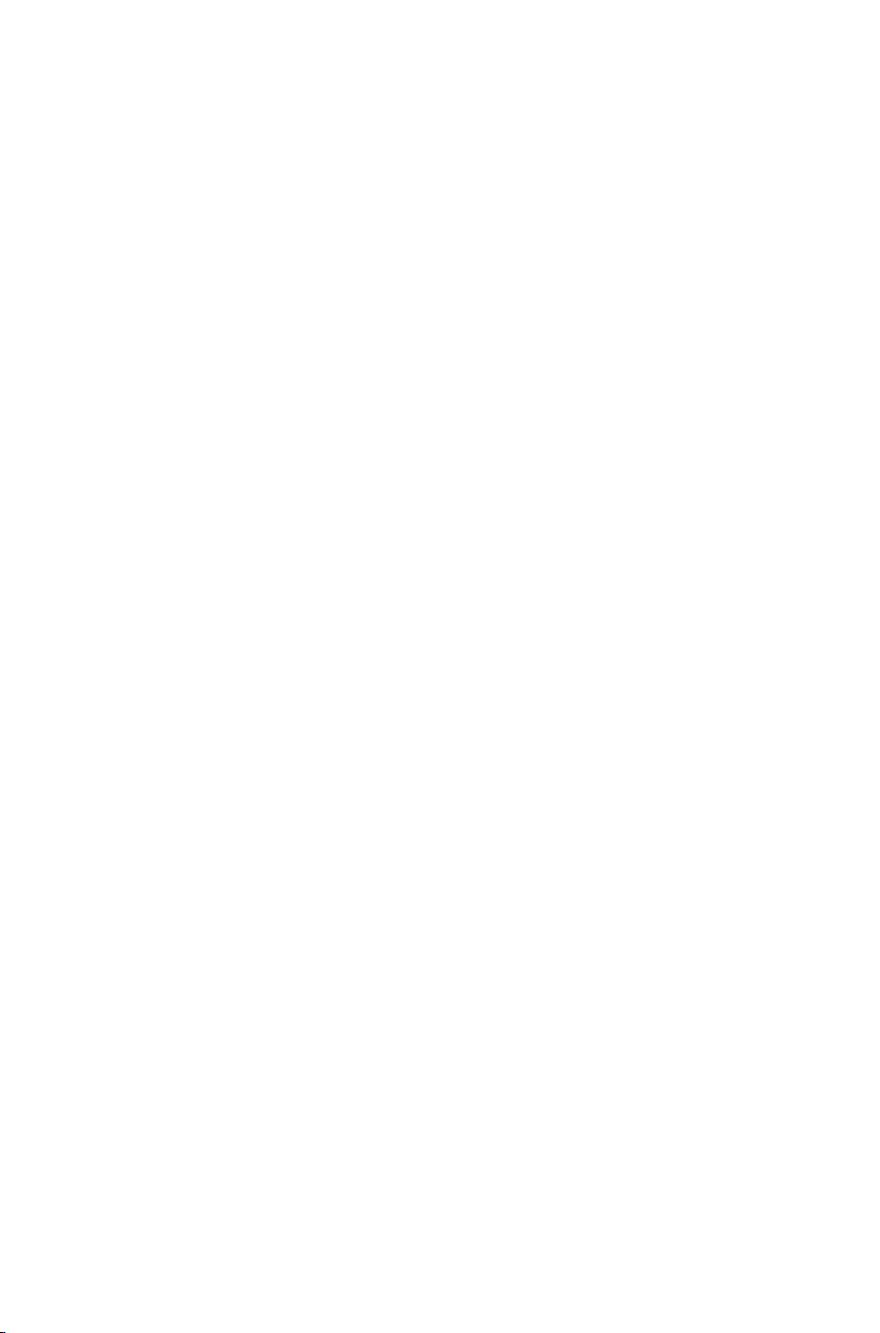
may report on the results obtained on the training and validation sets, but only the results
on the test set will be taken into account in the final competition.
Since emotion recognition has received increasing attention, we also want to take
this opportunity to promote the development of affective computing by encouraging the
participants to share their work. The participants should submit executable files used in
this challenge and documentation to OpenPR (http://www.openpr.org.cn/), and share
with other researchers. The Open Pattern Recognition (OpenPR) project is an open
source platform for sharing algorithms of image processing, computer vision, natural
language processing, pattern recognition, machine learning and related fields. OpenPR
was initiated in 2009 under the BSD license, and is currently supported by the National
Laboratory of Pattern Recognition, Institution of Automation, Chinese Academy of
Sciences. From 2009 to now, OpenPR has become a valuable resource in pattern recog‐
nition, and the codes contained have been downloaded more than 50,000 times.
The remainder of this paper is organized as follows. Section 2 describes the database
used in this challenge. Sections 3 and 4 present the features and the baseline experiments.
Finally, Sect. 5 concludes this paper.
2 Multimodal Emotional Database
One of the major needs of the affective computing society is the constant requirement
of emotional data. The existing emotional corpora could be divided into three types:
simulated/acted, elicited and natural(-istic) corpora [9, 10]. Recently, the demand for
real application forces emotion researchers to put more effort on natural and spontaneous
emotion data. Ideally, a corpus should be collected from our daily life which includes
natural and spontaneous emotion. But because of copyright and privacy issues, several
of the existing natural(-istic) emotion corpora were collected from films and TV
programs (Yu et al. 2001). Although movies and TV programs are often shots in
controlled environments, they are significantly closer to real-world environments than
the lab-recorded datasets due to highly varying and often adverse conditions. Some of
the successful examples are the Belfast Natural Database [11], the Vera am Mittag
German Audio-visual Emotional Speech Database (VAM) [12], the EmoTV Database
[13] and the SAFE (Situation Analysis in a Fictional and Emotional) Corpus [14]. But,
none of these corpora is in Chinese. Since emotion expression has some specific char‐
acteristics for different languages in different cultures, in the challenge, we choose the
CASIA Chinese Natural Emotional Audio-Visual Database (CHEAVD) [15], which
aims to provide a basic Chinese resource for the research on multimodal multimedia
interaction.
CHEAVD contains 140 min spontaneous emotional segments extracted from films,
TV plays and talk shows. 238 speakers, aging from child to elderly, are included in this
database. The partition of the recordings with respect to gender is as follows: 52.5 % are
male subjects, 47.5 % are female subjects. A discrete emotion annotation strategy is
adopted, and 26 non-prototypical emotional states, including the basic six, were labeled
by four native speakers. Pairwise kappa coefficients were calculated to evaluate the
annotation consistency, which are shown in Table 1. In contrast to most other available
MEC 2016: The Multimodal Emotion Recognition Challenge 669