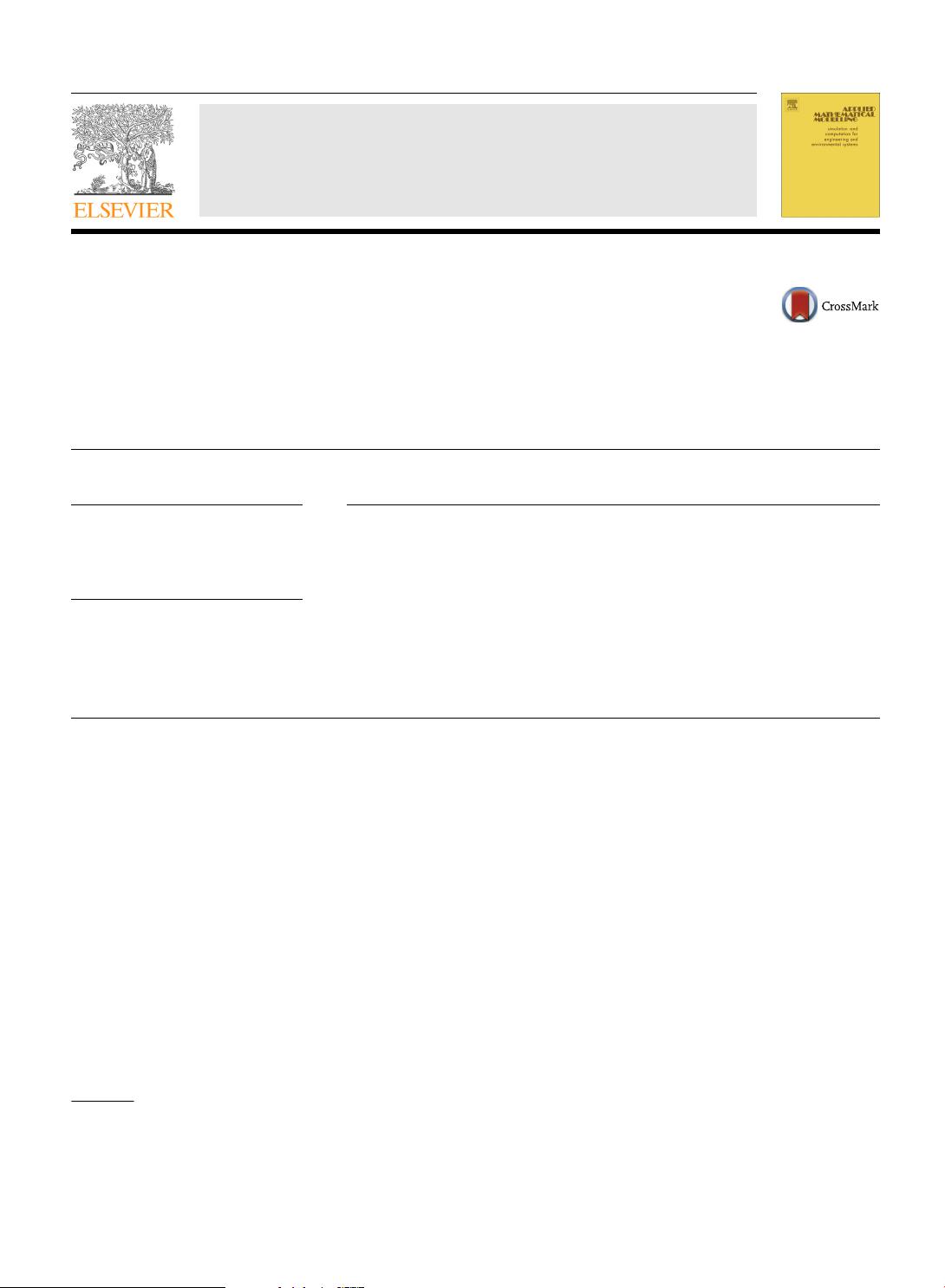
Auxiliary model based recursive and iterative least squares
algorithm for autoregressive output error autoregressive
systems
Qibing Jin, Liting Cao, Ruigeng Yang
⇑
, Qi Wang, Zhu Wang
Institute of Automation, Beijing University of Chemical Technology, Beijing 100029, PR China
article info
Article history:
Received 14 September 2013
Received in revised form 12 January 2015
Accepted 10 February 2015
Available online 10 March 2015
Keywords:
Least squares
Auxiliary model
Recursive method
Iterative method
Autoregressive output error autoregressive
(AR-OEAR) model
abstract
This paper considers the stochastic systems described by the autoregressive output error
autoregressive (AR-OEAR) models. The AR-OEAR model is a special output error model
which has an extra autoregressive term of the output signal. Basing on the auxiliary model
idea, the recursive method and the iterative principle, the auxiliary model based recursive
and iterative least squares algorithms are proposed to estimate the parameters of the
autoregressive output error autoregressive system which contains the autoregressive out-
put error system model and the autoregressive noise model, respectively. The simulation
results indicate that the proposed algorithms can work well.
Ó 2015 Elsevier Inc. All rights reserved.
1. Introduction
System identification plays an important role in various scientific and engineering applications, including simulation,
optimization, fault detection and prediction [1,2]. Many algorithms are proposed to solve this problem, such as the least
squares methods [3–5], the gradient methods [6–8], the maximum likelihood identification methods [9,10], and some intel-
ligent algorithms such as the GA algorithm [11], the PSO algorithm [12], etc. Among these algorithms, the least squares
methods have wide applications in system identification [13–15]. Other ideas combining with the least square method gen-
erate many new algorithms, including the multi-innovation least squares algorithms [16], the hierarchical least squares algo-
rithms [17,18], the auxiliary model identification algorithms [19,20], the recursive algorithms [21,22] and the iterative
identification algorithms [23–26].
In the area of the auxiliary model identification, Ding et al. presented a combined parameter and output estimation of
dual-rate systems using an auxiliary model [19]. Wang et al. proposed auxiliary model based least square algorithm for
Hammerstein OEAR systems [20]. In the area of recursive identification, Wang and Ding proposed three-stage recursive least
squares parameter estimation for controlled autoregressive autoregressive systems [21]. Zhang and Cui proposed a bias com-
pensation recursive least squares methods for stochastic systems with colored noise [22]. In the area of iterative identifica-
tion, Liu and Lu discussed least square based iterative identification for a class of multirate systems [23]. Ding et al. proposed
http://dx.doi.org/10.1016/j.apm.2015.02.038
0307-904X/Ó 2015 Elsevier Inc. All rights reserved.
⇑
Corresponding author at: Beisanhuan East Road 15, Chaoyang District, Beijing 100029, PR China. Tel.: +86 18132603930.
E-mail addresses: librgy@163.com, jinqibing@sina.com (R. Yang).
Applied Mathematical Modelling 39 (2015) 7008–7016
Contents lists available at ScienceDirect
Applied Mathematical Modelling
journal homepage: www.elsevier.com/locate/apm