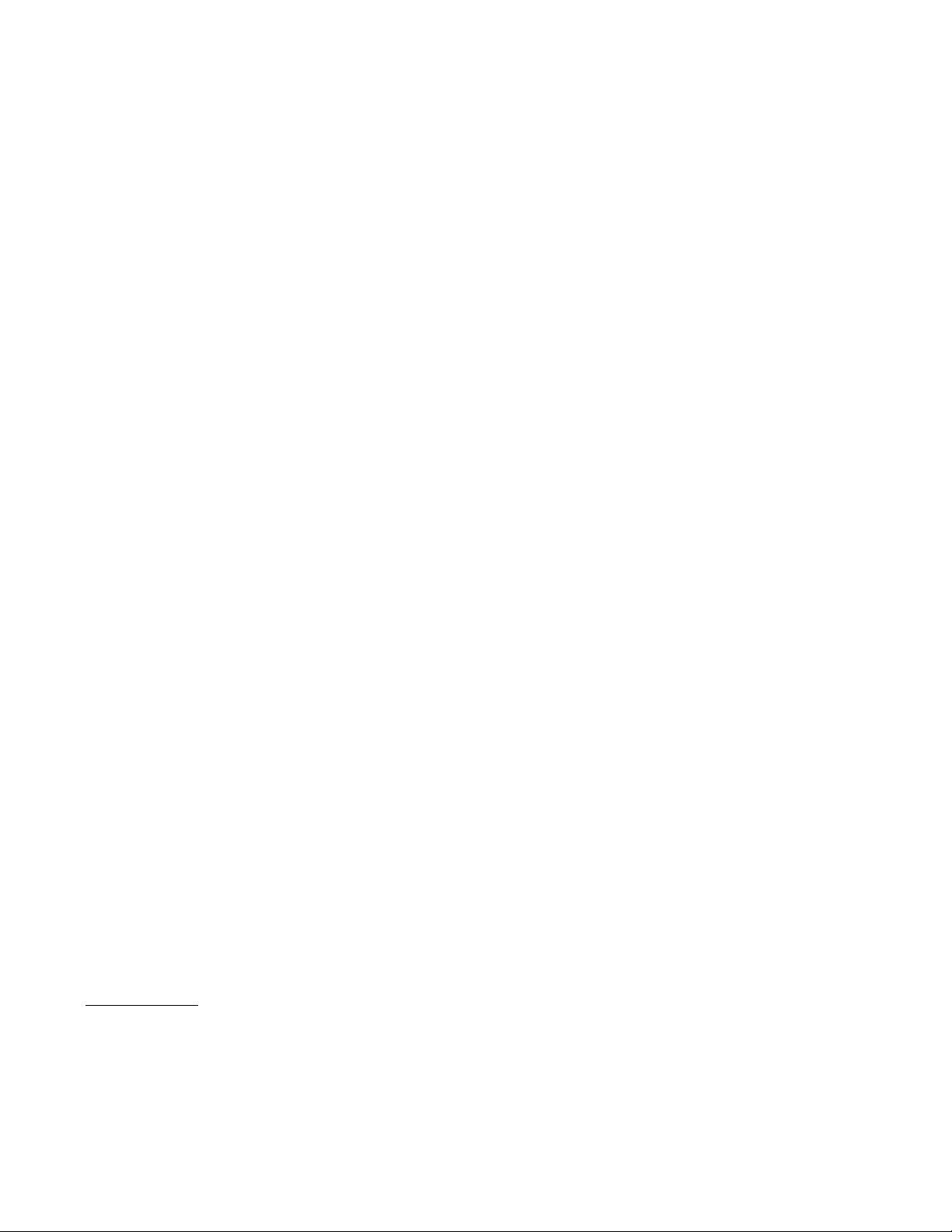
Speaker Adaptation of Acoustic Models Using Correlations of
Training Transfer Vectors
Satoshi Takahashi
*
and Shigeki Sagayama
NTT Human Interface Laboratories, Yokosuka, Japan 239-0847
SUMMARY
The authors proposed for acoustic models based on
the hidden Markov model a method that involves applying
constraints to the model structure and tying the models
parameters in order to improve the training efficiency.
Conventionally, the tied structure of an acoustic model is,
mostly, defined by tying several adjacent parameters and
expressing them with a single representative parameter.
This method can be regarded as a tying method based on
the parameters values, under the assumption that adjacent
parameters, usually, exhibit similar behavior. As opposed
to this concept, the current study proposes a tied structure
with consideration of transfer (movement) of parameters.
A large volume of training data was used to measure
transfer of each parameter during training, and tying rela-
tionships regarding the transfer vectors were organized
between parameters performing statistically similar move-
ments. In particular, in the current study, the authors con-
centrated on mean vectors of fundamental distributions and
followed movements of these mean vectors during training
of initial models (speaker-independent models) by acoustic
data from different speakers. The structure was defined by
identifying the mean vectors characterized by strong corre-
lation of movements during training, and tying their corre-
sponding transfer vectors. Speaker adaptation tests
confirmed high training efficiency of the model obtained as
a result of this tying. © 2000 Scripta Technica, Syst Comp
Jpn, 31(14): 7482, 2000
Key words:
HMM; tying of parameters; training
transfer vector; acoustic model; speaker adaptation.
1. Introduction
For acoustic models based on statistical approach
such as the hidden Markov model (HMM), the model
structure represents an important problem. There are two
main points to consider regarding the structure of speaker-
independent acoustic models in the speech recognition
field. First, the model structure should efficiently reflect the
training data. Following the recent expansion of use of
speech databases, a large volume of training data has be-
come available for generating speaker-independent acous-
tic models. However, even in this situation, it is, typically,
data of limited volume. Therefore, in order to use the data
more efficiently, one must prepare a model structure of high
performance. Second, model structure should enable easy
adaptation (to speaker, noise, speaking style, and so on)
even with a small data volume. For example, speaker adap-
tation based on speech data from a specific speaker is
adopted to speaker-independent acoustic models. For fast
speaker adaptation, even with a small volume of speech
data, it is necessary to prepare a model structure that can be
© 2000 Scripta Technica
Systems and Computers in Japan, Vol. 31, No. 14, 2000
Translated from Denshi Joho Tsushin Gakkai Ronbunshi, Vol. J82-D-II, No. 3, March 1999, pp. 324331
*
Presently with Hokkaido Business Communications Headquarters of
NTT.
Presently with the Japan Advanced Institute of Science and Technology,
Hokuriku.
74