【Code Practice】: Implementing GAN with TensorFlow_Keras: Beginners Can Also Get Started Easily
发布时间: 2024-09-15 16:49:31 阅读量: 23 订阅数: 26 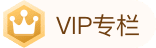
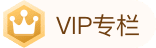
# 1. Introduction to Generative Adversarial Networks (GAN)
## 1.1 Overview of GAN
Generative Adversarial Networks (GAN) are a type of deep learning model comprised of two networks: a generator and a discriminator. They are trained through an adversarial process where the generator attempts to produce realistic data, and the discriminator tries to distinguish between real and generated data.
## 1.2 Applications of GAN
GANs can be applied to scenarios such as image generation, image restoration, and style transfer. For instance, they can generate non-existent human faces or convert sketches into realistic landscape paintings.
## 1.3 How GAN Works
The generator produces data from random noise and gradually learns to create realistic data. The discriminator evaluates the authenticity of data and provides feedback to the generator. This adversarial process drives the model to continuously improve until the generator can create indistinguishable fake data from real data.
```python
# A simple pseudo-code demonstrating the basic structure of GAN
# Assuming we use Python and Keras to build the model
# Generator model
def build_generator():
model = ... # Construct the generator model
return model
# Discriminator model
def build_discriminator():
model = ... # Construct the discriminator model
return model
# GAN model
def build_gan(generator, discriminator):
model = ... # Integrate the generator and discriminator into a GAN model
return model
# Instantiate the models
generator = build_generator()
discriminator = build_discriminator()
gan = build_gan(generator, discriminator)
```
The above sections introduce the basic concepts of GAN, including its introduction, applications, principles of operation, and a simple pseudo-code example, providing readers with a comprehensive and actionable knowledge framework.
# 2. Introduction to TensorFlow and Keras
## 2.1 Relationship and Advantages of TensorFlow and Keras
### 2.1.1 Basic Architecture of TensorFlow
TensorFlow is an open-source machine learning library developed by Google, utilizing dataflow graphs for numerical computation. Its underlying layers are written in C++, providing flexibility and performance advantages, while its upper layers are encapsulated by Python interfaces, making it more user-friendly for development and debugging. The dataflow graph is the core concept of TensorFlow, consisting of nodes and edges. Nodes typically represent mathematical operations, while edges represent the multidimensional array data, or tensors, being passed between these nodes. This architecture can decompose computation tasks into small subtasks, then execute them in parallel on multiple devices, greatly enhancing computational efficiency.
TensorFlow allows users to define and run complex algorithms with high-level languages like Python, internally converting algorithms into efficient execution plans through computational graphs. This design enables TensorFlow to effectively support deep learning models such as Convolutional Neural Networks (CNNs) and Recurrent Neural Networks (RNNs).
TensorFlow also provides TensorBoard for data visualization, which is particularly useful during model debugging and optimization phases. Its ecosystem is complete, with extensive community support and abundant learning resources. TensorFlow also supports distributed computing, making it suitable for processing large-scale datasets, especially for the needs of the deep learning field.
### 2.1.2 Features of Keras as a High-Level API
Keras is an open-source high-level neural network API that can run on different backends such as TensorFlow, CNTK, Theano, etc. Keras's design philosophy is user-friendly, modular, and extensible. Keras's API design is clean and intuitive, making the construction, training, and debugging of neural networks easier and more intuitive.
A key feature of Keras is its modularity. Models are composed of a series of reusable modules, including layers, loss functions, optimizers, etc. This design allows users to quickly combine and experiment with different neural network structures.
Another significant feature is its extensibility. While Keras provides many predefined components, users can also create new components by inheriting and extending existing classes. In addition, Keras allows users to define their own layers, loss functions, activation functions, etc., providing researchers and developers with a high degree of freedom.
Keras also supports rapid experimentation by automatically handling many low-level details of the model, such as data preprocessing and optimizer selection, allowing developers to iterate and improve models more quickly. It also includes various pre-trained models that can be directly applied to specific tasks or used as a starting point for one's own models.
## 2.2 Installation and Configuration of TensorFlow Environment
### 2.2.1 System Requirements and Installation Steps
Before installing TensorFlow, it's essential to ensure the system meets basic hardware and software requirements. TensorFlow supports both CPU and GPU, but for GPU operation, CUDA and cuDNN libraries are required. Additionally, at least 4GB of RAM is recommended, although 8GB or more memory is more ideal for large datasets and complex models.
The CPU version of TensorFlow can be installed using Python's package manager pip. Open a command line or terminal window, and enter the following command:
```bash
pip install tensorflow
```
If you need to install the GPU-supported TensorFlow version, first ensure that the CUDA and cuDNN libraries are correctly installed and configured. Then install TensorFlow-GPU:
```bash
pip install tensorflow-gpu
```
### 2.2.2 Verifying Installation and Configuring the Environment
After installation, it's necessary to verify that TensorFlow is correctly installed. This can be done by running a simple Python program. Open a Python file or interactive interpreter and attempt to import the TensorFlow module:
```python
import tensorflow as tf
hello = tf.constant('Hello, TensorFlow!')
sess = tf.Session()
print(sess.run(hello))
```
If the above code runs smoothly and outputs "Hello, TensorFlow!" on the screen, the installation is correct. If errors occur, the error information will usually indicate the problem, such as incorrect environment variable settings or version incompatibility.
## 2.3 Basic Operations in TensorFlow
### 2.3.1 Tensor Operations and Dataflow Graphs
In TensorFlow, a tensor is a multidimensional array used to carry data within a graph. Constants and variables are both tensors. Basic tensor operations include creating, indexing, slicing, reshaping, etc. Here are some basic tensor operations:
```python
import tensorflow as tf
# Create a constant tensor
constant_tensor = tf.constant([[1, 2], [3, 4]])
# Create a variable tensor
variable_tensor = tf.Variable(tf.random_normal([2, 2]))
# Tensor shape
shape = constant_tensor.get_shape()
# Tensor indexing and slicing
element = constant_tensor[1, 1]
slice_tensor = constant_tensor[0:2, 1:]
# Execute tensor operations within a session
sess = tf.Session()
print(sess.run(element)) # Output the result of indexing
print(sess.run(slice_tensor)) # Output the result of slicing
sess.close()
```
In TensorFlow, all computations are organized into a dataflow graph format. This graph consists of nodes and edges, where nodes perform operations, and edges represent multidimensional arrays passed between nodes. The graph is built during the definition phase, while the actual numerical computation is performed in a session (Session).
### 2.3.2 Automatic Differentiation and Gradient Descent
TensorFlow includes an automatic differentiation system that effectively computes gradients. This is particularly useful for training deep learning models, as these models often involve complex loss functions and many parameters. Automatic differentiation greatly simplifies the training process for models, as developers do not need to manually derive and write gradient computation code.
In TensorFlow, the basic steps of using the gradient descent algorithm for model parameter optimization are as follows:
```python
# Define the loss function
W = tf.Variable(tf.random_normal([1]), name="weight")
b = tf.Variable(tf.zeros([1]), name="bias")
x = tf.placeholder(tf.float32, shape=[None])
y_true = tf.placeholder(tf.float32, shape=[None])
# Define the predicted value
linear_model = W * x + b
# Define the loss function
loss = tf.reduce_mean(tf.square(linear_model - y_true))
# Define the gradient descent optimizer
optimizer = tf.train.GradientDescentOptimizer(learning_rate=0.01)
train = optimizer.minimize(loss)
# Execute the session
with tf.Session() as sess:
sess.run(tf.global_variables_initializer())
for i in range(1000):
sess.run(train, feed_dict={x: [1, 2, 3, 4], y_true: [2, 4, 6, 8]})
print(sess.run([W, b]))
```
In the above code, we first define a simple linear model and the loss function between the predicted value and the true value. We then use a gradient descent optimizer to minimize the loss function. By running the optimization steps within the session, the model parameters `W` and `b` are updated, gradually approaching the values that minimize the loss function.
# 3. Basic Structure of GAN Implementation in Keras
## 3.1 Theoretical Architecture of GAN
### 3.1.1 Role and Principle of the Generator
The generator plays a crucial role in GANs, with its main task to generate data close to the real distribution from a random noise vector. Theoretically, the generator learns the distribution of real data and gradually generates increasingly realistic data samples.
The principle of the generator can be likened to that of an artist who aims to create artwork from a pile of disordered raw materials (random noise). To achieve this, the generator learns to replicate the statistical characteristics of the real dataset. As training progresses, the generator gradually masters how to transform noise into meaningful data structures.
**Key Parameter Explanation**:
- **Dimension of the input noise vector**: This is where the generator starts, typically with a random noise vector as input.
- **Network structure**: The generator is composed of a series of neural network layers, commonly including fully connected layers, convolutional layers, transposed convolutional layers, etc.
- **Activation function**: Nonlinear activation functions such as ReLU or tanh are commonly used to enable the generator to learn complex distributions.
### 3.1.2 Role and Principle of the Discriminator
The discriminator plays another key role in the GAN model, tasked with distinguishing between real data and fake data generated by the generator. Through continuous learning and adjustment, the discriminator's ability to differentiate between the two improves.
In the theoretical model, the discriminator's working principle is similar to that of an art appraiser. Its goal is to identify which is the authentic piece and which is the counterfeit produced by the generator. To train the discriminator, it makes selections between a pair of real data and generated data through this adversarial process, and the discriminator's discernment capability gradually improves.
**Key Paramet
0
0
相关推荐
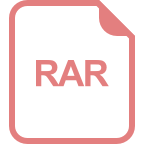
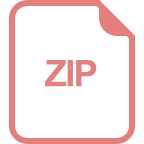
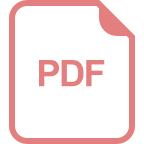





