Keras深度学习中的混淆矩阵自动生成技巧

资源摘要信息:"在深度学习和机器学习领域,混淆矩阵是评估模型分类性能的重要工具之一。它通过展示实际类别与模型预测类别之间的关系,帮助我们直观理解模型在各个类别的分类准确性。Keras是一个流行的开源深度学习库,它提供了用于构建和训练深度神经网络的高级API。TensorFlow2是谷歌开发的一个开源机器学习框架,它支持大规模的深度学习应用。Python3.7是目前广泛使用的编程语言,它在数据科学和机器学习领域中占据了非常重要的地位。
利用Keras进行深度学习模型开发时,我们可以利用内置函数或自定义函数来生成混淆矩阵。在本例中,提供了一个名为`plot_confusion.py`的Python脚本文件,该文件旨在使用matplotlib库来绘制混淆矩阵图表。matplotlib是Python中最常用的绘图库,它能够生成高质量的图形,这对于模型结果的可视化是必不可少的。
在这个过程中,首先需要运行深度学习模型并获得预测结果,然后根据预测结果和真实标签生成混淆矩阵数据。生成的数据通常是一个二维数组,其行代表实际类别,列代表预测类别,对角线上的元素表示模型正确分类的数量,而非对角线上的元素则表示被错误分类的数量。通过这种方式,我们可以清晰地看到模型在不同类别上的表现。
使用plot_confusion.py文件,可以自动化这一过程,使得开发者能够快速地通过视觉化图表来评估模型性能。这不仅提高了效率,同时也使得非技术人员能够更容易理解模型的分类结果。
本资源的标题`plot_confusion_keras_混淆矩阵_plotconfusion_`明确指出了资源的核心功能,即利用Keras和Python来生成和绘制混淆矩阵图表。该资源描述了所需的技术栈包括深度学习、Keras、TensorFlow2以及Python3.7,并且附带了相关的标签以方便在项目中引用和搜索。文件`plot_confusion.py`是该资源的具体实现文件,它很可能是包含了一系列函数的Python脚本,这些函数能够接受模型的预测结果和实际标签作为输入,然后输出一个格式化的混淆矩阵图像。
在实际应用中,混淆矩阵不仅帮助我们识别模型在哪些类别上的表现不佳,而且还可以用作模型调优的依据。例如,如果一个类别经常被错误分类为另一个类别,那么我们可能需要增加该类别样本的数据量,或者调整模型结构和参数以改善分类效果。
总结来说,本资源为深度学习开发者提供了一种快速、便捷的方法来评估和优化他们的模型分类性能,通过自动生成可视化图表的方式,使得模型评估过程更加直观和高效。"
2021-01-07 上传
2020-09-19 上传
2023-06-06 上传
2020-09-16 上传
点击了解资源详情
点击了解资源详情
2023-05-10 上传
2023-05-29 上传
2023-05-25 上传
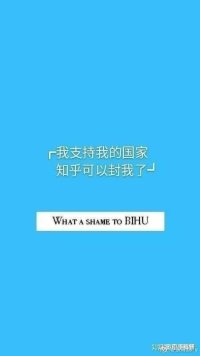
慕酒
- 粉丝: 53
- 资源: 4823
最新资源
- 全国江河水系图层shp文件包下载
- 点云二值化测试数据集的详细解读
- JDiskCat:跨平台开源磁盘目录工具
- 加密FS模块:实现动态文件加密的Node.js包
- 宠物小精灵记忆配对游戏:强化你的命名记忆
- React入门教程:创建React应用与脚本使用指南
- Linux和Unix文件标记解决方案:贝岭的matlab代码
- Unity射击游戏UI套件:支持C#与多种屏幕布局
- MapboxGL Draw自定义模式:高效切割多边形方法
- C语言课程设计:计算机程序编辑语言的应用与优势
- 吴恩达课程手写实现Python优化器和网络模型
- PFT_2019项目:ft_printf测试器的新版测试规范
- MySQL数据库备份Shell脚本使用指南
- Ohbug扩展实现屏幕录像功能
- Ember CLI 插件:ember-cli-i18n-lazy-lookup 实现高效国际化
- Wireshark网络调试工具:中文支持的网口发包与分析