【Advanced Tips】: Avoiding Mode Collapse: Advanced Solutions in GAN Training
发布时间: 2024-09-15 16:33:55 阅读量: 6 订阅数: 15 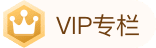
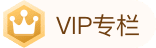
# Advanced Techniques: Avoiding Mode Collapse in GAN Training
## 1. Overview of Generative Adversarial Networks (GANs) and Challenges
### 1.1 Generative Adversarial Networks (GANs) Overview
Generative Adversarial Networks (GANs), proposed by Ian Goodfellow in 2014, are a class of deep learning models consisting of two primary neural network components: the Generator and the Discriminator. The Generator aims to produce samples as close to real data as possible, while the Discriminator's task is to differentiate between generated samples and actual ones. As training progresses, the Generator and Discriminator compete with each other, continuously improving the authenticity of the generated samples and the ability to discern, eventually reaching a dynamic equilibrium state.
### 1.2 GAN Application Scenarios
GANs have a wide range of applications in the field of computer vision, such as image synthesis, image restoration, style transfer, and data augmentation. Additionally, GAN technology has shown its potential in various other domains, including sound synthesis and text generation.
### 1.3 GAN Challenges
Despite the broad prospects and numerous applications of GANs, the training process is plagued by the problem of Mode Collapse. Mode Collapse occurs when the Generator starts producing repetitive samples, allowing the Discriminator to easily distinguish between generated and real samples, leading to ineffective model training. This is one of the key issues that current GAN research needs to address.
# 2. Theory and Impact of Mode Collapse
## 2.1 Definition and Causes of Mode Collapse
### 2.1.1 Theoretical Basis of Mode Collapse
Mode Collapse is a phenomenon in Generative Adversarial Networks (GANs) where the Generator begins to produce almost identical outputs instead of covering the entire data distribution. This typically occurs during training when the Generator finds a specific output that can easily deceive the Discriminator. It then continuously outputs this result.
To understand Mode Collapse, we must delve into the training mechanism of GANs. GAN consists of two main parts: the Generator and the Discriminator. The Generator's task is to create realistic data instances, while the Discriminator's task is to differentiate between generated data and real data. Their training is conducted through an adversarial process aiming to make the Generator produce data that is authentic enough to fool the Discriminator.
However, when the Generator learns to output a particular data point that can deceive the Discriminator with a high probability, it will repeatedly produce this output, resulting in Mode Collapse. This is because, in such cases, the Generator's gradient descent optimization algorithm cannot receive sufficient signals to explore other possible outputs, thus falling into a local optimum.
### 2.1.2 Conditions for Mode Collapse
The conditions that lead to Mode Collapse involve various aspects, including network architecture, training parameter settings, and the characteristics of the training data itself. A key factor is the competitive balance between the Discriminator and the Generator. If the Discriminator is too strong, it might quickly reduce its confidence in the generated data, causing the Generator to lose direction for progress and resort to simple yet incorrect strategies that lead to Mode Collapse.
Another significant factor influencing Mode Collapse is the diversity and complexity of the training data. If the data distribution is sparse in certain areas, the Generator might find a "shortcut" that does not require covering the entire distribution to achieve high scores. Additionally, unstable learning rates, excessively small batch sizes, and inappropriate loss functions are potential contributors to Mode Collapse.
## 2.2 Impact of Mode Collapse on GAN Training
### 2.2.1 Performance during Training
Mode Collapse is mainly表现为 a sharp decline in the diversity of generated data during training. Specifically, the Generator might begin to produce almost identical outputs or switch between a small number of different outputs. This phenomenon can be observed intuitively when generating samples from a trained GAN.
When Mode Collapse occurs, the training curve (e.g., the value of the loss function over time) usually exhibits an abnormal stable state rather than the expected fluctuations. This stability indicates that the Generator's updates are at a standstill because it has fallen into a state of producing similar samples. Consequently, the Discriminator's performance will also tend towards a fixed value since it faces almost unchanged generated samples.
### 2.2.2 Decline in Generated Sample Quality
The impact of Mode Collapse on the quality of generated samples is evident, directly causing a reduction in both the diversity and realism of the generated data. A healthy GAN system should be able to generate data that covers the entire distribution and is indistinguishable from real samples in quality. However, once Mode Collapse happens, the Generator's output becomes repetitive and unrepresentative.
This not only affects the practical value of the GAN system but also poses barriers to further training. Since the generated data is limited in diversity, the training of the Discriminator is also restricted, making it difficult for it to access enough varieties of data for effective learning. Moreover, due to the decline in the quality of the samples produced by the Generator, the model's generalization ability is reduced, resulting in poor performance in real-world applications.
## 2.3 Identification and Prevention of Mode Collapse
To proactively identify signs of Mode Collapse and take corresponding preventive measures, researchers and engineers must closely monitor various signals during the training process. A crucial step is to periodically check the Generator's output and use visualization tools or statistical analysis methods to assess the diversity of the samples.
Furthermore, adopting appropriate model and training strategies is essential. For example, using more complex or better-suited network architectures for specific datasets, introducing regularization techniques to prevent overfitting to specific samples by the Generator, and dynamically adjusting the learning rate and batch size are all effective methods.
Code example and explanation:
```python
# Assuming we have a basic GAN training function
def train_gan(generator, discriminator, dataset, epochs):
for epoch in range(epochs):
for real_data in dataset:
# Train Discriminator to recognize real data
discriminator.train_on(real_data)
# Generate some fake data
fake_data = generator.generate()
# Train Discriminator to recognize fake data
discriminator.train_on(fake_data)
# Train Generator to produce better fake data
generator.train_on(discriminator)
# Periodically check the diversity of generated samples
if should_check_diversity(epoch):
diversity_score = evaluate_diversity(generator)
if diversity_score < threshold:
# Signs of Mode Collapse detected, take action
apply_prevention_strategies(generator, discriminator)
def evaluate_diversity(generator):
# Evaluate the diversity of generated samples, implementation details omitted
pass
def apply_prevention_strategies(generator, discriminator):
# Implement preventive strategies, such as regularization techniques or architectural adjustments
pass
```
In this code, we define a function `train_gan` to train a GAN, which evaluates the diversity of samples at the end of each epoch and calls `apply_prevention_strategies` when signs of Mode Collapse are detected. Here, the implementation details of `evaluate_diversity` are omitted; this function would assess the diversity of the generated samples using statistical or visual analysis methods. In this way, we can take appropriate preventive measures before Mode Collapse occurs.
In the next section, we will delve into practical strategies to avoid Mode Collapse, including optimizing GAN loss functions, introducing regularization techniques, and employing advanced architectures and tricks.
# 3. Practical Strategies to Avoid Mode Collapse
## 3.1 Optimizing GAN Loss Functions
### 3.1.1 Basic Principles of Loss Functions
In Generative Adversarial Networks (GANs), the loss function is the core mechanism guiding network training, responsible for measuring the competitive relationship between the Generator and the Discriminator. The design of the loss function directly affects the training stability of GANs and the quality of the generated samples.
Typical GAN loss functions include minimizing the Discriminator's error rate in distinguishing between real and generated data and maximizing the probability of generated data being judged as real by the Discriminator. In practice, commonly used loss functions include Wasserstein loss, binary cross-entropy loss, and LSGAN (Least Squares GAN) loss, among others
0
0
相关推荐
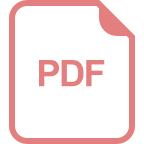
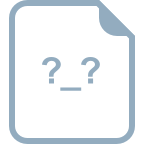
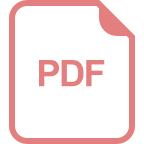
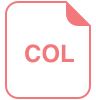
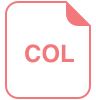
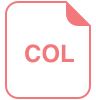
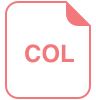

