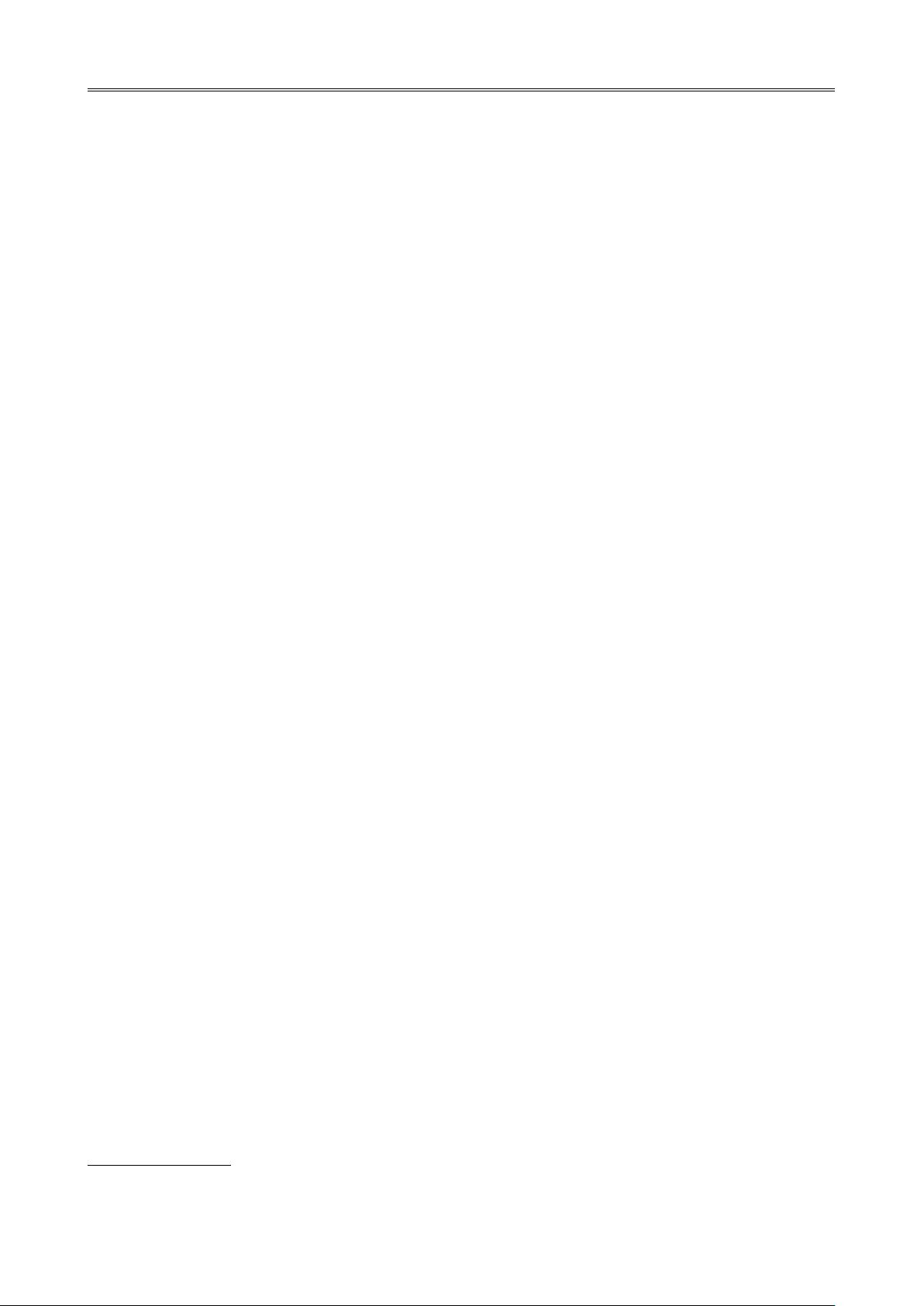
第 34卷 第 11期 控 制 与 决 策 Vol.34 No.11
2019年 11月 Control and Decision Nov. 2019
文章编号: 1001-0920(2019)11-2479-06 DOI: 10.13195/j.kzyjc.2018.0229
具有融合罚约束的低秩结构化稀疏表示目标跟踪算法
田 丹
1,2†
, 张国山
1
, 谢英红
2
(1. 天津大学 电气自动化与信息工程学院,天津 300072;2. 沈阳大学 信息工程学院,沈阳 110044)
摘 要: 现有的低秩稀疏表示目标跟踪算法在目标突然运动和严重遮挡等情况下, 经常出现跟踪漂移现象. 为此,
提出一种具有融合罚约束的低秩结构化稀疏表示目标跟踪算法. 首先, 利用混合 L
1,2
范数稀疏表示候选粒子的局
部斑块, 增强对斑块间结构信息的描述, 从而保护候选粒子间及其局部斑块间空间布局结构以解决遮挡问题; 然
后, 借鉴融合 Lasso 模型的建模思想, 在目标表观模型中引入融合罚项, 约束稀疏系数差分的绝对值, 以保证表示系
数稀疏性的同时, 使其连续性差异亦稀疏, 从而限制表示系数轮廓具有平滑性, 易于获取跳跃信息, 适应目标的突
然运动; 最后, 利用核范数低秩约束目标表观的时域相关性, 以适应目标的外观变化. 实验结果表明, 所提出的算法
能够适应复杂场景下的跟踪任务,特别是在目标形变、遮挡、突然运动等情况下具有更好的适应性.
关键词: 目标跟踪;稀疏表示;混合范数;低秩表示;融合罚;粒子滤波
中图分类号: TP391 文献标志码: A
Object tracking via low-rank and structural sparse representation with
fused penalty constraint
TIAN Dan
1,2†
, ZHANG Guo-shan
1
, XIE Ying-hong
2
(1. School of Electrical and Informatin Engineering,Tianjin University,Tianjin 300072,China;2. School of
Information Engineering
,
Shenyang University
,
Shenyang 110044
,
China)
Abstract: Tracking drift usually occurs in the existing low-rank sparse representation based object tracking algorithm
when the object facing abrupt motion and serious occlusion.Therefore, a low-rank structural sparse representation based
object tracking algorithm with fused penalty constraint is proposed.Firstly, candidate particles and their local patches
learn their sparse representations using L
12
mixed-norm, which can describe the structure information among the patches,
and solve the occlusion problem by preserving the spatial layout structure relationship among candidate particles and
their local patches. Then, based on the idea of the fused Lasso model, the fused penalty item is introduced into the object
appearance model to constrain the absolute value of the sparse coefficient difference, which can make the representation
coefficient and their continuity difference sparse. This can restr ict the outline of the representation coefficient smoothly,
acquiring the jumping information easily, so as to adapt abrupt motion. Furthe more, the low rank constraint based on
the nuclear norm is used to restrain the temporal correlation of the objective appearance, so as to adapt the appearance
change. The experimental results show that the proposed algorithm can track objects in complicated scenes, and has
better adaptability for object deformation, occlusion and abrupt motion.
Keywords: object tracking;sparse representation;mixed norm;low-rank representation;fused penalty;particle filter
0 引
视频目标跟踪是计算机视觉领域的一个重要研
究内容,已广泛应用于军事制导、人机交互、安防监控
等领域. 但随着应用范围的逐渐扩展, 该技术经常面
临目标外观变化、严重遮挡、光照骤变等问题,严重影
响跟踪效果,导致跟踪漂移.
考虑到遮挡位置具有稀疏性特征, 基于稀疏表
示
[1-5]
的目标表观建模有利于抑制遮挡因素的影响,
但是该方 法 中目标模 板 缺乏图像 特征信息. 低 秩
约束
[6-8]
能将目标表观建模在低维子空间, 提取候
选目标的全局子空间结构, 描述更为丰富的图像特
征, 增强跟踪对位置和光照变化的鲁棒性. 因此, 融
合低秩和稀疏表示能够增强跟踪的精确性和鲁棒
性
[9-10]
. Sui等
[11]
在粒子滤波框架下, 联合全局粒子的
帧间子空间结构关系和相邻斑块的局域相关性, 通过
局域低秩稀疏表示建模目标表观; Zhong 等
[12]
融合
收稿日期: 2018-02-28;修回日期: 2018-08-13.
基金项目: 国家自然科学基金项目(61703285, 61473202);沈阳市科技计划项目(17-231-1-14).
†
通讯作者. E-mail: www.sltd2008@163.com.