【Algorithm Comparison】: A Major Contest of GAN Architecture Performance: Who is the Pioneer of Deep Learning?
发布时间: 2024-09-15 16:35:56 阅读量: 18 订阅数: 23 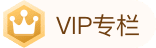
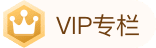
# 1. Introduction to Generative Adversarial Networks (GANs)
In the field of artificial intelligence, Generative Adversarial Networks (GANs) are a class of generative models that are trained using deep learning techniques. They learn data distributions through an adversarial process and create new instances of data. Since being proposed by Ian Goodfellow in 2014, GANs have garnered widespread attention from researchers and industry due to their powerful data generation capabilities.
The fundamental concept of GAN is to view the training process as a game between two neural networks: the Generator and the Discriminator. The Generator aims to produce data that is as realistic as possible, while the Discriminator tries to distinguish between generated data and real data. This adversarial mechanism is unique to GANs; it encourages both networks to progress interactively, ultimately allowing the Generator to produce outputs indistinguishable from real data.
This chapter will introduce the principles and architecture of GANs, as well as their potential applications across various fields, providing readers with a comprehensive understanding of GANs.
# 2. Basic Theory and Architecture of GANs
### 2.1 Theoretical Basis of GANs
#### 2.1.1 How GANs Work
Generative Adversarial Networks (GANs) consist of two primary components: the Generator and the Discriminator. During training, the goal of the Generator is to create fake data that closely resembles the true data distribution, while the Discriminator's goal is to differentiate between real and fake data generated by the Generator.
The Generator takes a random noise vector z as input and maps it to the data space through the network, outputting a sample G(z) that is尽可能 close to the real data. The Discriminator, on the other hand, receives a data sample x as input and outputs the probability D(x) that the sample comes from the real data distribution.
When training a GAN, the objective function often takes the form of a logarithmic function. The training goal for the Generator is to minimize the log-likelihood function log(1-D(G(z))), while the Discriminator aims to maximize the log-likelihood function log(D(x)) + log(1-D(G(z))).
The code block below demonstrates a simple GAN structure:
```python
import torch
import torch.nn as nn
# Generator network structure
class Generator(nn.Module):
def __init__(self):
super(Generator, self).__init__()
self.main = nn.Sequential(
# Network layer details omitted for brevity
)
def forward(self, z):
return self.main(z)
# Discriminator network structure
class Discriminator(nn.Module):
def __init__(self):
super(Discriminator, self).__init__()
self.main = nn.Sequential(
# Network layer details omitted for brevity
)
def forward(self, x):
return self.main(x)
# Initialize models and optimizers
generator = Generator()
discriminator = Discriminator()
g_optimizer = torch.optim.Adam(generator.parameters(), lr=0.0002)
d_optimizer = torch.optim.Adam(discriminator.parameters(), lr=0.0002)
```
In the above code, the `Generator` and `Discriminator` classes define the structures of the Generator and Discriminator, respectively. The `forward` method defines the forward propagation of the network layers. The `g_optimizer` and `d_optimizer` are used to optimize the parameters of the Generator and Discriminator.
#### 2.1.2 Key Components of GANs
The key components of GANs include the Generator and the Discriminator. The Generator learns to produce samples that increasingly approach the true data distribution, while the Discriminator gradually enhances its ability to discern real data from fake data.
The Generator consists of multiple fully connected layers, convolutional layers, or transposed convolutional layers, aiming to capture the true data distribution. To achieve this, the Generator typically includes a random noise input that is mapped through the neural network layer by layer to ultimately generate realistic data samples.
The Discriminator is a binary classifier that aims to give the probability that input data is real or fake data generated by the Generator. Like the Generator, the Discriminator is composed of multiple fully connected layers, convolutional layers, or pooling layers, and its recognition ability can be improved through training.
### 2.2 Common Variants of GAN Architectures
#### 2.2.1 Principles and Applications of DCGAN
The Deep Convolutional Generative Adversarial Network (DCGAN) is an important variant of GAN. It incorporates the structures of Convolutional Neural Networks (CNNs) into GANs, allowing the network to learn to generate high-resolution images more effectively. DCGAN uses deep convolutional layers in place of traditional fully connected layers and introduces Batch Normalization techniques to improve training stability.
DCGAN has a wide range of applications, including art creation, face image generation, and medical image analysis. Its exceptional image generation capabilities make it stand out in these fields.
The code block below shows an example of a convolutional layer in the Discriminator of DCGAN:
```python
class Discriminator(nn.Module):
# ... (other parts of the code)
def __init__(self):
super(Discriminator, self).__init__()
self.main = nn.Sequential(
nn.Conv2d(3, 64, 4, stride=2, padding=1), # Input channels 3, output channels 64
nn.LeakyReLU(negative_slope=0.2, inplace=True),
# ... (other convolutional layers)
)
def forward(self, x):
return self.main(x)
```
In the above code, `Conv2d` is the convolutional layer, and `LeakyReLU` is an activation function. Input images are processed through the convolutional layers and activation functions to extract features progressively, which are then passed to the classifier for real vs. fake data judgment.
#### 2.2.2 Innovations in CycleGAN
CycleGAN is a special architecture of GANs that innovates by not requiring paired training data to achieve image transformation between two different domains. CycleGAN imposes cycle consistency constraints (Cycle Consistency Loss) on two different Generators and Discriminators, allowing the model to learn the mapping between domains without relying on paired samples.
This architecture has shown its superiority in tasks such as style transfer, image-to-image translation, and seasonal image generation.
The code block below demonstrates the cycle consistency loss function in CycleGAN:
```python
def cycle_consistency_loss(real_A, reconstructed_A, lambda_weight):
loss = torch.mean(torch.abs(real_A - reconstructed_A))
return lambda_weight * loss
```
Here, `real_A` is an image from domain A, `reconstructed_A` is the result of an image being transformed through domain B and then back to domain A by the A-domain Generator. `lambda_weight` is the weight for the cycle consistency loss, used to balance this loss term's contribution to the overall loss.
#### 2.2.3 Analysis of StyleGAN Advantages
StyleGAN introduces operations in the latent space within the GAN architecture, allowing the model to control attributes of the generated images, such as pose, expression, and hair. StyleGAN introduces a latent style space (W-space) and a series of mapping networks, permitting more detailed and specific control over the generated images.
The advantage of StyleGAN lies in the higher quality, more detailed, and higher-resolution images it generates. Additionally, it allows users to create images with specific styles or attributes by modifying vectors in the W-space.
### 2.3 Performance Evaluation Standards for GANs
#### 2.3.1 FID and Inception Score
A common metric for evaluating the quality of images generated by GANs is the Fréchet Inception Distance (FID) score, which assesses the Generator's performance by comparing the distribution difference of real and generated images in feature space. A lower FID score indicates higher quality generated images.
Another commonly used evaluation metric is the Inception Score (IS), which uses a pre-trained Inception model to evaluate the diversity and quality of generated images. The Inception Score combines the assessment of both the quality and diversity of generated images, with a higher IS score indicating more realistic and diverse images.
The table below compares the FID and IS scores of different GAN models on standard datasets:
| Model | FID | Inception Score |
|--------------|-------|-----------------|
| StyleGAN | 12.8 | 19.6 |
| BigGAN | 15.6
0
0
相关推荐
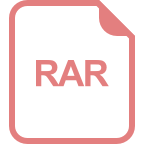
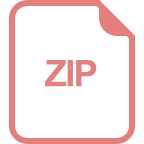
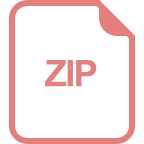
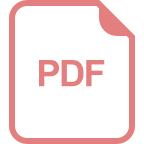
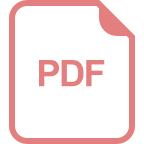
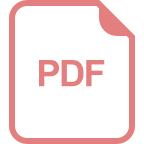
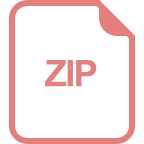
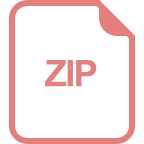
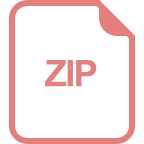