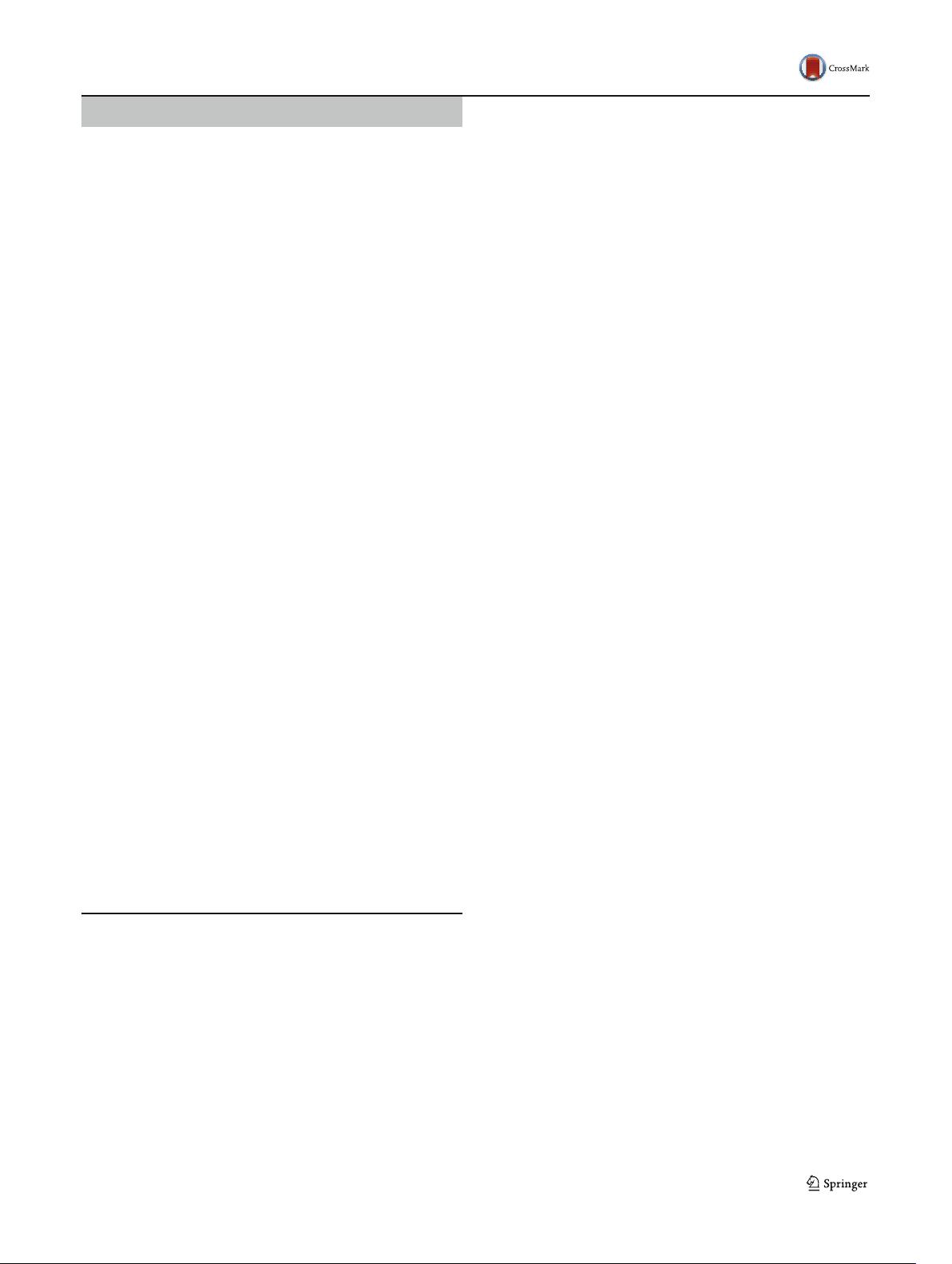
ORIGINAL PAPER
Chao Wang
1,2
& Wei Xu
1,2
& Xiao-fang Pei
1,3
& Xiao-yan Zhou
1,2
Received: 16 November 2015 /Accepted: 19 September 2016 /Published online: 30 September 2016
#
Saudi Society for Geosciences 2016
Abstract The continuous improvement of the launched satel-
lites’ spatial and spectral resolutions has brought new chal-
lenges for remote sensing image segmentation technology.
The traditional supervised methods greatly depend on artificial
interpretation and reduce the degree of automation and robust-
ness of image segmentation. Therefore, the article proposes a
novel unsupervised multi-scale segmentation method for high-
resoluti on remote sensin g images based on automated parame-
terization and it mainly includes three steps, adaptive selection
of scale parameter (SP) based on local area homogeneity index
J-value, multi-scale segmentation based on the inter-scales
boundaries constraint strategy, and region merging based on
multi-features. The article makes experiments by multi-group
high-resolution remote sensing images of different launched
satellites and compares the proposed method with the well-
known commercial software eCognition and a traditional su-
pervised method. The results show that the proposed method
can locate the object edges more accurately and extract the
object outlines more completely, and needs no human interven-
tion in segmentation process, so it can provide a generic and
effective unsupervised solution for high-resolution remote
sensing image segmentation.
Keywords High-resolution
.
Remote sensing image
.
Multi-scale segmentation
.
Unsupervised
.
Automated
parameterization
Introduction
In recent years, object-based image analysis (OBIA) has been
more and more emphasized in the fields of geographic infor-
mation science (GIScience) and remote sensing, especially the
application field of high-resolution remote sensing images.
Image segmentation is one of the most important treatment
aspects of OBIA. By segmenting images into spatially contin-
uous objects with relatively consistent internal attributes, ob-
ject classification and recognition can be performed based on
the statistical features (such as texture, structure) of these ob-
jects (Blaschke 2010). With increasing spatial resolution of
launched sensors, meter and sub-meter high-resolution data
coming from SPOT 5 and QuickBird have been widely ap-
plied for each field of social life, including urban land plan-
ning and monitoring, etc. Therefore, the image segmentation
technology aiming at high-resolution remote sensing images
has become one of the research hotspots in the field of remote
sensing (Gong et al. 2008; Liu et al. 2014; Gaetano et al.
2015).
Compared with moderate or low-resolution remote sensing
images, high-resolution remote sensing images can provide
more abundant texture features, clearly express detailed spa-
tial information such as the structure and shape of geograph-
ical objects. Besides, they effectively solve the pro minent
problem of mixed pixels to enhance inter-class separability
of adjacent surface features (Malik and Bhat 2014;Lietal.
2014). However, increasing spatial resolution also brings new
difficulties and challenges for the image segmentation. For
example, the phenomenon of Bsame objects with different
* Chao Wang
hhu_wch@163.com; chaowang@nuist.edu.cn
1
Key Laboratory of Meteorological Disasters of China Ministry of
Education, Nanjing University of Information Science and
Technology, Nanjing, China
2
Jiangsu Key Laboratory of Meteorological Observation and
Information Processing, Nanjing University of Information Science
and Technology, Nanjing, China
3
Collaborative Innovation Center of Atmospheric Environment and
Equipment Technology, Nanjing, China
Arab J Geosci (2016) 9: 651
DOI 10.1007/s12517-016-2683-4
An unsupervised multi-scale segmentation method based
on automated parameterization