Unable to get the primary key of the biz_aidperson table, please try to decorate it with the annotation @AutoId(primaryKey = "id")on the primary key ?
时间: 2024-04-21 17:22:58 浏览: 11
To get the primary key of the `biz_aidperson` table, you can decorate the primary key field with the `@AutoId` annotation and specify the primary key column name using the `primaryKey` parameter. Here's an example:
```java
import com.xxx.annotation.AutoId;
public class BizAidPerson {
@AutoId(primaryKey = "id")
private Long id;
// Other fields and methods
}
```
By adding the `@AutoId(primaryKey = "id")` annotation to the `id` field, you are indicating that this field is the primary key for the `biz_aidperson` table, and the column name for the primary key is "id". Make sure to replace "id" with the actual column name of your primary key in the table.
相关问题
WARNING:tensorflow:AutoGraph could not transform <function Model.make_test_function.<locals>.test_function at 0x0000018CEA87BEE8> and will run it as-is. Please report this to the TensorFlow team. When filing the bug, set the verbosity to 10 (on Linux, `export AUTOGRAPH_VERBOSITY=10`) and attach the full output. Cause: 'arguments' object has no attribute 'posonlyargs' To silence this warning, decorate the function with @tf.autograph.experimental.do_not_convert
这是一条 TensorFlow 的警告信息,通常是因为 TensorFlow 无法将某个函数转换为 TensorFlow 的计算图形式。如果这个警告信息不影响你的程序运行,你可以忽略它。如果这个警告信息影响了程序的运行,你可以尝试使用 `@tf.autograph.experimental.do_not_convert` 装饰器来禁止 TensorFlow 将这个函数转换为计算图形式。例如:
```python
import tensorflow as tf
@tf.autograph.experimental.do_not_convert
def test_function():
# your code here
```
另外,如果你想报告这个警告信息给 TensorFlow 团队,可以按照警告信息中的提示设置 `AUTOGRAPH_VERBOSITY` 环境变量,并将完整的输出附上。例如:
```bash
export AUTOGRAPH_VERBOSITY=10
python your_code.py
```
RuntimeError: params_0: Attempting to capture an EagerTensor without building a function.
This error occurs when trying to use an EagerTensor object without building a TensorFlow function first.
To fix this error, you should build a TensorFlow function that includes the EagerTensor object. This can be done using the `tf.function` decorator, which converts a Python function into a TensorFlow graph function.
For example:
```
import tensorflow as tf
x = tf.constant([1, 2, 3], dtype=tf.float32)
y = tf.constant([4, 5, 6], dtype=tf.float32)
@tf.function
def my_func(params_0):
return params_0 * x + y
z = my_func(tf.constant([0.5, 0.6, 0.7], dtype=tf.float32))
```
In this example, we define a function `my_func` that takes a parameter `params_0` and returns the result of multiplying it with `x` and adding `y`. We decorate `my_func` with `tf.function` to convert it into a TensorFlow graph function.
We then call `my_func` with an EagerTensor `tf.constant([0.5, 0.6, 0.7], dtype=tf.float32)` to get the result `z`.
By building a TensorFlow function using `tf.function`, we ensure that TensorFlow can properly capture the EagerTensor object and execute it within the graph.
相关推荐
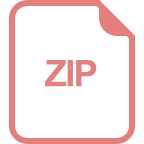
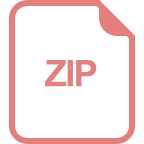
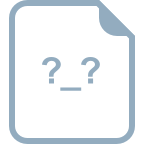













