【Application Analysis of Causal Inference】: The Application of Causal Inference and Counterfactual Reasoning in Linear Regression
发布时间: 2024-09-14 17:59:53 阅读量: 9 订阅数: 13 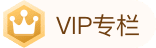
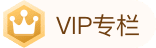
# 1. Introduction to the Application of Causal Inference and Counterfactual Reasoning in Linear Regression
In practical data analysis, causal inference and counterfactual reasoning are among the important methods for evaluating causal relationships between events. In linear regression, applying causal inference and counterfactual reasoning techniques can help us analyze the causal relationships between data more accurately and provide decision support. Through learning this chapter, you will gain an in-depth understanding of the principles and methods of causal inference in linear regression, explore how to introduce these theories into practical applications, and provide more convincing conclusions for data analysis.
# 2. Fundamental Knowledge of Causal Inference
Causal inference is an important branch of statistics that aims to identify causal relationships between variables through data analysis, rather than just demonstrating their correlations. In fields such as machine learning, medical research, social sciences, and business decision-making, causal inference plays a crucial role. In this chapter, we will introduce the basics of causal inference, including an overview of causal inference, the difference between causal relationships and correlations, and the construction of causal diagrams.
### 2.1 Overview of Causal Inference
Causal inference aims to determine the impact of a certain factor on a specific outcome, rather than just identifying the correlation between them. In this section, we will briefly outline the basic concepts of causal inference.
#### 2.1.1 The Difference Between Causal Relationships and Correlations
In statistics, causal relationships and correlations are often confused by people. Correlation indicates that there is some association between two variables, but it does not mean that a change in one variable will cause a change in another. Causal relationships clearly state that a change in one variable causes a change in another variable.
#### 2.1.2 Construction of Causal Diagrams
Causal diagrams are one of the important tools of causal inference, which are used to describe the causal relationships between different variables. By constructing causal diagrams, we can clearly understand the causal connections between variables and help us perform effective causal inference analysis.
### 2.2 Methods of Causal Inference
In addition to basic concepts, understanding the methods of causal inference is also crucial. In this section, we will introduce several commonly used causal inference methods.
#### 2.2.1 Limitations of Ordinary Linear Regression
Ordinary linear regression is a common statistical analysis method, but it has limitations in causal inference. It cannot directly explain the causal relationships between variables, is susceptible to the influence of confounding variables, and results in bias.
#### 2.2.2 Introduction to the Instrumental Variable Method
The instrumental variable method is a causal inference method for solving endogeneity problems. By introducing an exogenous good instrumental variable, we can eliminate the confusion between causal effects and interfering variables, thereby estimating causal effects more accurately.
#### 2.2.3 Regression Discontinuity Design
Regression discontinuity design is a special regression method for handling mutations at a certain threshold. By observing the changes near the threshold, we can infer whether a causal relationship exists.
#### 2.2.4 Detailed Explanation of Propensity Score Matching
Propensity score matching is a method for dealing with selection bias by matching the observed data of the treatment group and the control group to reduce the estimation bias of causal effects caused by selection bias.
In the following chapters, we will delve into the application and practical cases of causal inference, further understanding its importance in practical data analysis.
# 3. The Role of Counterfactual Reasoning in Causal Inference
### 3.1 Analysis of the Concept of Counterfactual Reasoning
In the theoretical framework of causal inference, counterfactual reasoning plays a crucial role. It is a form of inference that analyzess "what would happen if something happened" by hypothetically reconstructing historical events to deduce causal relationships between things. Next, we will delve into the definition, characteristics, and its connection with causal inference.
#### 3.1.1 Definition and Characteristics
Counterfactual reasoning is a hypothetical reconstruction of historical events, that is, if the situation of historical events had been different, what would the outcome have been? Its characteristics include:
- Inference is based on assumptions about historical events;
- The process of inference is based on known facts and logical reasoning;
- Can help understand causal connections between things.
#### 3.1.2 The Connection Between Counterfactual Reasoning and Causal Inference
Counterfactual reasoning is closely related to causal inference, with an inherent logical connection. In causal inference analysis, counterfactual reasoning can help reveal the causal relationships between events, thereby better understanding the potential mechanisms that affect the results of causal inference. By analyzing the results under d
0
0
相关推荐
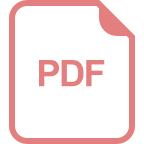
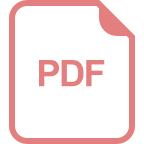
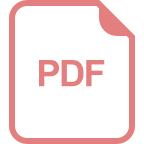





