优化DETR模型的注意力机制
发布时间: 2024-02-22 23:14:15 阅读量: 34 订阅数: 33 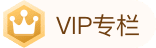
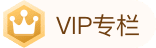
# 1. 引言
## 1.1 研究背景
在目标检测领域,深度学习模型一直是研究的热点。近年来,随着Transformer等模型的兴起,端到端的目标检测模型也相继涌现。其中,DETR(DEtection TRansformer)模型作为一种全新的思路,通过引入注意力机制实现了目标检测的端到端训练,取得了令人瞩目的成果。
## 1.2 研究意义
DETR模型的问世引发了对目标检测模型的新思考,其引入的注意力机制也让人们看到了更多优化的可能性。优化DETR模型的注意力机制不仅可以提升目标检测的准确性,还可以对注意力机制在神经网络中的应用进行更深入的探索。
## 1.3 研究目的
本文旨在探讨如何优化DETR模型中的注意力机制,提升目标检测性能,同时对注意力机制在神经网络中的作用进行深入分析和探讨。
## 1.4 文章结构
本文将从DETR模型的概述开始,接着介绍注意力机制在神经网络中的作用,然后分析当前注意力机制存在的问题,提出优化的思路并进行实验分析,最后对本文进行总结并展望后续研究方向。
# 2. DETR模型概述
### 2.1 DETR模型简介
The DETR (DEtection TRansformer) model is a state-of-the-art object detection model that revolutionizes the traditional two-stage object detection pipeline by using a transformer-based architecture.
### 2.2 DETR模型结构解析
The DETR model comprises a convolutional neural network (CNN) backbone for image feature extraction, which is then fed into a transformer encoder-decoder architecture to generate object queries and predict bounding boxes.
### 2.3 DETR模型在目标检测中的应用
The DETR model has shown remarkable performance in various object detection tasks, achieving competitive results with faster and more accurate predictions than traditional models. Its end-to-end desig
0
0
相关推荐
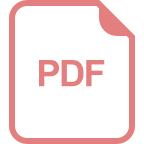





