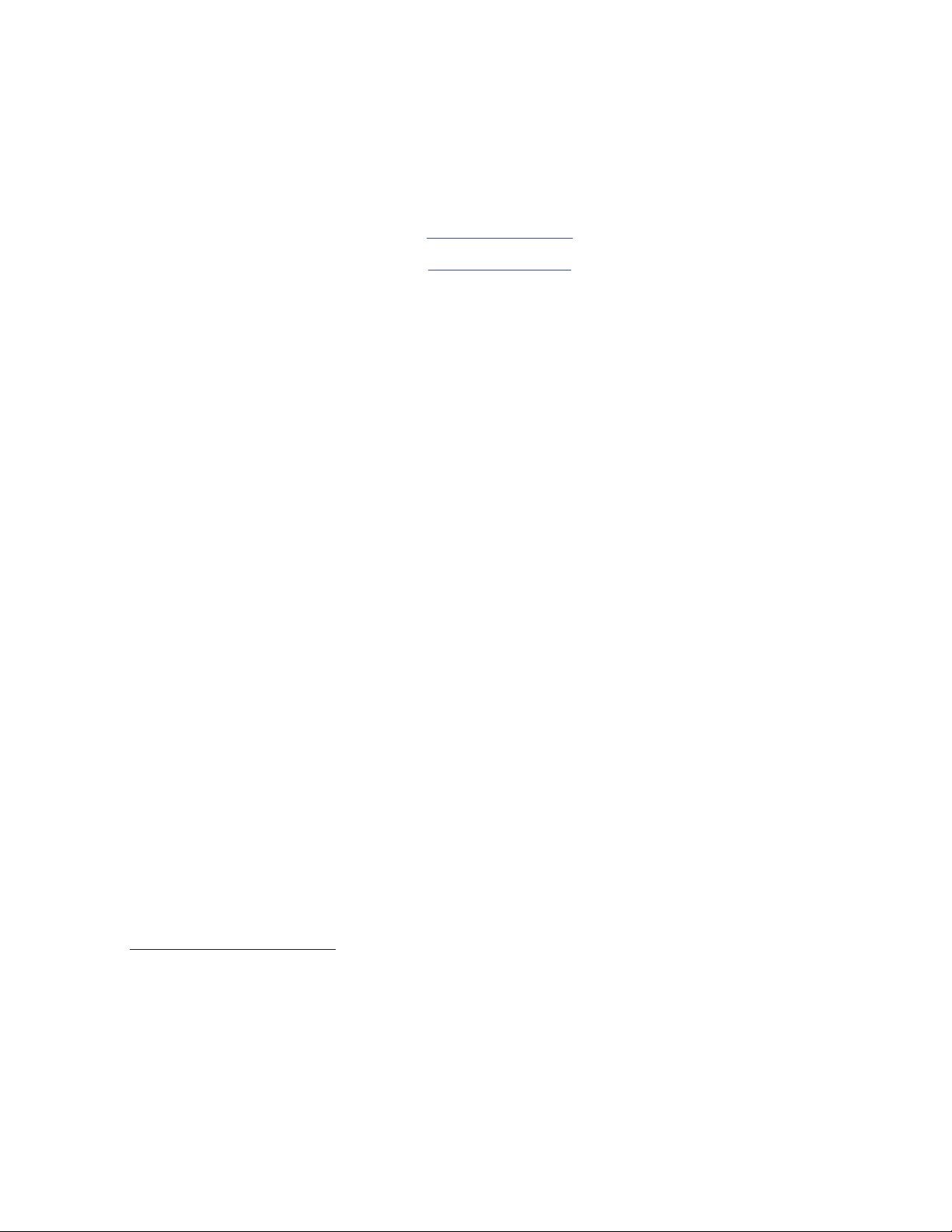
1 INTRODUCTION
With functional navigation computer, it is realizable for
MEMS IMUs / GPS that yields small size and low cost to
provide relatively precise parameter estimates [1, 2].
Theoretically, typical Kalman filter is truly capable of
estimating the navigation parameters under the conditions
of knowing the exact system model and the process/
measurement noise statistic characteristics. However,
accepting real system’s certain restrictions, linearized state
equation or measurement equation themselves, either
explicitly or implicitly, is not sufficiently accurate to
describe a wide range of kinematics phenomena. Moreover,
when it comes to the issue of achieving accurate navigation
estimates and better filter convergences, from the
mathematical perspective, interest should be focused more
upon deeper statistic characteristics analysis of
measurement noise than upon simple iterative Kalman
process improvements.
Typical Sage-Husa adaptive filtering algorithm forms the
basis of estimating system noise variances by means of
iterative calculation [3-5]. Owing to this, a class of
improved methods has been derived, strictly falling within
the basic Sage-Husa filtering process. Along with thorough
This work is supported by National Nature Science Foundation of
China under Grant 61503073; Natural Research Fund of Science and
Technology Department, Jilin Province under Grant 20170101125JC;
Science and Technology Research Fund of Education Department,
Jilin Province under Grant JJKH20170103KJ; Research Fund for
Distinguished Young Scholars of Jilin City under Grant 20166005.
research, the fuzzy adaptive design is introduced to correct
the time-varying measurement noise variance matrix, by
which, filtering accuracy may be enhanced considerably [6].
Inspired by Ref. [6], normal cloud model adaptive design is
proposed for a class of uncertain inferences in terms of
cloud drops, expectation
x
E
, entropy
n
E
and hyper entropy
e
H
concepts, realizing the uncertain and qualitative
knowledge representation concerned with randomness and
fuzziness. Noting that, compared with Sage-Husa design,
normal cloud model adaptive filtering simplifies the whole
estimation process, which then reduces the computational
work accordingly. The elaborate design procedures will be
presented in section 3, to begin with, the mathematical
model of Kalman filtering process of MEMS IMUs/GPS is
shown in the following section.
2 MATHEMATICAL MODEL OF MEMS
IMUs/GPS
MEMS IMUs are MEMS-based inertial measurement units,
being termed MIMUs. In this frame work, an ideal
positioning model of integrated navigation is achieved by
combining MIMUs with a miniature GPS receiver [7]. It’s
worth noting that, typical loosely-coupled MIMUs/GPS can
be seen as a low-cost solid state INS/GPS, so for Kalman
filter state model anatomy, the equation is correctly
encompassed by basic inertial navigation error equation. As
for the measurement model part, considering the navigation
parameters (which have been selected as the state variables),
the velocity/position difference between, that calculated by
MIMU/GPS Information Fusion: Normal Cloud Model Based Fuzzy Adaptive
Filtering
Xia Linlin
1,2
, Zhao Yao
1
, Ma Wenjie
3
, Su Chenxi
1
, Huang Fadong
1
1. School of Automation Engineering, Northeast Electric Power University, Jilin 132012, China
2. Intelligence Robot Collaborative Innovation Group, Northeast Electric Power University, Jilin 132012, China
E-mail:
prettylin521@aliyun.com
3. Science College, Northeast Electric Power University, Jilin 132012, China
E-mail:
mawenjie@neepu.edu.cn
Abstract: As a consequence of the statistical characteristics of noise changes with time or surrounding environment,
typical MEMS IMUs/ GPS can hardly provide the ideal navigation accuracy as expected. A normal cloud model based
fuzzy adaptive filtering (NCMFAF) is proposed for optimal state estimates, and the essential part of this derived filtering
consists in its positive effect on updating the covariance difference degree between theoretical residuals and actual ones
even with the large external variation of navigation process. Specifically, Normal cloud model module in cases is adopted
as an adaptive controller, fulfilling the coefficient adjustment of measurement noise variance involved in typical Kalman
filter, which, in turn, improves the performance of Kalman filter in dealing with the time/ environment -varying noise.
With the numerical simulation being carried out, the results indicate the NCMFAF process can respond to this sudden
statistical characteristics change of noise in real time, and the estimates accuracy of velocity/position is proved to be
dramatically enhanced by reference to the dynamic environment statistics.
Key Words: Normal Cloud Model, Fuzzy Adaptive Filtering, MEMS IMUs/ GPS, Information Fusion, Statistical
Characteristics, Estimates Accuracy, Kalman Filter
4076
978-1-5386-1243-9/18/$31.00
c
2018 IEEE