【Application Extension】: The Potential of GAN in Speech Synthesis: Welcoming a New Era of Voice AI
发布时间: 2024-09-15 16:43:06 阅读量: 25 订阅数: 32 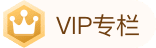
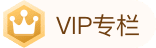
# 1. Overview of GAN Technology and Its Application in Speech Synthesis
Since its introduction in 2014, Generative Adversarial Networks (GANs) have become a hot topic in the field of deep learning. GANs consist of a generator and a discriminator, which oppose and learn from each other. This unique training approach has shown significant potential in dealing with high-dimensional data, especially achieving breakthrough results in areas such as image generation and artistic creation.
In recent years, the application of GAN technology in speech synthesis has gradually gained attention. Speech synthesis refers to the process of converting textual information into speech information, and GANs, through their unique generative adversarial mechanism, can effectively improve the naturalness and clarity of synthetic speech, addressing issues such as poor sound quality and insufficient emotional expression in traditional speech synthesis.
In this chapter, we will briefly introduce the basic concepts of GAN technology and explore its application in the field of speech synthesis. Additionally, we will learn how GANs improve the quality of speech synthesis through their unique learning mechanism and look forward to future development trends. By the end of this chapter, readers will have a comprehensive understanding of GAN technology and comprehend its practical application value in speech synthesis.
# 2. GAN Fundamental Theory and Architecture Analysis
### 2.1 Basic Principles of Generative Adversarial Networks (GANs)
#### 2.1.1 Core Components and Working Mechanism of GANs
Generative Adversarial Networks (GANs) consist of two parts: the generator and the discriminator. The generator's task is to create fake data that is as close to real data as possible. The discriminator, on the other hand, tries to distinguish between real data and the fake data generated by the generator. These two models compete with each other during training; the generator continuously learns to improve the quality of the data it generates, while the discriminator continuously improves its ability to distinguish between real and fake.
Technically, GAN training can be described in the following steps:
1. **Initialization**: Randomly initialize the parameters of the generator and discriminator.
2. **Generator Generating Data**: The generator receives a random noise vector and converts it into fake data.
3. **Discriminator Judging Data**: The discriminator receives a batch of data (including real and fake data generated by the generator) and outputs the probability that each piece of data is real.
4. **Loss Calculation and Parameter Update**:
- The generator's goal is to make the discriminator's output as close to 1 as possible (considering the generated data as real), so its loss function is typically the negative log-likelihood of the discriminator's output.
- The discriminator's goal is to correctly distinguish between real and fake data, so its loss function is the negative sum of the log-likelihood of real data and the log-likelihood of fake data.
5. **Iterative Training**: Repeat steps 2-4 until convergence.
#### 2.1.2 Challenges and Solutions During GAN Training
GAN training is very complex and prone to various issues, such as mode collapse, unstable training, and convergence to local optima. To address these challenges, researchers have proposed various strategies:
- **Wasserstein Loss**: Use the Wasserstein distance to measure the difference between two distributions, thus guiding the generator to produce more realistic data.
- **Label Smoothing**: Reduce the extreme values of real labels (usually 1) to lower the discriminator's overconfidence.
- **Gradient Penalty**: Introduce gradient penalty based on WGAN to ensure that the gradients of the generator and discriminator are neither too large nor too small during training.
- **Two-Phase Training**: Train the discriminator first to achieve a certain level of performance, then train the generator and discriminator simultaneously.
### 2.2 Different GAN Architectures and Variants
#### 2.2.1 Comparison of Common GAN Architectures
Different GAN architectures vary in terms of generation quality, training difficulty, and application scenarios. Here are some common GAN architectures and their characteristics:
- **DCGAN (Deep Convolutional GAN)**: Combines deep convolutional neural networks with GAN ideas, significantly improving the quality of generated images and making the process more stable.
- **StyleGAN**: Introduces the concept of style transfer by incorporating style codes into the generator, allowing it to control the style and texture of generated images.
- **CycleGAN**: Can achieve data transformation between two different domains, such as transforming horse images into zebra images. Its characteristic is that it does not require paired training data.
#### 2.2.2 Introduction and Application Scenarios of Special GAN Types
In addition to common GAN architectures, there are also some GAN variants designed for specific tasks:
- **Pix2Pix**: A conditional GAN often used for image-to-image translation tasks, such as converting sketch images into color images.
- **StackGAN**: Can generate high-resolution images by stacking multiple generators and discriminators, layer by layer, to enhance the details of the generated images.
- **BigGAN**: Generates high-quality images from large datasets by increasing the scale and number of parameters of the model.
### 2.3 Detailed Theoretical Analysis and Parameter Interpretation of GANs
#### 2.3.1 Parameter Interpretation and Theoretical Logic
In GANs, both the generator and discriminator are deep neural networks. Taking a simple fully connected network as an example, we can define the parameters as follows:
- `Wg`: Weight matrix of the generator
- `Wd`: Weight matrix of the discriminator
- `b_g`: Bias term of the generator
- `b_d`: Bias term of the discriminator
- `z`: Input noise vector of the generator
- `x`: Real data input
- `G(z)`: Function of the generator converting noise vector `z` into generated data
- `D(x)`: Function of the discriminator determining whether the input data `x` is real
During training, we aim to minimize the loss functions of the discriminator and generator:
- **Discriminator's loss function**:
$$ L_D = E_x[\log D(x)] + E_z[\log(1-D(G(z)))] $$
- **Generator's loss function**:
$$ L_G = E_z[\log(1-D(G(z)))] $$
Gradients for parameter updates are typically calculated using backpropagation. The parameters of the discriminator and generator are updated through gradient descent.
```python
# Pseudocode for the generator model
def generator(z):
# z is a random noise vector
return G(z) # Convert the noise vector into generated data
# Pseudocode for the discriminator model
def discriminator(x):
# x is the input data, which can be real or generated data
return D(x) # The output is the probability that the data is real
# Pseudocode for loss function calculation and parameter update
def train_step(x, z):
# Train the discriminator with real and generated data
D_real = discriminator(x)
G_fake = generator(z)
D_fake = discriminator(G_fake)
loss_d = ... # Calculate the discriminator's loss function
loss_g = ... # Calculate the generator's loss function
# Update the discriminator's parameters
d_optimizer.step(loss_d)
# Update the generator's parameters
g_optimizer.step(loss_g)
# Training loop
for epoch in range(num_epochs):
for x, z in data_loader:
train_step(x, z)
```
### 2.4 GAN Architecture Analysis and Model Structural Evolution
#### 2.4.1 Architecture Analysis
GAN architecture has evolved from simple fully connected networks to deep convolutional networks. This transition has greatly improved the quality and diversity of generated images. Convolutional GAN architectures, such as DCGAN, replace the fully connected layers in GANs with convolutional and transposed convolutional layers, allowing the generator to produce images with higher resolution and more complex structures.
Batch normalization is often used in the design of discriminators to accelerate training and improve the quality of generated images. To enhance the stability of the training process, discriminators are frequently designed as deep networks.
```mermaid
graph TD;
Z[z (random noise vector)]
G[Generator <br> G(z)]
D[Discriminator <br> D(x)]
X[Real Data x]
XG[Generated Data G(z)]
Z --> G -->|G(z)| D
X -->|x| D
D -->|D(x)| C1[Discriminator Loss]
D -->|D(G(z))| C2[Generator Loss]
```
#### 2.4.2 Model Structural Evolution
GAN architecture has continuously evolved with in-depth research. For example, StyleGAN introduced AdaIN (Adaptive Instance Normalization) and progressive training strategies, allowing for fine control over the local and global styles of generated images. BigGAN significantly improved the resolution and quality of generated images by increasing model capacity and the scale of parameters.
When choosing an architecture, it is necessary to consider the specific requirements of the target task, such as the resolution of generated images, stylistic consistency, and diversity. For specific tasks, it may be necessary to improve existing GAN architectures or develop new ones to meet the requirements.
In GAN research and applications, theoretical analysis, parameter interpretation, and model structural evolution are inseparable. A deep understanding of these contents can help researchers and practitioners design, train, and apply generative adversarial networks more effectively.
# 3. GAN Application Practice in Speech Synthesis
## 3.1 Theoretical Application of GAN in Speech Synthesis
### 3.1.1 Role of GAN in Improving Speech Quality
A significant application of Generative Adversarial Networks (GANs) in speech synthesis is to enhance the naturalness and quality of synthetic speech. Traditional speech synthesis systems, such as parametric synthesis or Concatenative TTS (Text-to-Speech), often face drawbacks like unnatural sound and lack of realism. GANs, through adversarial learning, can generate more realistic speech waveforms.
To make GANs work in speech synthesis, researchers usually design GANs as a sequence generation model, where the generator (Generator) is responsible for generating spe
0
0
相关推荐
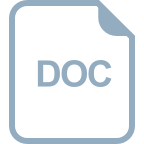
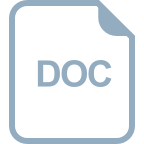
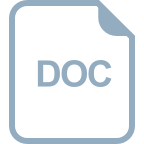
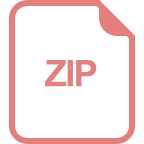
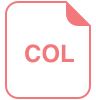
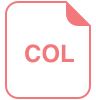
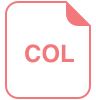
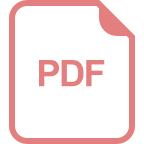
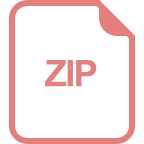