【Theoretical Deepening】: Cracking the Convergence Dilemma of GANs: In-Depth Analysis from Theory to Practice
发布时间: 2024-09-15 16:31:54 阅读量: 36 订阅数: 31 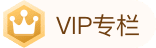
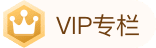
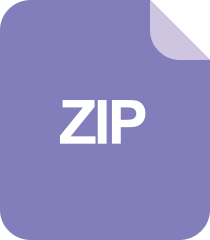
果壳处理器研究小组(Topic基于RISCV64果核处理器的卷积神经网络加速器研究)详细文档+全部资料+优秀项目+源码.zip
# Deep Dive into the Convergence Challenges of GANs: Theoretical Insights to Practical Applications
## 1. Introduction to Generative Adversarial Networks (GANs)
Generative Adversarial Networks (GANs) represent a significant breakthrough in the field of deep learning in recent years. They consist of two parts: the generator and the discriminator. The goal of the generator is to create data that is as similar as possible to real data, while the discriminator aims to accurately identify whether the data is real or generated by the generator. The two work in opposition to each other, jointly advancing the model.
### 1.1 The Basics of GAN Components and Operating Principles
The training process of GANs can be understood as a game between a "forger" and a "cop." The "forger" continuously attempts to create more realistic fake data, while the "cop" tries to more accurately distinguish between real and fake data. In this process, the capabilities of both sides improve, and the quality of the generated data becomes increasingly high.
### 1.2 GAN Application Domains
GAN applications are very broad, including image generation, image editing, image super-resolution, and data augmentation, among others. It can even be used to generate artworks, offering endless possibilities for artists and designers. Furthermore, GANs have tremendous potential in medical, game development, and natural language processing fields.
### 1.3 GAN Advantages and Challenges
The greatest advantage of GANs lies in their powerful generation capabilities, enabling them to generate highly realistic data without the need for extensive labeled datasets. However, GANs also face challenges, such as mode collapse, unstable training, and more. Addressing these issues requires a deep understanding of the principles and mechanisms of GANs.
# 2. Theoretical Foundations and Mathematical Principles of GANs
## 2.1 Basic Concepts and Components of GANs
### 2.1.1 The Interaction Mechanism Between Generators and Discriminators
Generative Adversarial Networks (GANs) consist of two core components: the Generator and the Discriminator. The Generator's task is to create data that looks real from random noise, while the Discriminator's task is to distinguish generated data from real data.
The training of the Generator relies on feedback from the Discriminator. During training, the Generator continuously generates data, the Discriminator evaluates its authenticity, and provides feedback. The Generator uses the information provided by the Discriminator to continuously adjust its parameters to improve the quality of the generated data.
To understand the interaction between the Generator and Discriminator, we can compare it to an adversarial game. In this game, the Generator and Discriminator compete and promote each other until they reach a balanced state where the Generator can produce data that is almost indistinguishable from real data, and the Discriminator cannot effectively differentiate between generated data and real data.
```python
# Below is a simplified code example of a GAN model
# Import necessary libraries
from keras.layers import Input, Dense, Reshape, Flatten, Dropout
from keras.layers import BatchNormalization, Activation, LeakyReLU
from keras.layers.advanced_activations import LeakyReLU
from keras.models import Sequential, Model
from keras.optimizers import Adam
# Architecture definition for the generator and discriminator
def build_generator(z_dim):
model = Sequential()
# Add network layers here
return model
def build_discriminator(img_shape):
model = Sequential()
# Add network layers here
return model
# Model building and compilation
z_dim = 100
img_shape = (28, 28, 1) # Example using the MNIST dataset
generator = build_generator(z_dim)
discriminator = build_discriminator(img_shape)
# During discriminator training, only the discriminator's weights are trained, and the generator's weights are set to non-trainable
discriminator.trainable = False
# Next, define the GAN model
z = Input(shape=(z_dim,))
img = generator(z)
valid = discriminator(img)
combined = Model(z, valid)
***pile(loss='binary_crossentropy', optimizer=Adam(0.0002, 0.5))
# Training logic
# Omit specific training code, but generally includes generating batches of fake and real data, then training the discriminator, followed by fixing the discriminator parameters and training the generator, iterating this process
```
### 2.1.2 Loss Functions and Optimization Goals
The training goal of GANs is to make the performance of the Generator and Discriminator as close as possible, which is typically represented as a minimax problem. Ideally, when the Generator and Discriminator reach a Nash equilibrium, the data generated by the Generator will not be effectively distinguished by the Discriminator.
Mathematically, GAN loss functions are typically defined using cross-entropy loss functions to measure the difference between generated data and real data. The Discriminator's loss function minimizes the gap between the probability of real data being recognized as true and the probability of generated data being recognized as true. Similarly, the Generator's loss function minimizes the probability of generated data being recognized as true.
```python
# GAN loss functions can take the following form
# For the Discriminator
def discriminator_loss(real_output, fake_output):
real_loss = binary_crossentropy(tf.ones_like(real_output), real_output)
fake_loss = binary_crossentropy(tf.zeros_like(fake_output), fake_output)
total_loss = real_loss + fake_loss
return total_loss
# For the Generator
def generator_loss(fake_output):
return binary_crossentropy(tf.ones_like(fake_output), fake_output)
```
When training GANs, we generally need to train the Discriminator and Generator alternately until the model converges. In practice, this process may require a large number of iterations and parameter adjustments to achieve the desired effect.
## 2.2 Mathematical Model Analysis of GANs
### 2.2.1 Probability Distributions and Sampling Theory
To understand how GANs work, it is necessary to first understand the concept of probability distributions. In GANs, the Generator samples from a latent space (usually a multidimensional Gaussian distribution) and then maps it to the data space through a neural network. The Discriminator tries to distinguish these generated data from the real data.
Sampling theory is a series of theories studying how to extract samples from probability distributions. In GANs, the Generator's sampling process needs to capture the key characteristics of the real data distribution to generate high-quality synthetic data. To achieve this, the Generator needs to continuously learn the structure of the real data distribution during training.
Mathematically, we can represent the Generator's sampling process as a mapping function \(G: Z \rightarrow X\), where \(Z\) is the latent space, and \(X\) is the data space. This process is parameterized by a neural network, with parameters \(\theta_G\) mapping the latent variable \(z\) to the data \(x\).
### 2.2.2 Generalization Ability and Model Capacity
Generalization ability is a machine learning model's ability to predict unseen data based on training data. The generalization ability of GANs is crucial for generating realistic data. Model capacity refers to the complexity of the model's ability to fit data. A model with too low capacity may lead to underfitting, while a model with too high capacity may lead to overfitting.
In GANs, generalization ability and model capacity are influenced by the architecture of the Generator and Discriminator. Too simple models may not capture the real data distribution, while too complex models may overfit on the training data, leading to decreased generalization performance.
To balance model capacity and generalization ability, it is usually necessary to carefully design the network architecture, and regularization techniques such as Dropout or weight decay may also be needed.
## 2.3 Challenges in GAN Training
### 2.3.1 Theoretical Explanation of Mode Collapse Issues
Mode Collapse is a severe problem in GAN training, where the Generator starts to repeatedly generate almost identical data points and no longer covers all modes of the real data distribution. This leads to a decrease in the diversity of generated data and a weakening of the model's generalization ability.
The theoretical explanation of mode collapse is usually related to the problem of gradient vanishing. When the Generator generates certain data that the Discriminator cannot effectively distinguish, the gradient information the Generator receives will be very small, causing learning to stop or proceed very slowly, thus stopping the Generator from learning.
```python
# Below is a simplified GAN training code, showing where mode collapse issues may occur
# Define the training loop
def train(epochs, batch_size=128, save_interval=50):
# Data loading and preprocessing code omitted
for epoch in range(epochs):
# Omitting the training steps for the Generator and Discriminator
# Assuming that the model training does not sufficiently
```
0
0
相关推荐
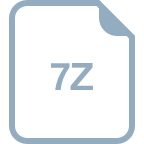
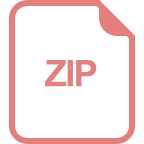
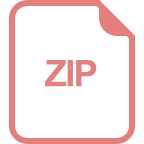
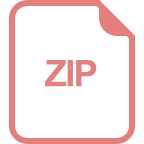
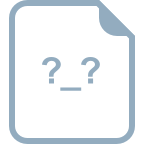
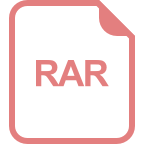
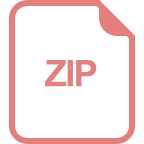