SSD算法在人脸检测中的应用与优化
"该资源是一篇关于基于SSD算法的人脸目标检测的研究论文,由杨璐和吴陈共同撰写,发表于2019年。文章探讨了如何使用SSD算法改进人脸检测,并通过添加负样本和应用正则化防止过拟合,以提升模型的精度和鲁棒性。实验在MXnet框架下进行,结果表明模型的mAp性能提升到0.997,并且在处理遮挡、光照和小目标时表现优秀。" 本文主要关注的是基于SSD(Single Shot MultiBox Detector)算法的人脸目标检测技术。SSD是一种用于目标检测的一体化深度学习算法,它能够在单次前向传播过程中预测出图像中的多个物体位置和类别,从而提高了检测效率。 首先,为了优化SSD模型对人脸的检测,研究者使用了公开的人脸数据集FDDB(Fddb Faces)对网络模型进行再训练和改进。通过使用不同的人脸数据集,他们能够使模型更好地适应各种人脸的特征,从而提升检测效果。 针对人脸检测过程中常见的过拟合问题,研究者采用了降噪自编码器(Denoising Autoencoder)方法。他们在输入数据集中添加负样本,即与人脸无关的图像,通过这种方式在训练模型时引入噪声,以增强模型的抗干扰能力。 此外,他们利用L1正则化来创建一个稀疏模型。L1正则化鼓励模型权重尽可能地稀疏,这意味着大部分权重将接近于零,有助于减少过拟合,同时保持模型对高维特征的有效处理,增强了模型的泛化性能。这样,在网络的迭代训练过程中,可以有效地降低噪声并防止模型陷入过拟合状态。 最后,研究人员应用了非极大值抑制(Non-Maximum Suppression,NMS)算法。NMS是目标检测中的关键步骤,用于从多个检测框中筛选出最有可能包含目标的那一个,从而确定最终的人脸检测窗口,有效地减少了冗余的检测结果。 实验在MXnet深度学习框架下进行,结果显示,经过噪声处理的人脸检测模型的平均精度(mean average precision, mAp)提升到了0.997,这表明模型的检测性能得到了显著改善。同时,模型在面对遮挡、光照变化以及小目标等复杂情况时,仍然保持了较高的鲁棒性和快速的收敛速度。 关键词包括:卷积神经网络(CNN)、SSD算法、NMS算法、正则化以及人脸检测,这些关键词反映了研究的主要内容和技术手段。这篇论文对于理解SSD算法在人脸检测中的应用,以及如何通过正则化和负样本训练来优化模型性能具有重要参考价值。
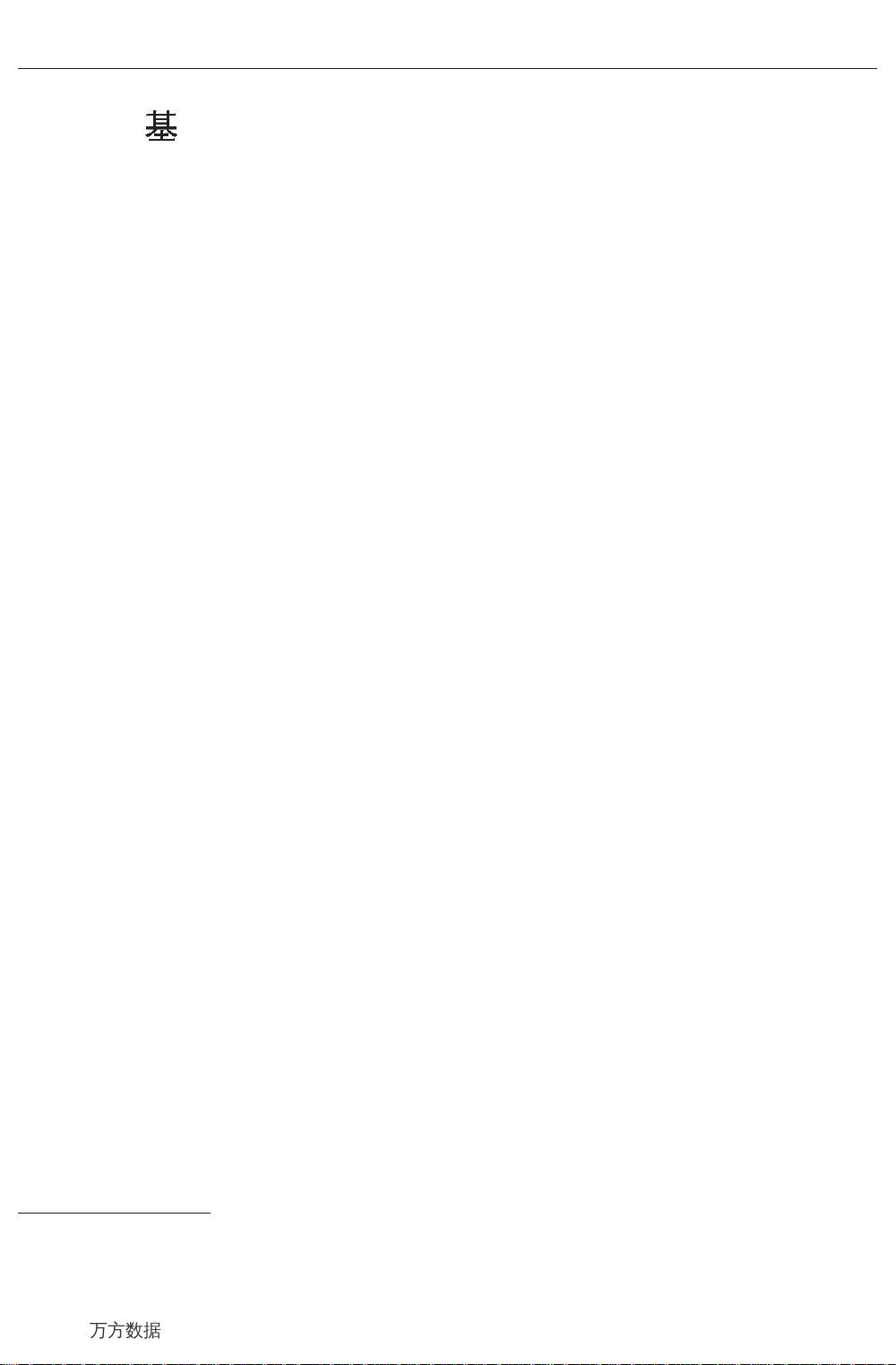
下载后可阅读完整内容,剩余4页未读,立即下载
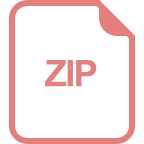

















- 粉丝: 31
- 资源: 801
我的内容管理 展开
我的资源 快来上传第一个资源
我的收益
登录查看自己的收益我的积分 登录查看自己的积分
我的C币 登录后查看C币余额
我的收藏
我的下载
下载帮助

最新资源
- C++标准程序库:权威指南
- Java解惑:奇数判断误区与改进方法
- C++编程必读:20种设计模式详解与实战
- LM3S8962微控制器数据手册
- 51单片机C语言实战教程:从入门到精通
- Spring3.0权威指南:JavaEE6实战
- Win32多线程程序设计详解
- Lucene2.9.1开发全攻略:从环境配置到索引创建
- 内存虚拟硬盘技术:提升电脑速度的秘密武器
- Java操作数据库:保存与显示图片到数据库及页面
- ISO14001:2004环境管理体系要求详解
- ShopExV4.8二次开发详解
- 企业形象与产品推广一站式网站建设技术方案揭秘
- Shopex二次开发:触发器与控制器重定向技术详解
- FPGA开发实战指南:创新设计与进阶技巧
- ShopExV4.8二次开发入门:解决升级问题与功能扩展

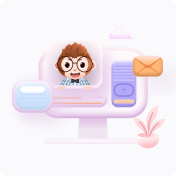
