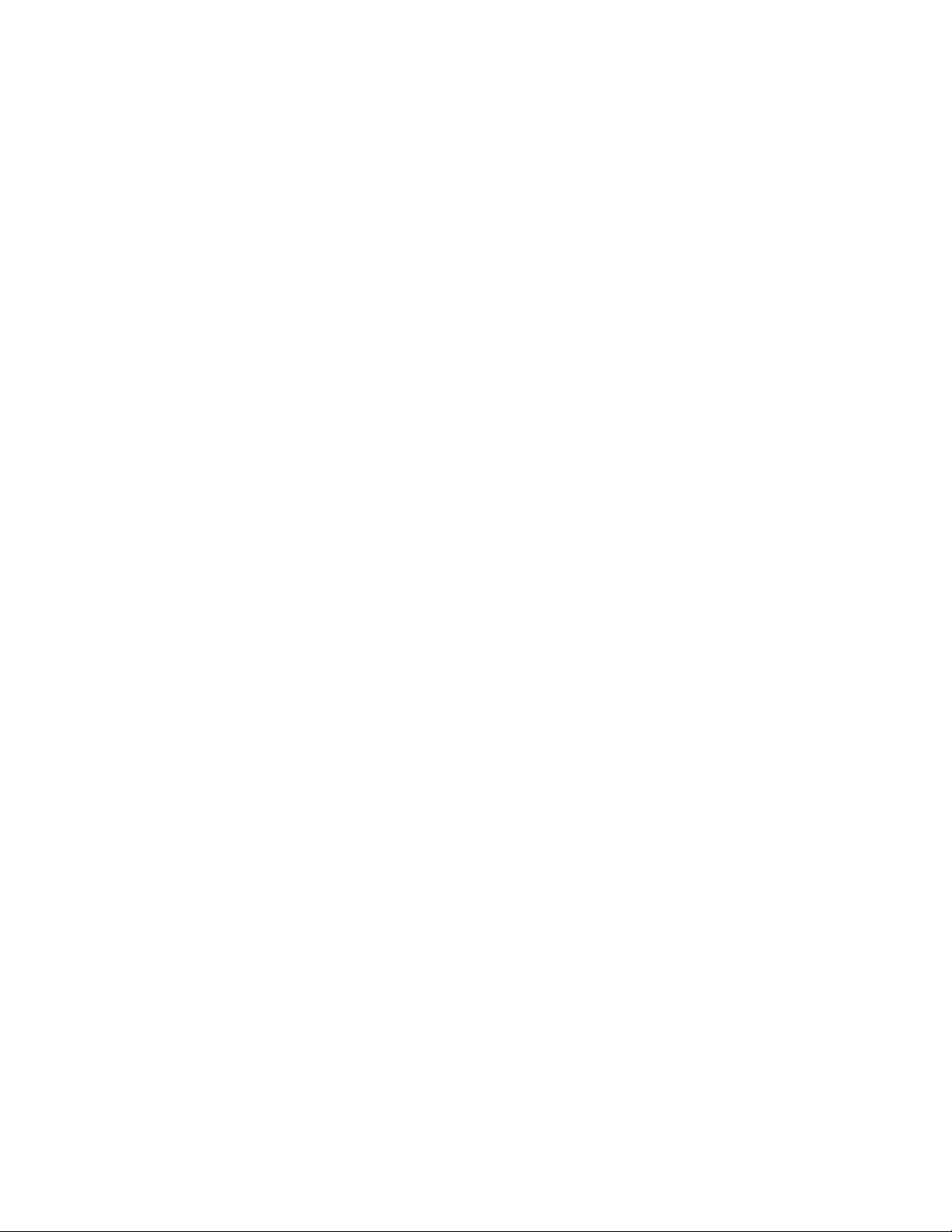
estimates station dwelling time is proposed in (Buchmueller, Weidmann, & Nash, 2008) that
works based on the input parameters of vehicle type (number, position, width and level of
doorways), infrastructure (platform level) and demand (number and distribution of passengers).
Workflow nets and triangular fuzzy numbers are applied in (Martinek & Zemlicka, 2013) to
study the issues associated with predicting train operation conflicts. However, the most common
limitation of these work is that there is no real operation data that can illustrate and validate
them practically in detail.
There are two types of delay propagation and recovery models, one is based on simulation
and theoretical hypotheses, and the other is based on historical data. In the former type, a study
that attempts to reproduce the situation of train operation under the knock-on delay was found
to be an effective measure to recover the knock-on delays (Zhang & Lv, 2009). An analytical
stochastic model of train delay propagation in stations that estimates the knock-on delays of
trains caused by route conflicts and late transfer connections realistically is established (Yuan
& Hansen, 2007). It was found that the railway system and capacity utilization are sensitive to
disturbances and delays and trains may be forced to stop or decelerate and result in losing of
running time in sections or additional dwelling time at stations (Bai, Ho, & Mao, 2011).
Andersson et al. studied the relationship between train delay and stops at junction areas, where
there are resources utilization conflicts (Andersson, Peterson, & Törnquist Krasemann, 2015).
Meester presented a fairly general but not too detailed model for delay propagation and showed
that it is possible to derive secondary delay distributions from primary delay distributions, and
some attentions are paid to the issue of primary delay propagation (Kingston, 2007). In further,
Hansen et al. presented a delay propagation model in which train path conflicts and dispatching
decisions are taken into account, and parameters are estimated by offline statistical analysis of
historical train detection data (Mehta, Rossiger, & Montigel, 2010). However, this work does
not address the delay probabilities at each station and in each section. An intelligent onboard
system for the high-speed trains is preliminarily implemented rest on the historical data
presented in (Khan & Zhou, 2010) that can provide better detection ability than other models
when processing real-time events stored in high-speed train Juridical Recording Unit. Jouni
uses a data-mining approach for analyzing rail transport delay chains, with data from passenger
train traffic on the Finnish rail network; however, the data from a train operation process limited
to one month’s data (Wallander & Makitalo, 2012).
The size of primary delays and the ability of a timetable to absorb primary delays are two
important ingredients for timetable robustness and performances measurement (Fernandez,
Cucala, & Sanz, 2004). For the research of delay recovery, the concept of resilience has been
introduced to measure the system ability in absorbing perturbations, and its ability to recover
rapidly from perturbations (Adjetey-Bahun, Birregah, Châtelet, & Planchet, 2016). Hars had
studied the delay probability distributions and found the mean recovery rate of 0.13 minute/km
based on historical data, which is used in modeling delay recovery. However, this mean value
can hardly reflect the real recovery ability of each station and section that can affect the
prediction accuracy of the model (Khadilkar, 2016).
Reviewing the literature, reveals that train delay management is a concern both in theory
and practice, and modeling of PDR and its factors based on train operation records has not been
done systematically. We present our work on modeling of PDR, using train operation records
from Wuhan–Guangzhou high-speed railway in Guangzhou Railway Bureau, as an example of