【Discussion on Gradient Descent Algorithm】: Application of Gradient Descent Algorithm in Linear Regression Optimization
发布时间: 2024-09-14 18:01:17 阅读量: 18 订阅数: 39 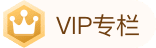
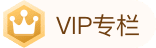
# 1. In-depth Understanding of Gradient Descent Algorithm
The gradient descent algorithm is a pivotal component in the realm of optimization algorithms, characterized by its simplicity and robust power. Widely utilized in machine learning to seek the optimal solution for loss functions, the fundamental concept involves iteratively updating parameters in the direction of the descending gradient of the objective function, gradually approaching the optimal solution. There are various variants of gradient descent, such as batch gradient descent, stochastic gradient descent, and mini-batch gradient descent, each suited to different scenarios.
### Learning Objectives:
- Understand the basic principles of gradient descent.
- Master the characteristics and应用场景of different gradient descent algorithms.
- Deeply analyze the relationship between gradient descent and linear regression optimization.
This chapter will guide you through an in-depth study of the gradient descent algorithm, laying a solid theoretical foundation for subsequent linear regression optimization.
# 2. Linear Regression Fundamentals
### 2.1 Understanding Linear Regression Principles
#### 2.1.1 Linear Regression Model
Linear regression is a fundamental method of regression analysis, used to describe the linear relationship between independent variables and dependent variables. Its mathematical expression is as follows:
y = β_0 + β_1*x_1 + β_2*x_2 + ... + β_n*x_n
Where y is the dependent variable, x_i (i=1,2,...,n) are independent variables, and β_i are the corresponding coefficients for the independent variables.
#### 2.1.2 Least Squares Method Solution
The least squares method is a common parameter estimation technique that fits model parameters by minimizing the sum of squared residuals between actual observed values and model predicted values. The specific formula is as follows:
\underset{\beta}{\min} \sum_{i=1}^{n}(y_i - \beta_0 - \sum_{j=1}^{p}\beta_j*x_{ij})^2
#### 2.1.3 Regression Evaluation Metrics
Common evaluation metrics in linear regression tasks include Mean Squared Error (MSE), Root Mean Squared Error (RMSE), and Coefficient of Determination (R^2), which are used to measure the goodness of model fit.
### 2.2 Linear Regression Practice
#### 2.2.1 Data Preparation and Feature Engineering
When undertaking a linear regression task, data preparation and feature engineering are the first steps. This includes data cleaning, feature selection, feature transformation, etc., to enhance the model's accuracy and generalization capabilities.
#### 2.2.2 Model Training and Evaluation
Next, input the prepared data into the linear regression model for training and evaluation. Fit model parameters using training data, then use test data to assess model performance, obtaining evaluation metrics for comparative analysis.
#### 2.2.3 Result Analysis and Optimization
Finally, based on the results of model training and evaluation, perform result analysis and optimization measures. The linear regression model can be optimized by adjusting features, trying different optimization algorithms, and tweaking hyperparameters, thereby enhancing the model's predictive and generalization abilities.
Through the above practical operations, you can better understand the basic principles of the linear regression model and apply it to real-world problems.
# 3. Principles of the Gradient Descent Algorithm
The gradient descent algorithm plays a crucial role in the field of machine learning, capable of effectively optimizing model parameters and serving as the foundation for many optimization algorithms. In this chapter, we will delve into the principles of the gradient descent algorithm, including the concept of gradients, batch gradient descent, stochastic gradient descent, and the specifics of mini-batch gradient descent algorithms.
### 3.1 Concept of Gradient
#### 3.1.1 Definition of Gradient
In mathematics, the gradient is a vector composed of a set of partial derivatives, representing the direction derivative of a function at a point in all directions. For the objective function J(θ), the gradient ∇J(θ) can be expressed as:
∇J(θ) = \begin{pmatrix} \dfrac{\partial J}{\partial \theta_1} \\ \dfrac{\partial J}{\partial \theta_2} \\ \vdots \\ \dfrac{\partial J}{\partial \theta_n} \end{pmatrix}
#### 3.1.2 Direction of Gradient Descent
The gradient descent algorithm updates parameters in the opposite direction of the gradient to gradually approach the optimal value of the objective function. The update rule is:
θ = θ - α ∇J(θ)
#### 3.1.3 Selection of Learning Rate
The learning rate α determines the step size of parameter updates; too large may cause oscillation, and too small may lead to slow convergence. Choosing an appropriate learning rate is crucial for the algorithm's performance.
### 3.2 Batch Gradient Descent
#### 3.2.1 Steps of Batch Gradient Descent Algorithm
Batch gradient descent computes the gradient using all samples and updates the parameters. Algorithm steps include:
1. Calculate the gradient of the entire training set;
2. Update parameters based on the gradient;
3. Repeat the above steps until convergence.
#### 3.2.2 Advantages and Disadvantages of
0
0
相关推荐
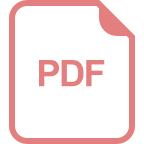
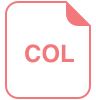
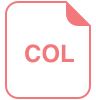
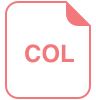
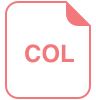
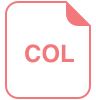
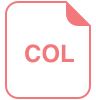
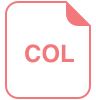
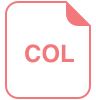