文本聚类算法原理与实践
发布时间: 2023-12-23 15:11:27 阅读量: 74 订阅数: 22 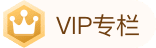
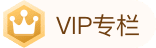
# 第一章:文本聚类概述
1.1 文本聚类概念
1.2 文本聚类应用场景
1.3 文本聚类在信息检索领域的重要性
## 第二章:文本聚类算法基础
文本聚类算法基础主要包括了基于距离的文本聚类算法、基于密度的文本聚类算法、基于概率的文本聚类算法以及常见的文本聚类算法对比分析。在这一章节中,我们将介绍文本聚类算法的基本原理及其在实际场景中的应用。
### 第三章:文本表示与特征选择
在文本聚类中,文本的表示和特征选择是至关重要的步骤。本章将介绍文本表示与特征选择的基本概念以及常用方法。
#### 3.1 文本预处理与特征抽取
文本预处理是指对原始文本数据进行清洗和处理,包括但不限于去除停用词、词干提取、去除特殊字符、转换为小写等步骤。特征抽取是将文本数据转换为计算机可以理解的特征表示,常见的特征抽取方法包括词袋模型、N-gram模型等。
```python
# Python示例:使用nltk进行文本预处理和特征抽取
import nltk
from nltk.corpus import stopwords
from nltk.tokenize import word_tokenize
from nltk.stem import PorterStemmer
from sklearn.feature_extraction.text import CountVectorizer
# 示例文本数据
text_data = ["This is an example sentence.", "Another example of a sentence."]
# 去除停用词和进行词干提取
nltk.download('punkt')
nltk.download('stopwords')
stop_words = set(stopwords.words('english'))
ps = PorterStemmer()
preprocessed_data = []
for sentence in text_data:
word_tokens = word_tokenize(sentence)
filtered_sentence = [ps.stem(w) for w in word_tokens if not w in stop_words]
preprocessed_data.append(' '.join(filtered_sentence))
# 使用词袋模型进行特征抽取
vectorizer = CountVectorizer()
X = vectorizer.fit_transform(preprocessed_data)
print(vectorizer.get_feature_names())
print(X.toarray())
```
#### 3.2 词袋模型与TF-IDF权重计算
词袋模型是文本特征抽取的一种方法,它将文本表示为词汇的存在与否,忽略其在文本中的顺序。TF-IDF(Term Frequency-Inverse Document Frequency)是词袋模型的一种改进,它考虑了词频和逆文档频率,用于评估一个词对于一个文档集或语料库中的一份文件的重要程度。
```java
// Java示例:使用Lucene进行词袋模型与TF-IDF权重计算
import org.apache.lucene.analysis.standard.StandardAnalyzer;
import org.apache.lucene.index.IndexWriter;
import org.apache.lucene.index.Term;
import org.apache.lucene.search.similarities.ClassicSimilarity;
import org.apache.lucene.store.Directory;
import org.apache.lucene.store.RAMDirectory;
import org.apache.lucene.util.BytesRef;
import org.apache.lucene.document.*;
// 示例文本数据
String[] textData = {"This is an example sentence.", "Another example of a sentence."};
// 使用词袋模型和TF-IDF权重计算
Directory index = new RAMDirectory();
IndexWriterConfig config = new IndexWriterConfig(new StandardAnalyzer());
IndexWriter writer = new IndexWriter(index, config);
for (String text : textData) {
Document doc = new Document();
doc.add(new TextField("content", text, Field.Store.YES));
writer.addDocument(doc);
}
writer.close();
// TF-IDF权重计算
Term term = new Term("content", "example");
IndexSearcher searcher = new IndexSearcher(DirectoryReader.open(index));
long totalTermFreq = searcher.collectionStatistics("content").sumTotalTermFreq();
long docCount = searcher.collectionStatistics("content").docCount();
ClassicSimilarity classicSimilarity = new ClassicSimilarity();
float tfidf = classicSimilarity.idf(docCount
```
0
0
相关推荐
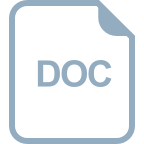
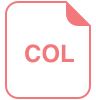
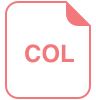
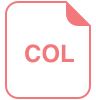
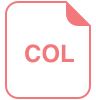
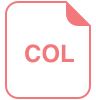