【Neural Network Expansion】: The Application of Neural Networks and Deep Learning Models in Linear Regression Problems
发布时间: 2024-09-14 18:05:57 阅读量: 25 订阅数: 43 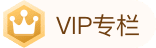
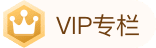
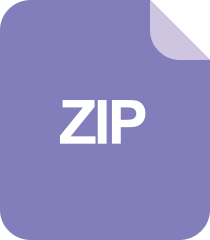
AVR单片机项目-ADC键盘(源码+仿真+效果图).zip
# 1. Introduction to the Application of Neural Networks and Deep Learning Models in Linear Regression Problems
In the realm of machine learning, neural networks and deep learning have become a hot topic, especially for their powerful capabilities in solving linear regression problems. This chapter will start with an introduction to the basics of neural networks, gradually guiding readers to a deep understanding of the fundamentals of linear regression, and then explore the specific applications of neural networks and deep learning in linear regression problems. Through discussions on performance evaluation methods, this chapter aims to help readers better understand the advantages of neural networks and deep learning models in solving linear regression problems. Ultimately, by analyzing practical examples, we will demonstrate how to leverage neural networks to address actual linear regression issues, providing readers with practical and valuable information.
# 2. Basic Knowledge of Neural Networks
Artificial neural networks are computational systems inspired by the nervous system, serving as mathematical models that simulate the information transmission between neurons in the human brain. In neural networks, the most basic unit is the artificial neuron. This section will introduce the artificial neuron model and the fundamentals of deep neural networks.
### 2.1 Artificial Neuron Model
The artificial neuron model is the basic component of neural networks, possessing the characteristics of a biological neuron, capable of processing input signals with weights and producing output. It mainly includes the following aspects:
#### 2.1.1 Perceptron Model
A perceptron is a simple artificial neuron model, consisting of an input layer, weights, an activation function, and an output layer. It works by multiplying input signals with corresponding weights and then processing the result through an activation function. The specific implementation is shown in the following table:
| Input | Weight | Calculation Formula |
|------|------|----------------------------------------|
| $x_1$ | $w_1$ | $z = x_1 \times w_1$ |
| $x_2$ | $w_2$ | $output = f(z)$ |
#### 2.1.2 Role of Activation Functions
Activation functions play a crucial role in neural networks by introducing non-linear factors, ***monly used activation functions include Sigmoid, ReLU, etc., specifically表现为:
```python
# Sigmoid Activation Function Example
def sigmoid(x):
return 1 / (1 + np.exp(-x))
```
#### 2.1.3 Forward Propagation Process of Neural Networks
The forward propagation of neural networks refers to the process where input sample data passes through each layer of the neural network to the output layer. During this process, each layer of neurons computes its output based on the output from the previous layer and passes it to the next layer. The specific flow is shown in the following mermaid flowchart:
```mermaid
graph TD;
A[Input Sample Data] --> B[Hidden Layer 1];
B --> C[Hidden Layer 2];
C --> D[Output Layer];
```
### 2.2 Deep Neural Networks
Deep neural networks refer to neural networks with multiple hidden layers, typically consisting of an input layer, several hidden layers, and an output layer. In deep neural networks, complex features can be learned through the combination of multiple layers of neurons. This section will focus on multi-layer perceptrons and gradient descent algorithms and backpropagation algorithms in neural networks.
#### 2.2.1 Multi-layer Perceptrons
A multi-layer perceptron is a typical deep neural network structure that can learn more abstract and complex features through multiple hidden layers. Its structure is shown as follows:
```mermaid
graph LR;
A[Input Layer] --> B[Hidden Layer 1];
B --> C[Hidden Layer 2];
C --> D[Output Layer];
```
#### 2.2.2 Gradient Descent Algorithm in Neural Networks
Gradient descent is a commonly used optimization algorithm that continuously adjusts model parameters by minimizing the loss function to improve model performance. In neural networks, the gradient descent algorithm can be used to update weights and biases, as specifically implemented below:
```python
# Gradient Descent Algorithm Example
def gradient_descent(weights, lr, grad):
weights = weights - lr * grad
```
#### 2.2.3 Backpropagation Algorithm
The backpropagation algorithm is a key algorithm for training neural networks in deep learning, continuously adjusting model parameters by calculating the gradients of output errors with respect to each layer's weights. The process can be illustrated by the following table:
| Step | Operation |
|------|-----------|
| Step 1 | Forward propagation to compute output values |
| Step 2 | Compute the value of the loss function |
| Step 3 | Backpropagation to compute gradients for each layer |
| Step 4 | Update model parameters |
In this section, we have delved into the fundamentals of neural networks, including perceptron models, activation functions, the forward propagation process, multi-layer perceptrons, gradient descent algorithms, and backpropagation algorithms. This knowledge will lay the foundation for subsequent chapters to explore the application of neural networks in linear regression problems.
# 3. Fundamentals of Linear Regression
Linear regression is one of the most common regression analysis methods in statistics, used to find the linear relationship model between independent and dependent variables. In this chapter, we will delve into the fundamentals of linear regression, including simple linear regression and multiple linear regression, along with related principles and applications.
### 3.1 Simple Linear Regression
#### 3.1.1 Principles of Linear Regression Model
The linear regression model is represented as: $y = wx + b$, where $y$ is the dependent variable (target value), $x$ is the independent variable (feature), $w$ is the weight (slope), and $b$
0
0
相关推荐
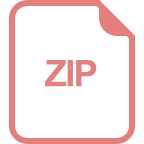
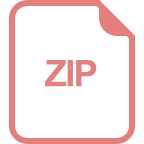
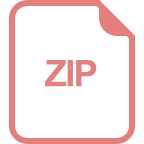
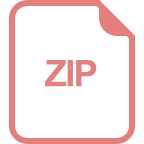
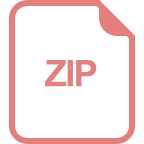
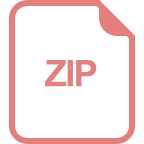
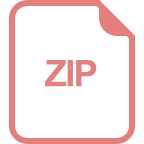
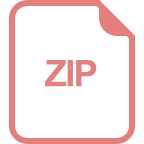