GraphSAGE节点分类在交通管理中的应用:改善交通拥堵与提高道路安全,畅通城市交通
发布时间: 2024-08-21 09:28:44 阅读量: 16 订阅数: 15 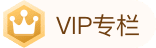
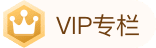
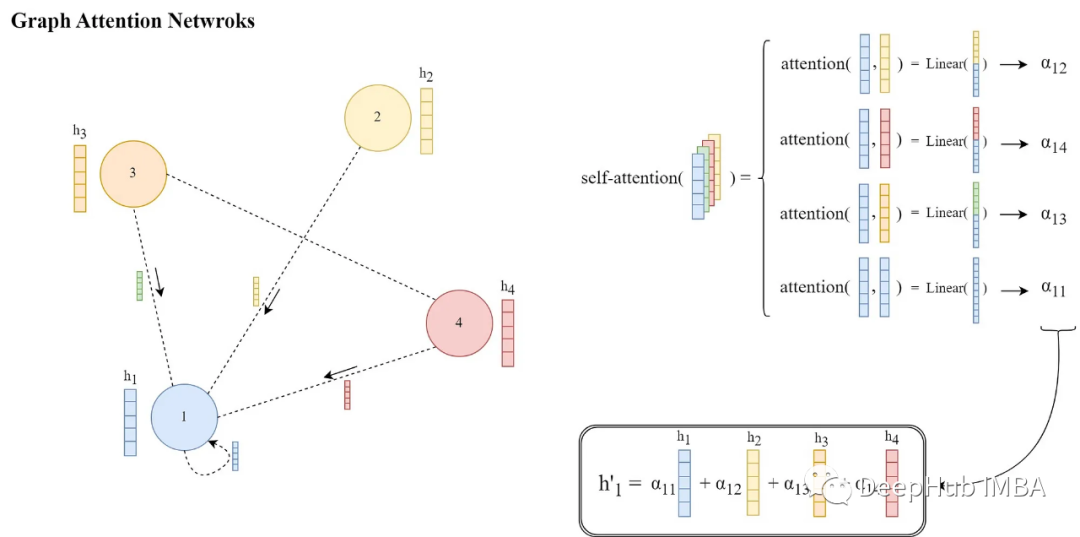
# 1. GraphSAGE节点分类概述**
GraphSAGE(Graph SAmple and aggreGatE)是一种用于节点分类的图神经网络算法。它通过对节点的邻域进行聚合,学习节点的特征表示。GraphSAGE算法具有采样机制,可以有效地处理大规模图数据。
# 2. GraphSAGE节点分类算法
### 2.1 GraphSAGE算法原理
GraphSAGE(Graph Sample and Aggregate)是一种用于节点分类的图神经网络算法。它通过采样节点的邻域并聚合邻域信息来学习节点表示。
#### 2.1.1 邻域聚合机制
GraphSAGE使用一种称为LSTM(长短期记忆)的循环神经网络来聚合邻域信息。LSTM可以捕获序列中的长期依赖关系,非常适合处理图数据中节点之间的复杂关系。
聚合过程如下:
1. **采样邻域:**对于每个节点,从其邻域中随机采样一个固定大小的子集。
2. **计算节点表示:**使用LSTM将采样邻域中每个节点的表示聚合为一个向量。
3. **更新节点表示:**将聚合后的向量与原始节点表示连接起来,得到更新后的节点表示。
#### 2.1.2 采样策略
采样策略是GraphSAGE算法的关键部分。它决定了从邻域中采样的节点。有两种常见的采样策略:
* **均匀采样:**从邻域中随机采样节点。
* **负采样:**从邻域中采样负节点,即与目标节点没有连接的节点。
负采样可以帮助算法学习区分正例和负例,从而提高分类性能。
### 2.2 GraphSAGE算法的变体
GraphSAGE算法有多种变体,旨在提高其性能或适应不同的任务。
#### 2.2.1 GraphSAGE++
GraphSAGE++是GraphSAGE的扩展,它引入了多层聚合机制。在每一层,算法从上一层的聚合结果中采样邻域,并使用LSTM进行聚合。多层聚合可以捕获更深层次的邻域信息。
#### 2.2.2 GraphSAGE-GAT
GraphSAGE-GAT是GraphSAGE与图注意力网络(GAT)的结合。GAT是一种注意力机制,它可以赋予不同邻域节点不同的权重。通过结合GAT,GraphSAGE-GAT可以学习更具辨别力的节点表示。
**代码示例:**
```python
import dgl
import torch
import torch.nn as nn
import torch.nn.functional as F
class GraphSAGE(nn.Module):
def __init__(self, in_feats, out_feats, k):
super(GraphSAGE, self).__init__()
self.in_feats = in_feats
self.out_feats = out_feats
self.k = k
self.lstm = nn.LSTM(in_feats, out_feats)
def forward(self, graph
```
0
0
相关推荐
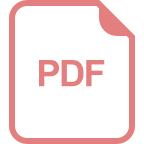
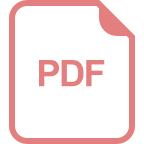
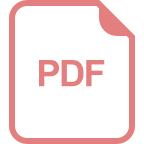





