[Frontier Developments]: GAN's Latest Breakthroughs in Deepfake Domain: Understanding Future AI Trends
发布时间: 2024-09-15 17:06:01 阅读量: 17 订阅数: 15 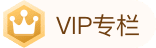
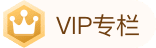
# 1. Introduction to Deepfakes and GANs
## 1.1 Definition and History of Deepfakes
Deepfakes, a portmanteau of "deep learning" and "fake", are technologically-altered images, audio, and videos that are lifelike thanks to the power of deep learning, particularly Generative Adversarial Networks (GANs). They have the potential to mislead viewers by manufacturing false information visually and aurally. The deepfake technology emerged in 2017, initially for creating pornographic videos, but it quickly evolved to spread across various fields including politics and entertainment.
## 1.2 Origin and Applications of GANs
Generative Adversarial Networks (GANs) were introduced by Ian Goodfellow in 2014, and they consist of two main parts: a generator and a discriminator. The generator creates fake data, while the discriminator tries to distinguish between the real and the fake. GANs have broad applications in image generation, image restoration, super-resolution, and beyond.
## 1.3 The Connection between GANs and Deepfakes
GANs are at the core of deepfake technology. With GANs, we can generate realistic fake images, audio, and videos, which is the primary method for creating deepfakes. However, this also brings challenges of legality and ethics, and how to effectively detect and prevent deepfakes has become an urgent issue to address.
# 2. The Fundamental Principles and Mathematical Foundations of GANs
## 2.1 The Theoretical Framework of GANs
### 2.1.1 Origin and Development of Generative Adversarial Networks
Generative Adversarial Networks (GAN), proposed by Ian Goodfellow and colleagues in 2014, marked a leap forward in the field of generative models, drawing inspiration from the zero-sum game in game theory. GAN utilizes two models—the Generator and the Discriminator—in an adversarial process to improve performance.
As research continued, variations such as DCGAN (Deep Convolutional Generative Adversarial Networks), WGAN (Wasserstein Generative Adversarial Networks), BigGAN, and StyleGAN were proposed, expanding the boundaries of GAN technology.
### 2.1.2 The Mathematical Model and Optimization Objective of GANs
The essence of GAN lies in the adversarial process, where the parameters of the generator and discriminator are continuously updated to achieve a state of Nash Equilibrium. Mathematically, the optimization goal of GAN can be represented as:
```
min_G max_D V(D, G) = E_x[log D(x)] + E_z[log(1 - D(G(z)))]
```
Where `D` is the discriminator, `G` is the generator, `x` represents real data samples, and `z` is random noise drawn from a prior distribution. The generator `G` aims to produce samples as close to the real data as possible, while the discriminator `D` tries to distinguish between generated samples and real samples.
## 2.2 Key Components of GANs
### 2.2.1 The Role and Structure of the Generator
The generator's task is to receive a random noise vector `z`, learn the distribution of real data, and generate samples that are as similar as possible to real data. The generator is typically realized by a multi-layer neural network, which can have dozens or even hundreds of layers.
The typical structure of a generator network includes multiple fully connected or convolutional layers, which may be followed by batch normalization layers and ReLU activation functions. Convolutional neural network structures are more common in image generation scenarios, as they effectively capture local features.
### 2.2.2 The Mechanism and Training of the Discriminator
The main task of the discriminator is to distinguish whether the input sample is real or generated by the generator. It is also a neural network, usually similar in structure to the generator. The discriminator improves performance by maximizing the probability of distinguishing real data from generated data.
During training, the discriminator tries to distinguish between real data and fake data generated by the generator. To enhance the performance of the discriminator, it needs to process both real and generated samples and provide a judgment. As training progresses, the generator learns to produce more realistic data to deceive the discriminator.
## 2.3 Challenges in the GAN Training Process
### 2.3.1 Mode Collapse Problem
Mode collapse is a common issue in GAN training. It refers to the generator beginning to produce samples from only a few distributions, ignoring other parts of the data space, resulting in reduced diversity.
There are various methods to address mode collapse, such as adding regularization terms, using historical data to combat the generator, or adopting more complex network structures. More advanced techniques like WGAN use the Wasserstein distance instead of the traditional objective function, which can effectively alleviate the mode collapse problem.
### 2.3.2 Loss Functions and Training Strategies
The design of the GAN loss function and training strategy is a key factor affecting its training effectiveness. Traditional GANs use cross-entropy loss functions, but there are some drawbacks, such as the difficulty in balancing the competition intensity between the generator and discriminator.
To optimize the training process, researchers have tried various methods, such as introducing label smoothing techniques, using gradient penalties to stabilize training, or applying gradient clipping with different strategies. In addition, some studies focus on improvements in model architecture, such as the self-attention mechanism introduced in BigGAN, all aimed at improving the training stability and generation quality of the model.
# 3. Practical Applications of GANs in Deepfakes
### 3.1 Basic Techniques of Deepfakes
#### 3.1.1 Overview of Deepfake Techniques for Images and Videos
Deepfake technology has gradually evolved into a comprehensive technology, including deep learning applications in images, videos, and voices. For images and videos, deepfake technology mainly relies on Generative Adversarial Networks (GANs) to achieve highly realistic fake content. This content can be anything from replacing a person's face, changing body movements, to applying one person's voice to another.
The emergence of deepfake technology is due to the demand for high-quality generated content. On the one hand, this brings good news to the film and entertainment industry, making the production of cooler special effects possible; on the other hand, it also brings many risks to society, such as using personal images and voices for inappropriate occasions, leading to privacy infringement and the spread of fake information.
#### 3.1.2 Deepfake Techniques for Voice Synthesis
Voice synthesis technology, also known as Text-to-Speech (TTS), has made significant progress. The voice synthesis technology in deepfakes can utilize GANs to generate realistic voices based on sound generation models such as WaveNet and Tacotron.
These technologies first collect a large amount of voice data and then use deep learning models to learn the characteristics of sound. The role of GAN here is to ensure, through the adversarial mechanism, that the generated sound is indistinguishable from the naturalness of real sounds. This technology has important application value in podcast production, voice assistants, and personalized education. However, similarly, it also brings the risk of being abused, such as deepfake-generated voices being used for fraud, defamation, and even impersonating public figures to make inappropriate statements.
### 3.2 Practical Applications of GANs in Image Deepfakes
#### 3.2.1 Facial Replacement and Expression Transfer Technologies
Facial replacement technology is mainly realized through GANs, among which one of the most famous models is DeepFake. This technology can seamlessly replace a person's face with another person's face while maintaining the naturalness of expressions and movements. The core of this technology lies in the generator's ability to produc
0
0
相关推荐
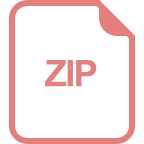
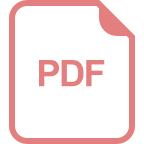
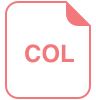
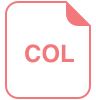





