def forward(self, input_question, input_answer): question_embed = self.embedding(input_question) answer_embed = self.embedding(input_answer) _, question_hidden = self.encoder(question_embed) answer_outputs, _ = self.encoder(answer_embed, question_hidden) attention_weights = self.attention(answer_outputs).squeeze(dim=-1) attention_weights = torch.softmax(attention_weights, dim=1) context_vector = torch.bmm(attention_weights.unsqueeze(dim=1), answer_outputs).squeeze(dim=1) logits = self.decoder(context_vector) top_100_values, _ = torch.topk(logits, self.topk, dim=1) mask = torch.zeros_like(logits, requires_grad=True) # 设置 requires_grad=True score = [] for i in range(logits.size(0)): top_100_indices = torch.argsort(logits[i])[-self.topk:] mask_i = mask[i].clone() # 创建副本 mask_i[top_100_indices] = 1.0 score.append(mask_i.clone()) # 创建副本并赋值回 mask score = torch.stack(score) return score 损失没有任何改变
时间: 2024-04-13 14:29:57 浏览: 22
根据你提供的代码,我注意到你尝试通过将 `score` 列表转换为张量来改变损失。然而,从你的描述中,我理解你想要改变的是 `mask` 张量,而不是 `score`。
在你的代码中,你创建了一个全零的 `mask` 张量,并在循环中根据 `top_100_indices` 更新了一部分元素为 1.0。然后,你将每个更新后的 `mask_i` 副本添加到 `score` 列表中。
如果你想改变 `mask` 张量而不是 `score` 列表,你可以直接使用列表推导式来构建修改后的 `mask` 张量。以下是修改后的代码示例:
```python
mask = torch.zeros_like(logits, requires_grad=True) # 设置 requires_grad=True
score = [torch.where(torch.argsort(logits[i])[-self.topk:] == j, torch.tensor(1.0), mask[i, j]).clone()
for i in range(logits.size(0))
for j in range(logits.size(1))]
score = torch.stack(score)
return score
```
在这个例子中,我们使用列表推导式来构建修改后的 `mask` 张量。对于每个样本和每个位置,我们使用 `torch.where()` 函数根据 `top_100_indices` 来判断是否将元素设置为 1.0 或保持原来的值,并创建一个包含所有修改后的元素的列表。最后,我们将这个列表转换为张量并返回。
希望这次能够解决问题!如果还有其他问题,请随时提问。
相关推荐
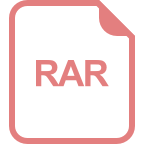






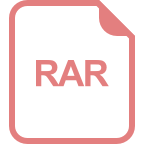
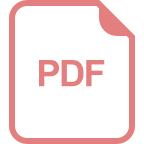
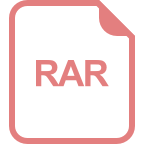
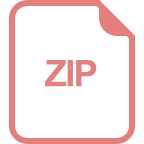
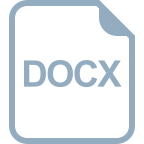
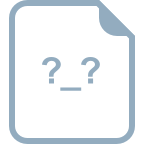