ARC GIS 数据查询与分析
发布时间: 2023-12-14 12:43:07 阅读量: 53 订阅数: 22 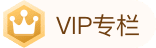
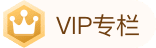
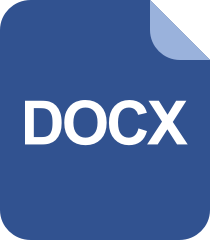
ArcGIS数据分析
## 一、概述ARC GIS数据查询与分析
### 1.1 什么是ARC GIS?
ARC GIS是一款由Esri公司开发的地理信息系统(Geographic Information System,简称GIS),它集数据采集、存储、管理、查询、分析、展示等功能于一体。ARC GIS以空间数据为核心,通过将地理位置信息与相关属性信息结合,可以帮助用户深入了解地理现象与空间关系,从而进行复杂的数据查询与分析。
### 1.2 数据查询与分析的重要性
数据查询与分析是ARC GIS的重要功能之一。在日常工作或研究中,我们经常需要从庞大的地理数据中提取出特定的信息,以便更好地理解和解决问题。而ARC GIS提供了多种查询和分析工具,帮助用户从海量数据中筛选、筛查和分析,进一步发现数据背后的规律与趋势,为决策提供有力支持。数据查询与分析的准确性和高效性对于各行业的决策者来说都至关重要,例如城市规划、环境保护、交通规划等。下面我们将介绍ARC GIS的基础知识,以及数据查询与分析的方法和应用。
## ARC GIS基础知识
ARC GIS是一个用于地理信息系统(GIS)的桌面应用程序,它包括一系列用于创建、编辑、分析和展示地图的工具。数据查询与分析是ARC GIS的核心功能之一,通过这些功能,用户可以轻松地从地理数据中提取信息并进行深入的分析。
### 2.1 ARC GIS软件介绍
ARC GIS由Esri公司开发,提供了多种产品版本,包括ARC GIS Pro和ARC GIS Online等。ARC GIS Pro是Esri最新推出的一款桌面应用程序,具有直观的界面和强大的数据处理能力;而ARC GIS Online则是基于云的GIS平台,可以实现在线地图制作和数据共享。
### 2.2 数据查询与分析工具概览
ARC GIS提供了丰富的数据查询与分析工具,包括属性查询工具、空间查询工具、地图代数工具、网络分析工具等。这些工具可以帮助用户快速地定位所需的地理数据,并进行多种复杂的空间分析操作。
### 三、数据查询方法
在ARC GIS中进行数据查询是非常重要的,它可以帮助我们从大量的数据中快速找到所需要的信息。ARC GIS提供了多种查询方法,包括属性查询、空间查询和查询表达式。
#### 3.1 属性查询
属性查询是根据要素的属性值进行查询。在ARC GIS中,可以使用SQL语句对属性进行查询,常用的操作符有等于(=)、不等于(<>)、大于(>)、小于(<)、大于等于(>=)、小于等于(<=)等。以下是一个简单的示例代码,演示如何进行属性查询:
```python
# 导入arcpy模块
import arcpy
# 设置工作空间
arcpy.env.workspace = r"C:\data"
# 设置查询的数据表
input_table = "cities.shp"
# 设置查询条件
query = "POPULATION > 5000000"
# 执行查询
arcpy.management.SelectLayerByAttribute(input_table, "NEW_SELECTION", query)
# 输出查询结果
result = arcpy.GetCount_management(input_table)
count = int(result.getOutput(0))
print(f"查询到的记录数为:{count}")
```
代码解析:
- 首先导入arcpy模块。
- 然后设置工作空间,即数据所在的文件夹。
- 接下来设置要查询的数据表,这里假设数据表为"cities.shp"。
- 然后设置查询条件,这里的查询条件是人口数量大于5000000。
- 使用`arcpy.management.SelectLayerByAttribute`方法执行查询,将符合条件的要素选中。
- 最后使用`arcpy.GetCount_management`方法获取查询结果的记录数,并进行输出。
#### 3.2 空间查询
空间查询是根据要素的空间位置进行查询。在ARC GIS中,可以进行基于距离、接触的空间查询,也可以进行相交、包含的空间查询。以下是一个简单的示例代码,演示如何进行空间查询:
```python
# 导入arcpy模块
import arcpy
# 设置工作空间
arcpy.env.workspace = r"C:\data"
# 设置查询的数据表
input_table = "states.shp"
# 设置查询的几何对象
geometry = arcpy.Geometry("POINT (118 36)", "SPATIALREFERENCE 4326")
# 设置查询类型
spatial_rel = "INTERSECT"
# 执行查询
arcpy.management.SelectLayerByLocation(input_table, spatial_rel, geometry)
# 输出查询结果
result = arcpy.GetCount_management(input_table)
count = int(result.getOutput(0))
print(f"查询到的记录数为:{count}")
```
代码解析:
- 首先导入arcpy模块。
- 然后设置工作空间,即数据所在的文件夹。
- 接下来设置要查询的数据表,这里假设数据表为"states.shp"。
- 然后使用`arcpy.Geometry`方法创建一个点几何对象,表示查询的位置。
- 设置查询类型,这里使用"INTERSECT"表示查询与给定几何对象相交的要素。
- 使用`arcpy.management.SelectLayerByLocation`方法执行查询,将符合条件的要素选中。
- 最后使用`arcpy.GetCount_management`方法获取查询结果的记录数,并进行输出。
#### 3.3 查询表达式
在ARC GIS中,查询表达式可以进一步细化查询条件。查询表达式是一种基于SQL的语法,可以用于属性查询和空间查询。以下是一个简单的示例代码,演示如何使用查询表达式进行查询:
```python
# 导入arcpy模块
import arcpy
# 设置工作空间
arcpy.env.workspace = r"C:\data"
# 设置查询的数据表
input_table = "roads.shp"
# 设置查询表达式
expression = "TYPE = 'Highway' AND LENGTH > 10"
# 执行查询
arcpy.management.SelectLayerByAttribute(input_table, "NEW_SELECTION", expression)
# 输出查询结果
result = arcpy.GetCount_management(input_table)
count = int(result.getOutput(0))
print(f"查询到的记录数为:{count}")
```
代码解析:
- 首先导入arcpy模块。
- 然后设置工作空间,即数据所在的文件夹。
- 接下来设置要查询的数据表,这里假设数据表为"roads.shp"。
- 然后设置查询表达式,这里的查询表达式是表示道路类型为"Highway"且长度大于10的要素。
- 使用`arcpy.management.SelectLayerByAttribute`方法执行查询,将符合条件的要素选中。
- 最后使用`arcpy.GetCount_management`方法获取查询结果的记录数,并进行输出。
四、数据分析方法
---
在ARC GIS中,数据分析是一个重要的功能,它可以帮助用户更好地理解和利用地理数据。下面将介绍三种常用的数据分析方法。
4.1 空间分析
---
空间分析是ARC GIS中最常用的一种分析方法,它可以帮助用户理解地理数据的空间关系,并从中提取有用的信息。常见的空间分析方法包括:
- 缓冲区分析:通过在目标要素周围创建缓冲区来分析空间关系,比如确定一定距离内的其他要素。
```python
# 创建缓冲区
arcpy.Buffer_analysis("input.shp", "output.shp", "500 Meters")
```
- 叠加分析:将多个要素图层进行叠加,分析它们之间的空间关系并生成新的图层。
```python
# 叠加分析
arcpy.Intersect_analysis(["input1.shp", "input2.shp"], "output.shp")
```
- 空间插值:通过已有的点数据生成连续的表面,用于分析地理现象的分布规律。
```python
# 空间插值
arcpy.Idw_3d("input.shp", "value_field", "output.tif")
```
4.2 地图代数
---
地图代数是一种基于地图代数运算的数据分析方法,它可以通过对栅格数据进行代数运算,生成新的栅格图层。常见的地图代数运算包括:
- 加法运算:将两个栅格图层叠加起来,生成新的栅格图层。
```python
# 加法运算
arcpy.Plus_3d("input1.tif", "input2.tif", "output.tif")
```
- 减法运算:将一个栅格图层减去另一个栅格图层,生成新的栅格图层。
```python
# 减法运算
arcpy.Minus_3d("input1.tif", "input2.tif", "output.tif")
```
- 乘法运算:将两个栅格图层相乘,生成新的栅格图层。
```python
# 乘法运算
arcpy.Times_3d("input1.tif", "input2.tif", "output.tif")
```
4.3 网络分析
---
网络分析是一种针对网络数据进行分析的方法,它可以帮助用户确定最佳路径、计算网络距离等。常见的网络分析包括:
- 最短路径分析:根据网络数据,确定两个点之间的最短路径。
```python
# 最短路径分析
arcpy.na.ShortestPath("network.lyr", "input.shp", "output.shp")
```
- 服务区分析:确定以特定点为中心,一定时间或距离范围内的可达区域。
```python
# 服务区分析
arcpy.na.ServiceArea("network.lyr", "input.shp", "output.shp")
```
- 障碍点分析:通过在网络上设置障碍点,分析路径的可行性。
```python
# 障碍点分析
arcpy.na.AddLocations("network.lyr", "barriers.shp", "input.shp")
```
### 五、实际案例
#### 5.1 基于ARC GIS的城市规划分析
在城市规划中,ARC GIS的数据查询与分析功能可以帮助规划师对城市地理数据进行深入分析,从而更好地制定规划方案。下面以一个城市人口密度分析为例进行说明。
首先,我们需要准备相关的数据。假设我们拥有一份包含城市边界、人口分布、交通等要素的地理数据。在ARC GIS中,可以将这些数据导入为要素类或栅格数据集。
```python
import arcpy
arcpy.env.workspace = "C:/path/to/workspace"
# 导入要素类或栅格数据集
arcpy.FeatureClassToGeodatabase_conversion("C:/path/to/input.shp", "C:/path/to/output.gdb")
# 设置分析环境
arcpy.env.extent = "C:/path/to/boundary.shp"
arcpy.env.cellSize = "30"
# 计算人口密度
arcpy.sa.ZonalStatistics("C:/path/to/boundary.shp", "POPULATION", "C:/path/to/population_density.tif", "SUM", "NODATA")
```
接着,我们可以利用ARC GIS提供的工具进行数据分析。例如,使用空间分析工具计算城市边界内的人口密度。下面是一个示例代码:
```python
import arcpy
arcpy.env.workspace = "C:/path/to/workspace"
# 设置分析环境
arcpy.env.extent = "C:/path/to/boundary.shp"
arcpy.env.cellSize = "30"
# 计算人口密度
arcpy.sa.ZonalStatistics("C:/path/to/boundary.shp", "POPULATION", "C:/path/to/population_density.tif", "SUM", "NODATA")
```
通过以上代码,我们利用`ZonalStatistics`函数对城市边界内的人口分布进行统计,并生成了人口密度图。可以通过该图像来分析城市中人口密度的分布情况,并作为规划师制定城市发展方案的参考依据。
#### 5.2 基于ARC GIS的环境保护分析
环境保护分析是另一个重要的应用领域。通过ARC GIS的数据查询与分析功能,我们可以对环境数据进行深入分析,评估环境质量、寻找污染源等。以下以水质分析为例进行说明。
假设我们拥有一份包含水体分布、污染源位置等要素的地理数据。在ARC GIS中,可以根据这些数据建立相应的要素类,并进行查询和分析。
```python
import arcpy
arcpy.env.workspace = "C:/path/to/workspace"
# 创建水体要素类
arcpy.CreateFeatureclass_management("C:/path/to/workspace", "water_bodies.shp", "POINT")
# 导入水体数据
arcpy.Append_management("C:/path/to/water_bodies.csv", "C:/path/to/workspace/water_bodies.shp")
# 创建污染源要素类
arcpy.CreateFeatureclass_management("C:/path/to/workspace", "pollution_sources.shp", "POINT")
# 导入污染源数据
arcpy.Append_management("C:/path/to/pollution_sources.csv", "C:/path/to/workspace/pollution_sources.shp")
# 进行空间查询
arcpy.SelectLayerByLocation_management("water_bodies.shp", "WITHIN_A_DISTANCE", "pollution_sources.shp", "100 Meters", "NEW_SELECTION")
# 导出查询结果
arcpy.CopyFeatures_management("water_bodies.shp", "C:/path/to/result.shp")
```
以上代码首先创建了水体和污染源的要素类,并导入相应的数据。然后利用空间查询工具`SelectLayerByLocation`选取距离污染源100米以内的水体要素,并将查询结果保存到`result.shp`文件中。
通过以上实例,我们可以利用ARC GIS进行环境保护分析,快速定位污染源,并评估其对周围水体的影响。这对环境保护工作具有重要意义。
以上是基于ARC GIS的城市规划分析和环境保护分析的简单示例,通过数据查询与分析工具的灵活运用,我们可以更好地理解城市和环境数据,并为相关决策提供科学依据。
注:以上代码仅为示例,实际使用时需根据具体数据和需求进行调整。
## 六、未来发展趋势
### 6.1 ARC GIS在大数据时代的应用展望
With the rapid development of big data technology, ARC GIS is facing new opportunities and challenges. The integration of ARC GIS with big data can bring about significant improvements in data processing, analysis, and visualization.
One of the main advantages of ARC GIS in the big data era is its ability to handle large volumes of data. Traditional GIS systems may struggle to process and analyze huge datasets, but ARC GIS has the potential to overcome these limitations. By leveraging distributed computing frameworks like Apache Hadoop or Apache Spark, ARC GIS can distribute the workload across multiple machines and efficiently process massive amounts of data.
Another area where ARC GIS can benefit from big data technology is in real-time data analysis. With the increasing availability of sensors, IoT devices, and social media data, there is a growing need for real-time analysis and visualization of geospatial data. ARC GIS can leverage streaming data processing frameworks like Apache Kafka or Apache Flink to analyze and visualize streaming data in real-time, enabling quick decision-making and response to dynamic situations.
Furthermore, the integration of ARC GIS with machine learning and artificial intelligence algorithms opens up new possibilities for advanced spatial analysis and predictive modeling. By applying machine learning algorithms to geospatial data, ARC GIS can uncover hidden patterns, detect anomalies, and make accurate predictions. For example, ARC GIS can be used to predict landslides, urban growth patterns, or disease outbreaks based on historical data and various environmental factors.
### 6.2 人工智能与ARC GIS的结合
The combination of artificial intelligence (AI) and ARC GIS holds immense potential for revolutionizing geospatial analysis and decision-making. AI technologies, such as computer vision, natural language processing, and deep learning, can enhance the capabilities of ARC GIS in various ways.
Computer vision algorithms can enable ARC GIS to automatically extract valuable insights from satellite imagery, aerial photos, and other visual data sources. For example, AI can be used to detect land cover changes, identify objects of interest, or classify vegetation types. This automation can significantly speed up the analysis process and reduce the manual effort required.
Natural language processing (NLP) techniques can also enhance ARC GIS by enabling users to interact with the system using natural language queries and commands. This simplifies the data querying and analysis process, making ARC GIS more accessible to a wider range of users. NLP algorithms can parse and understand user queries, extract relevant information, and provide accurate results or recommendations.
Deep learning algorithms, with their ability to learn from big data, can be used to develop models for solving complex geospatial problems. By training neural networks on large geospatial datasets, ARC GIS can create predictive models that can forecast environmental changes, assess infrastructure risks, or optimize resource allocation. These models can provide valuable insights for effective planning and decision-making.
In conclusion, the integration of AI technologies with ARC GIS opens up exciting possibilities for the future. It can enhance data processing, analysis, and visualization, enable real-time analysis of streaming geospatial data, and empower advanced spatial analysis and predictive modeling. The combined power of AI and ARC GIS holds great promise for addressing complex geospatial challenges and driving innovation in various domains.
0
0
相关推荐





