【Network Architecture】: Delving into DCGAN and Its Variants: Exploring the Diversity and Potential of GAN Architectures
发布时间: 2024-09-15 16:58:43 阅读量: 26 订阅数: 35 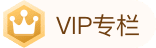
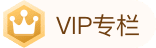
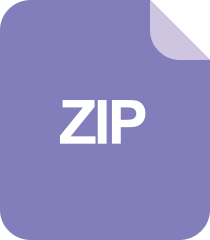
Dungeons-and-Delving:我们的德尔弗国防部
# 1. Deep Convolutional Generative Adversarial Networks (DCGAN): Exploring the Diversity and Potential of GAN Architectures
Generative Adversarial Networks (GAN) is a groundbreaking development in the field of artificial intelligence, particularly noted for its ability to generate images, videos, and other data that closely resemble reality. As an important variant of GAN, the Deep Convolutional Generative Adversarial Network (DCGAN) has garnered widespread attention for its exceptional performance in image generation. By incorporating deep convolutional networks, DCGAN significantly enhances the quality and diversity of images while ensuring the structural stability of the generator and discriminator. This chapter will provide an overview of the fundamental concepts, origins, and significance of DCGAN in the field of artificial intelligence, laying the foundation for a deeper understanding of the theoretical underpinnings and practical applications of DCGAN.
# 2. Theoretical Foundations and Architecture Analysis of DCGAN
## 2.1 Introduction to Generative Adversarial Networks (GAN)
### 2.1.1 How GAN Works
Generative Adversarial Networks (GAN) is a significant breakthrough in the field of deep learning, proposed by Ian Goodfellow in 2014. GAN consists of two components: the Generator and the Discriminator. The goal of the Generator is to create fake data that is as similar to real data as possible, while the Discriminator's task is to distinguish between real data and fake data generated by the Generator.
During training, the Generator and Discriminator compete with each other, akin to a zero-sum game in a contest. The Generator continuously learns to produce more realistic data to deceive the Discriminator, while the Discriminator continually improves its ability to better identify fake data. This adversarial training allows GAN to learn the underlying distribution of data and generate new, realistic data instances.
### 2.1.2 Loss Function and Optimization Objective of GAN
The loss function of GAN consists of two parts: one for the Discriminator and one for the Generator. The Discriminator's loss function aims to maximize its ability to distinguish between real and fake data, usually using cross-entropy loss. The Generator's loss is to minimize the probability that the Discriminator will judge its generated data as fake.
Specifically, the loss function can be formalized as:
```math
\min_G \max_D V(D, G) = \mathbb{E}_{x \sim p_{data}(x)}[\log D(x)] + \mathbb{E}_{z \sim p_z(z)}[\log(1 - D(G(z)))]
```
Here, `x` is the real data, `z` is the noise sampled from the latent space, `D(x)` represents the probability that the Discriminator judges data `x` as real, and `G(z)` represents the data generated by the Generator. During training, the Discriminator and Generator alternate between gradient ascent and descent, continuously updating their weights.
## 2.2 Key Improvements in DCGAN
### 2.2.1 Motivation for Introducing Deep Convolutional Structures
The Deep Convolutional Generative Adversarial Network (DCGAN), proposed by Radford et al. in 2015, aims to improve the stability issues of traditional GANs by incorporating Deep Convolutional Neural Network (CNN) structures. In traditional GANs, deep fully connected networks often led to training instability, and the quality of the generated images was unsatisfactory. The main motivation behind DCGAN is to leverage the successful experience of CNNs in image recognition, enhancing GAN performance through structured design.
### 2.2.2 Main Components of DCGAN Architecture
The key improvements in DCGAN mainly include replacing fully connected layers with convolutional layers and introducing Batch Normalization technology. In DCGAN, the generator gradually generates high-resolution images from random noise through a series of convolutional and deconvolutional layers. The discriminator uses convolutional layers and pooling layers to analyze image features.
Furthermore, DCGAN introduced Batch Normalization technology, which can stabilize the learning process and allow the use of a higher learning rate. Batch Normalization normalizes each small batch of data, reducing internal covariate shift, making training more stable.
## 2.3 Comparison of DCGAN with Other GAN Architectures
### 2.3.1 Differences from Traditional GAN Architectures
Compared to traditional GANs, DCGAN has made several key structural changes that significantly improve the model's performance and stability. First, DCGAN replaces the fully connected layers in the generator and discriminator with convolutional layers and transposed convolutional layers to capture the two-dimensional structural information of images. Second, DCGAN uses Batch Normalization to stabilize the training process and introduces LeakyReLU and tanh activation functions to enhance the model's nonlinear representation.
### 2.3.2 Advantages and Limitations of DCGAN
The advantage of DCGAN lies in its ability to generate higher resolution and clearer images, and it is more stable during training. DCGAN has achieved significant results in multiple image generation tasks, including face image synthesis and artistic style transfer.
However, DCGAN also has limitations. It may still face the problem of mode collapse, where the generator may repeatedly generate similar images, unable to cover the diversity of the data distribution. Additionally, training GANs typically requires finely designed training techniques and substantial computational resources, posing a considerable challenge for researchers and engineers.
DCGAN's success has provided an important reference for subsequent improvements in GAN architectures, and its applications in the field of image generation have greatly advanced research progress in GANs in other domains.
# 3. Practical Applications of DCGAN
The Deep Convolutional Generative Adversarial Network (DCGAN) has been widely applied in various fields, especially in tasks related to image and video generation, enhancement, and transformation. By replacing the fully connected layers of traditional Generative Adversarial Networks (GAN) with deep convolutional layers, DCGAN has greatly improved the quality and diversity of generated images while preserving the core concept of adversarial networks.
## 3.1 Image Generation and Synthesis
Image generation and synthesis is one of the typical application scenarios of GAN technology, and DCGAN has shown outstanding performance in this field, especially in generating highly realistic human face images and artistic creations.
### 3.1.1 Using DCGAN to Generate Human Face Images
DCGAN can generate new, realistic human face images by learning the distribution of a vast number of human face images. This process includes several steps:
1. Data Preparation: First, collect a large-scale human face dataset, ***
***work Construction: Construct the DCGAN generator and discriminator networks. The generator typically includes multiple convolutional layers and transposed convolutional layers to generate images from random noise; the discriminator includes convolutional layers and fully connected layers to distinguish between real and generated images.
3. Training Process: Use optimization algorithms, such as the Adam optimizer, to alternately train the generator and discriminator. In each training step, the generator tries to generate more realistic images to deceive the discriminator, while the discriminator tries to accurately identify real images.
4. Image Generation: After sufficient training, the generator can produce clear and diverse images.
```python
# Example code: Building the DCGAN generator model
from keras.models import Sequential
from keras.layers import Dense, Conv2D, Conv2DTranspose, Flatten, Reshape
def build_generator(z_dim):
model = Sequential()
model.add(Dense(1024*8*8, input_di
```
0
0
相关推荐







