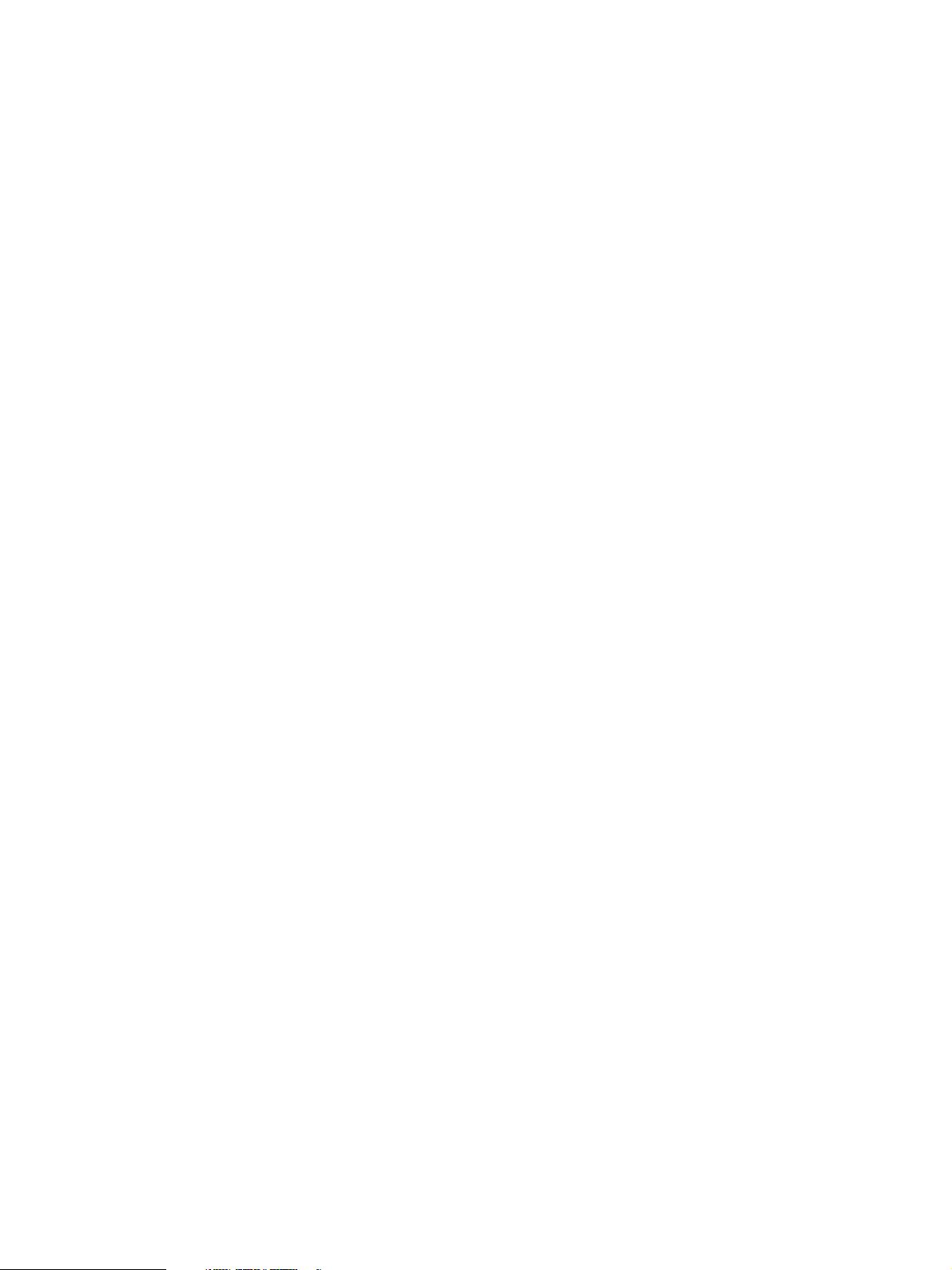
Wang BioData Mining
(2015) 8:13
Page 3 of 15
To minimize user effort, Vukicevic et al. [6] applied genetic algorithms to achieve the
best prognostic performances relevant for clinicians (i.e., correctness, discrimination and
calibration). The only 2 use r dependent tasks were data selection (input and output vari-
ables) and the evaluation of the ANN threshold probability with respect to regret theory
(RT). After optimally configuring ANNs with respect to these criteria, the clinical use-
fulness was evaluated by the RT Decision Curve Analysis. Tsao e t al. [7] develop ed an
ANN model to predict prostate cancer pathological staging in patients prior to receiv-
ing radic al prostatectomy. This exper imental study examined the cases of 299 patients
undergoing retro-pubic radical prostatectomy. In this investigation, the validation was
assessed by using the current Partin Tables for the Taiwanese population. ANN induced
larger AUCs and provided a more accurate pre diction of the pathologic stage of prost ate
cancer.
Bayesian networks (BNs) are characterised by the use of the probabilistic approach in
solving problems and encompass the uncertainty of specific occurrences. The origin of
BNs is ba sed on probability distribution, which can be graphically depicted. Alexander et
al. [8] applied the SEER database (1969 to 2006) to form a clinical dec ision support system
for the real-time estimation of the overall survival (OS) rate of colon cancer patients. The
BN model accurately estimated OS with an area under the receiver-operating character-
istic curve of 0.85. They significantly improved upon the existence of AJCC stage-specific
OS estimates. Furthermore, they determined the significant differences in OS b etween
low- and high-risk cohorts. Khan et al. [9] used Bayesian method to derive the poste-
rior density function for the parameters and the predictive inference for future survival
times from the exponentiated Weibull model, assuming that the observed breast can-
cer survival data follow such type of model. The Markov chain Monte Carlo method
was used to determine the inference for the parameters. They found that the exponen-
tiated Weibull model fits the male survival data. Mean predictive survival times, 95%
predictive intervals, predictive skewness and kurtosis were obtained. Jong et al. [10]
introduced a hybrid model that combined ANN and BN to obtain a good estimation
of prognosis and a good explanation of the results. In this research, the SEER database
(1973 to 2003) was employed to construct and evaluate the proposed models. Nine
clinically acceptable var iables were selected to be incorporated into the nodes of the pro-
posed models. Consequently, the hybrid model achieved the highest area under the curve
value of 0.935, and the corresponding values of ANN and BN were 0.930 and 0.813,
respectively.
Other machine learning models have also been applied to solve the problems in pre-
dicting cancer survivability. Molina e t al. [11] suggested that an incremental learning
ensemble of a suppor t vector machine (SVM) must be implemented to adapt to the work-
ing conditions in medical applications and to improve the effectiveness and robustness
of the system. These studies calculated the probability estimation of cancer structures
by using SVM and performed the corresponding optimisation with a heuristic method
together w ith a three-fold cross-validation methodology. Mahmoodian et al. [12] de vel-
ope d a new algorithm on the basis of fuzzy association rule mining to identify fuzzy
rules and significant genes. In this study, different sub sets of gene s that have been
selected by different me thods were used to separately generate primary fu zzy classifiers.
Subsequently, the researchers administered their proposed algorithm to mix the genes
associated with the primary classifiers and to generate a novel classifier.