"Linear Regression Deep Dive": Principles and Basic Assumptions Uncovered
发布时间: 2024-09-14 17:37:03 阅读量: 23 订阅数: 45 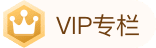
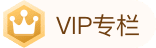
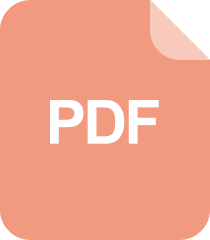
Linear Regression Analysis: Applications and Assumptions
# 1. Understanding the Basics of Linear Regression
Linear regression is a statistical method used for modeling and analyzing the relationships between variables. In data science and machine learning, linear regression is extensively used for prediction and estimation of numerical variables. Its fundamental principle is to describe the linear relationship between independent and dependent variables by fitting the best straight line. The linear regression model can be represented mathematically as: $y = mx + b$, where $y$ represents the dependent variable, $x$ represents the independent variable, $m$ represents the slope, and $b$ represents the intercept.
Through linear regression, we can understand the trends and relationships within the data, perform predictions and analyses, and provide a foundation for subsequent modeling and decision-making.
# 2. In-depth Analysis of Linear Regression Principles
### 2.1 Definition and Characteristics of Linear Regression
Linear regression is a statistical model used to establish linear relationships between variables, widely applied in data analysis and predictive modeling. Understanding the definition and characteristics of linear regression is crucial for an in-depth understanding of its principles.
#### 2.1.1 What is Linear Regression?
Linear regression is a model that uses independent variables (features) to predict dependent variables (targets) by finding a linear function to describe the relationship between them, usually represented as $y = wx + b$, where $w$ is the weight and $b$ is the bias term.
#### 2.1.2 Basic Assumptions of Linear Regression
Linear regression is based on several basic assumptions:
- Linearity: There is a linear relationship between the independent and dependent variables;
- Independence and Identical Distribution: Sample points should be independent and have the same distribution;
- Homoscedasticity: Each independent variable's effect on the dependent variable should be the same.
#### 2.1.3 Difference Between Linear and Nonlinear Relationships
A linear relationship refers to the relationship where the dependent variable changes proportionally with the increase of the independent variable, while a nonlinear relationship is not a direct proportional relationship. Linear regression is suitable for linear relationships, while nonlinear regression models are suitable for nonlinear relationships.
### 2.2 Mathematical Expression of Linear Regression
The mathematical expression of linear regression is one of the keys to understanding its principles. Let's systematically explore the mathematical expression of the linear regression model.
#### 2.2.1 Derivation of the Linear Regression Model Formula
In linear regression, our goal is to find the best-fit line that minimizes the error between predicted values and actual values. The best-fit line is obtained by minimizing the sum of squared residuals, mathematically expressed as:
$$\hat{y} = w_1x_1 + w_2x_2 + ... + w_nx_n + b$$
where $\hat{y}$ is the predicted value, $w_i$ is the weight of the feature, $x_i$ is the feature value, and $b$ is the bias term.
#### 2.2.2 Loss Function and Optimization Methods
In linear regression, the common loss function is Mean Squared Error (MSE), which is the mean of the squared differences between predicted values and true values. Optimization methods usually use gradient descent to continuously update weights and bias terms to minimize the loss function.
#### 2.2.3 Ordinary Least Squares and Its Applications
Ordinary Least Squares (OLS) is a commonly used method for estimating parameters in linear regression, solving for the optimal parameters by minimizing the sum of squared residuals. It is an analytical solution method that directly obtains the closed-form solution for regression coefficients.
The above content is part of the in-depth analysis of the principles of linear regression. Through in-depth exploration of the definition, characteristics, and mathematical expression of linear regression, we can better understand the working principle of the linear regression model.
# 3. Decoding the Basic Assumptions of Linear Regression
As a classic machine learning model, linear regression requires a series of basic assumptions to be met before application to ensure the reliability and effectiveness of the model. This chapter will deeply decode the basic assumptions of linear regression, including linearity, homogeneity, independence, and normality, to help readers better understand and apply linear regression models.
### 3.1 Linearity
#### 3.1.1 Discussion of Linear Relationships
In linear regression, we assume that there is a linear relationship between independent and dependent variables. A linear relationship means that the changes between variables are呈直线关系, that is, a unit change in the independent variable results in an equal proportional change in the dependent variable. By plotting scatter plots, the fitting of regression lines, and the observation of residual plots, we can preliminarily determine whether variables have a linear relationship.
#### 3.1.2 Verification of Linear Relationship Assumptions
The verification of linear relationship assumptions can be done using correlation coefficients and visualization tools. The correlation coefficient (Pearson correlation coefficient) ranges from -1 to 1, and the closer it is to 1, the stronger the linear correlation. Additionally, plotting scatter plots and observing the distribution of regression lines and residuals is an effective method for verifying linear relationships.
### 3.2 Homogeneity
#### 3.2.1 Interpretation of Homogeneity
Homogeneity refers to the homoscedasticity of error terms, meaning that the residual variance corresponding to different independent variable values should remain consistent. If the variance of the error terms does not satisfy the homogeneity assumption, it will lead to the inaccuracy and instability of the model.
#### 3.2.2 Methods for Judging Homogeneity Assumptions
The homogeneity assumption can be judged by plotting a scatter plot of squared residuals versus fitted values, observing whether residuals show a clear trend of change with the increase of fitted values. Formal tests of residual dispersion, such as the Breusch-Pagan test, can also be used to verify the homogeneity assumption.
### 3.3 Independence
#### 3.3.1 Test of Independence Between Independent Variables
In linear regression, independent variables should be independent of each other and not exhibit multicollinearity. By cal
0
0
相关推荐
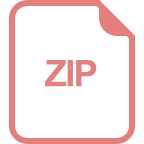
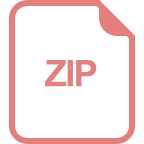
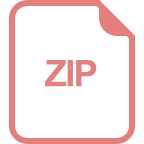
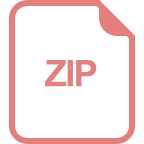
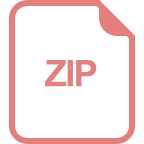
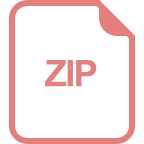
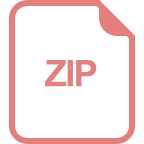
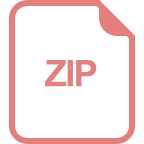