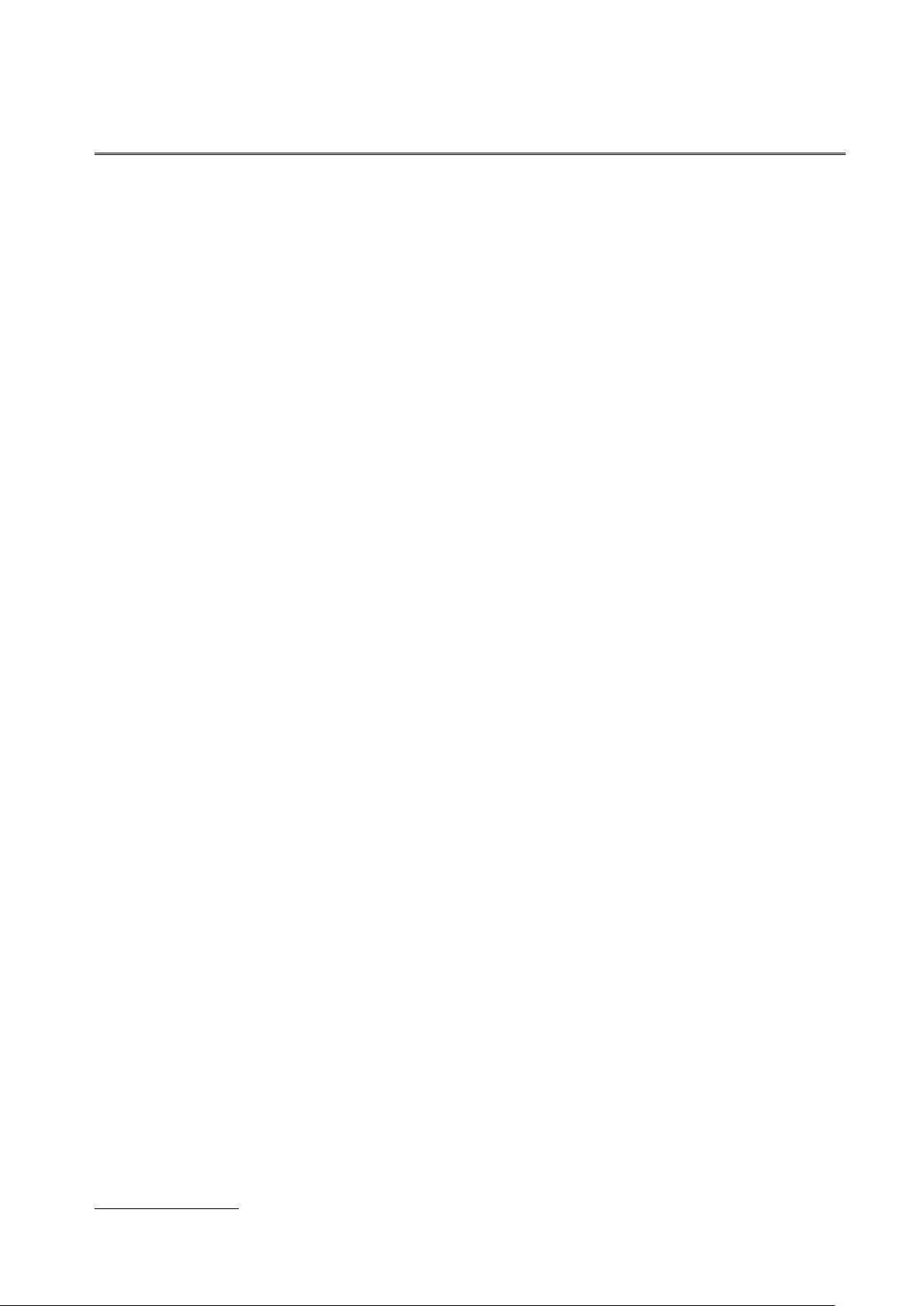
第 28 卷第 2 期
2011 年 2 月
控 制 理 论 与 应 用
Control Theory & Applications
Vol. 28 No. 2
Feb. 2011
多多多项项项式式式光光光滑滑滑的的的支支支持持持向向向量量量回回回归归归机机机
任 斌
1,2
, 程良伦
1
(1. 广东工业大学 自动化学院, 广东 广州 510090; 2. 东莞理工学院 电子工程学院, 广东 东莞 523808)
摘要: 光滑函数将不光滑的模型变为光滑模型, 改善支持向量回归机的回归性能和效率, 从而降低计算的复杂性.
寻找性能更好的光滑函数是研究光滑向量回归机的一个关键问题. 本文用级数展开的方法得出了ε–不敏感的支持
向量回归机|x|
2
ε
的一类新的光滑函数. 证明了这类函数的性能, 它能满足任意阶光滑的要求, 也能达到任意给定的
逼近精度. 实验结果表明, 随着光滑阶数的提高, 逼近精度和回归性能也相应提高. 从而为支持向量回归机和相关
研究领域提供了一类新的、性能更好的多项式光滑函数.
关键词: 支持向量回归机; ε–不敏感损失函数; 光滑; 多项式函数
中图分类号: TP311 文献标识码: A
Polynomial smoothing support vector regression
REN Bin
1,2
, CHENG Liang-lun
1
(1. Faculty of Automation, Guangdong University of Technology, Guangzhou Guangdong 510090, China;
2. Faculty of Electronic Engineering, Dongguan University of Technology, Dongguan Guangdong 523808, China)
Abstract: Smoothing functions can transform the unsmooth support vector regressions into smooth ones, and thus better
regression results can be obtained. It has been one of the key problems to seek a better smoothing function in this field for
a long time. Using the series expansion, a new class of polynomial smoothing functions is proposed for the |x|
2
ε
function in
ε-insensitive support vector regressions. Their important properties are then discussed. It is shown that the approximation
accuracy and smoothness rank of polynomial functions can be as high as required. The experimental results show that as
the smoothness rank of polynomial functions increases, the approximation accuracy and the regression performance are
correspondingly improved. Therefore, the new class of polynomial functions provides better performance for smoothing
the support vector regression.
Key words: support vector regression; ε-insensitive loss function; smoothing; polynomial function
文文文章章章编编编号号号: 1000−8152(2011)02−0261−05
1 引引引言言言(Introduction)
光滑函数可使原来不光滑模型变成光滑模型,
在一些重要的数学规划中得到了成功应用
[1∼5]
. 特
别是在分类问题和回归问题中获得了良好的效
果
[2,5∼7]
.
将光滑函数用于回归问题, 就是要解决原回归模
型中ε–不敏感损失函数平方不光滑的问题
[6]
. 按照
解决分类问题的基本思路, 2005年Lee等人用p
2
ε
–函
数作为光滑函数, 对 目 标 函 数 作 光 滑 逼 近, 提出
了ε–不敏感的支持向量回归机模型ε–SSVR
[6]
, 并采
用 了 快 速 的Newton-Armijo算 法 求 解. 结 果 表 明,
ε–SSVR的 效 果(回 归 性 能 和 效 率)比 传 统 的
LIBSVM
[8]
和SVM
light
[9]
算法好. 然而, 是否存在以
及如何寻找性能更好的光滑函数, 则是人们期待
解决的问题
[1∼3,5,6]
. 为解决此问题, 文献[10]用插
值函数, 给出了一类多项式光滑函数的一个递推公
式和复合函数的方法, 提出了两步求的基本思路, 虽
然理论上可行, 但实际应用时求出多项式函数的过
程非常繁琐, 本文用函数的级数展开法给出此类光
滑函数的通用公式, 经证明, 本文给出的光滑函数有
更好的光滑性能, 可以满足任意阶光滑的要求, 而且
可以达到任意给定的逼近精度, 从而为支持向量回
归机提供了新的光滑函数, 实验的结果表明, 随着多
项式光滑函数阶数的提高, 逼近精度和回归性能也
相应提高.
2 支支支 持持持 向向向 量量量 回回回 归归归 机机机(Support vector regres-
sion)
为了便于分析, 本文也采用文献[10]的定义关
于 自 变 量x的ε–不 敏 感 损 失 函 数 记 为|x|
ε
, |x|
ε
=
max{0, |x|−ε}. ε–不敏感损失函数的平方记为|x|
2
ε
.
数据集S = {(x
1
, y
1
), ··· , (x
m
, y
m
)} ⊆ R
n
×R,
令矩阵A = [x
1
x
2
··· x
m
], x
i
是一个n维向量, 每
收稿日期: 2009−12−17; 收修改稿日期: 2010−03−25.
基金项目: 国家自然科学基金资助项目(60673132); 广东省自然科学基金资助项目(07117421); 省部产学研资助项目(2009B090300242).