node_index, j] for j in range(n_waste_nodes + n_recycling_nodes +
n_manufacturing_nodes,
n_waste_nodes + n_recycling_nodes +
n_manufacturing_nodes + n_landfill_nodes)])
landfill_node_index = nearest_landfill_node + n_waste_nodes + n_recycling_nodes + n_manufacturing_nodes
landfill_variable_cost = df_landfills.iloc[nearest_landfill_node]['variable_cost']
landfill_carbon_emission = df_landfills.iloc[nearest_landfill_node]['carbon_emission']
transport_distance_to_landfill = dist_matrix[recycling_node_index, landfill_node_index]
transport_cost_to_landfill = transport_distance_to_landfill * waste_amount * 5.5
transport_carbon_to_landfill = transport_distance_to_landfill * waste_amount * 0.35
total_cost += landfill_variable_cost * waste_amount + transport_cost_to_landfill
total_carbon += landfill_carbon_emission * waste_amount + transport_carbon_to_landfill
else:
nearest_landfill_node = np.argmin(
[dist_matrix[i, j] for j in range(n_waste_nodes + n_recycling_nodes + n_manufacturing_nodes,
n_waste_nodes + n_recycling_nodes + n_manufacturing_nodes +
n_landfill_nodes)])
landfill_node_index = nearest_landfill_node + n_waste_nodes + n_recycling_nodes + n_manufacturing_nodes
landfill_variable_cost = df_landfills.iloc[nearest_landfill_node]['variable_cost']
landfill_carbon_emission = df_landfills.iloc[nearest_landfill_node]['carbon_emission']
transport_distance_to_landfill = dist_matrix[i, landfill_node_index]
transport_cost_to_landfill = transport_distance_to_landfill * waste_amount * 5.5
transport_carbon_to_landfill = transport_distance_to_landfill * waste_amount * 0.35
total_cost += landfill_variable_cost * waste_amount + transport_cost_to_landfill
total_carbon += landfill_carbon_emission * waste_amount + transport_carbon_to_landfill
# 平衡总成本和总碳排放
weighted_objective = total_cost + 0.1 * total_carbon
return weighted_objective
# 定义边界
lb = [0] * (len(df_recycling_centers) + len(df_manufacturing_centers))
ub = [1] * (len(df_recycling centers) + len(df_manufacturing_centers))
# 运行 PSO
opt = pso(objective_function, lb, ub, swarmsize=100, maxiter=100, minstep=1e-8, minfunc=1e-8)
print("Optimal solution found:")
print(f"Total cost: {opt[0]}")
print(f"Recycling nodes selected: {[int(round(x)) for x in opt[1][:len(df_recycling_centers)]]}")
print(f"Manufacturing nodes selected: {[int(round(x))
阅读全文
相关推荐
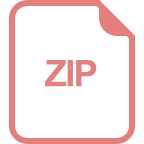
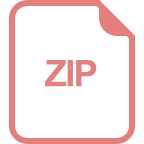
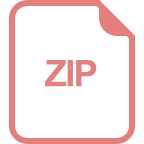
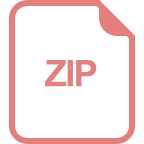
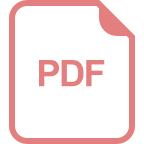
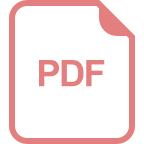
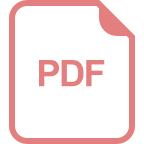
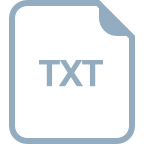
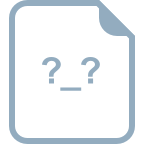
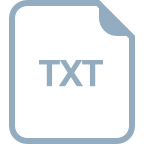
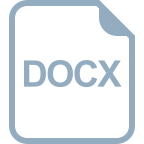
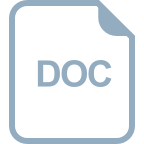
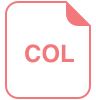
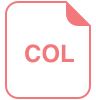
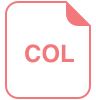
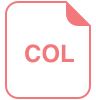
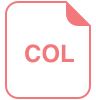
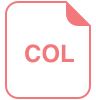
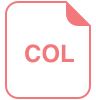